Measurement and Causal Inference-Causal Insights Discovery
Unlock the power of AI for causal insights
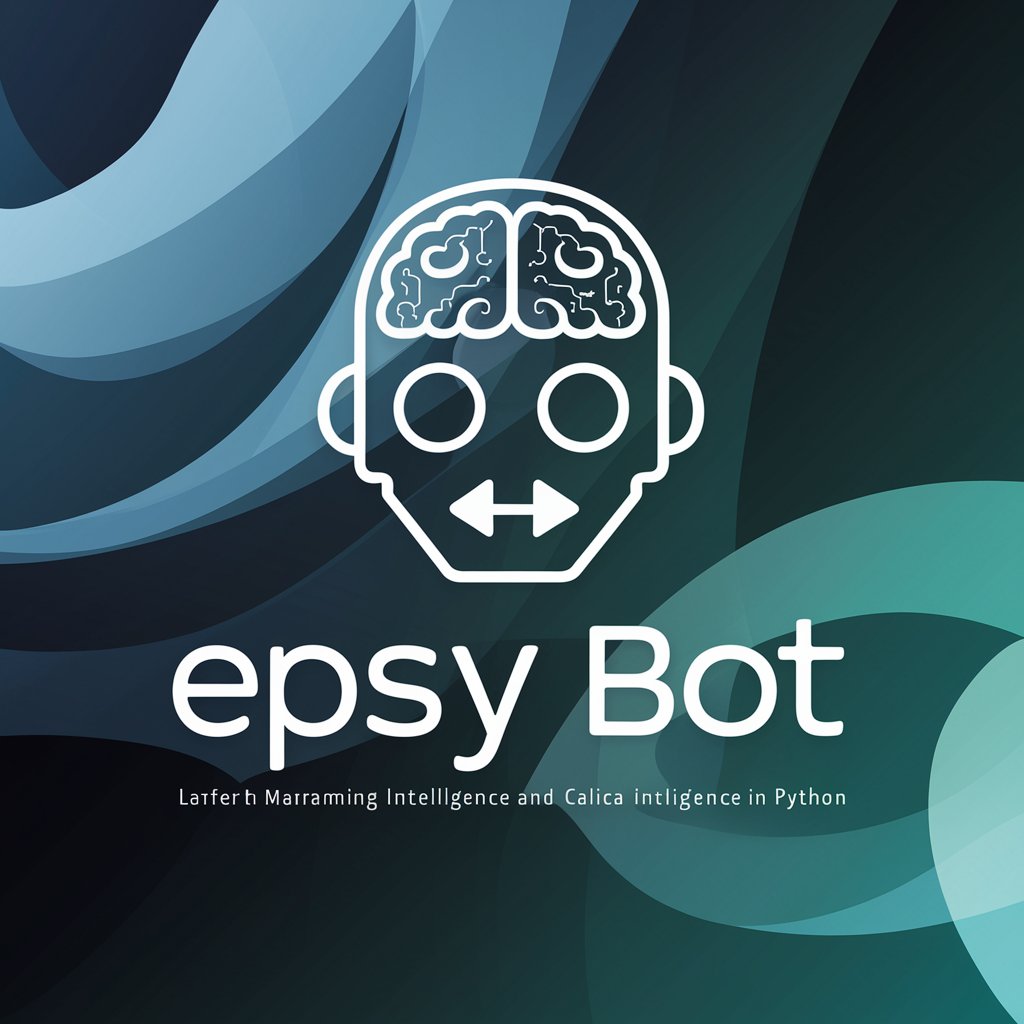
Explain the concept of causal inference using Python code.
Describe a method for measuring intangible business assets.
Provide an example of using propensity scores in Python.
Summarize the effectiveness of linear regression in causal analysis.
Related Tools
Load More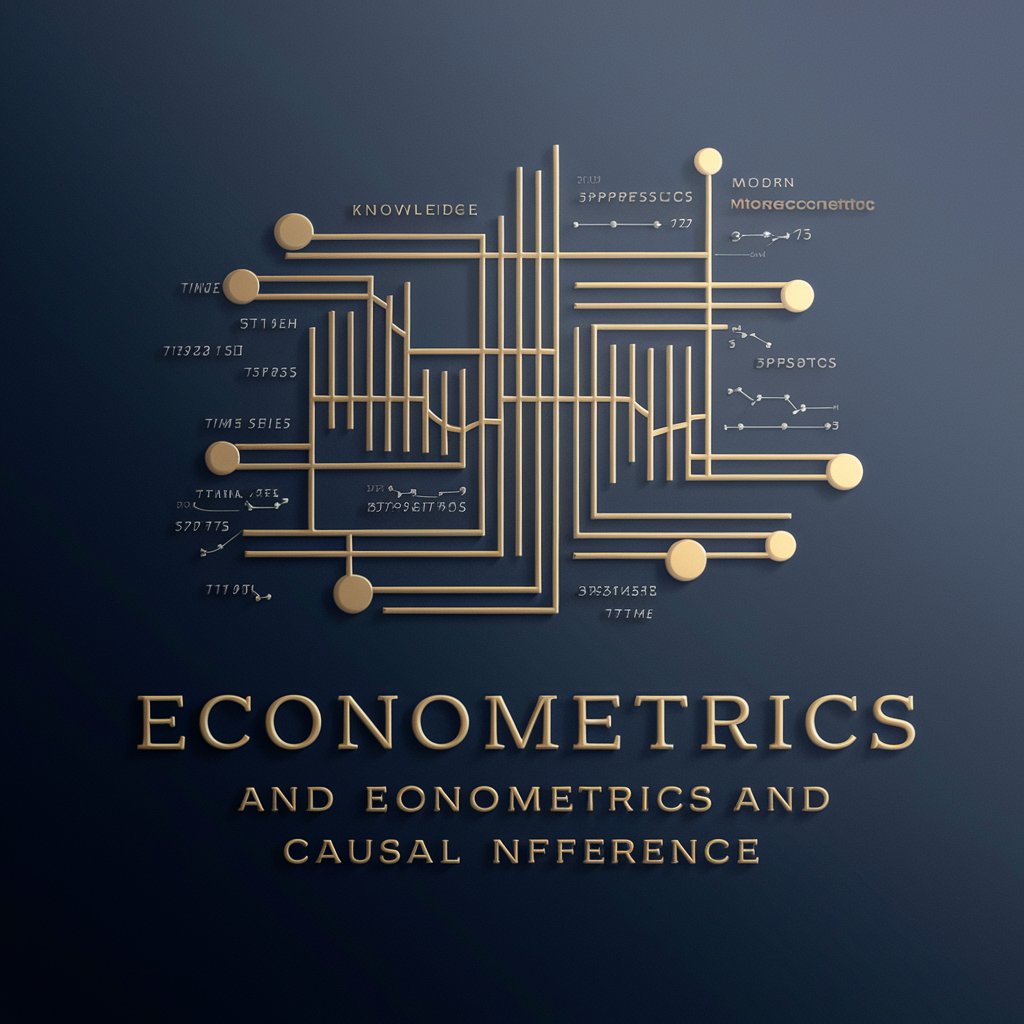
Econometrics and Causal Inference Expert
Expert in Econometrics, Causal Inference, and Economics
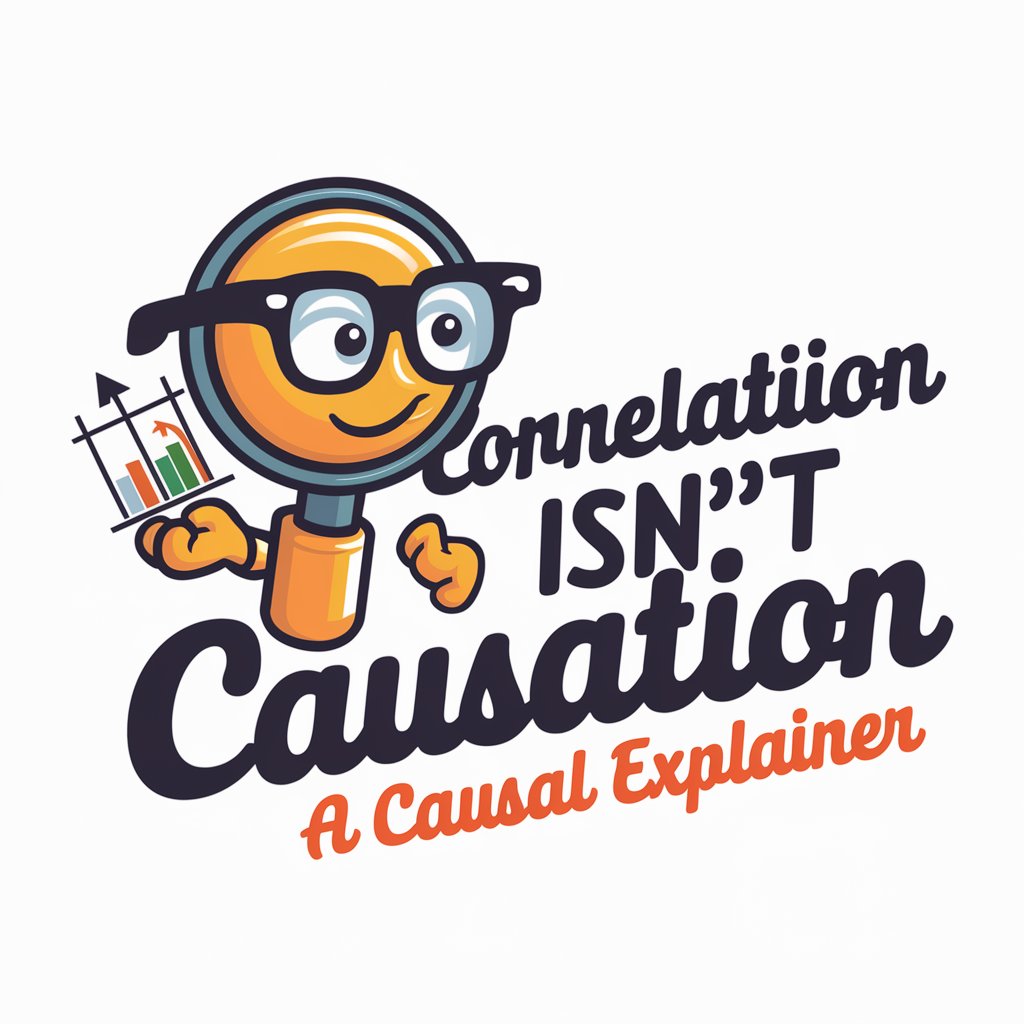
"Correlation isn't Causation" - A causal explainer
Answering everyone's favorite objection to academic papers
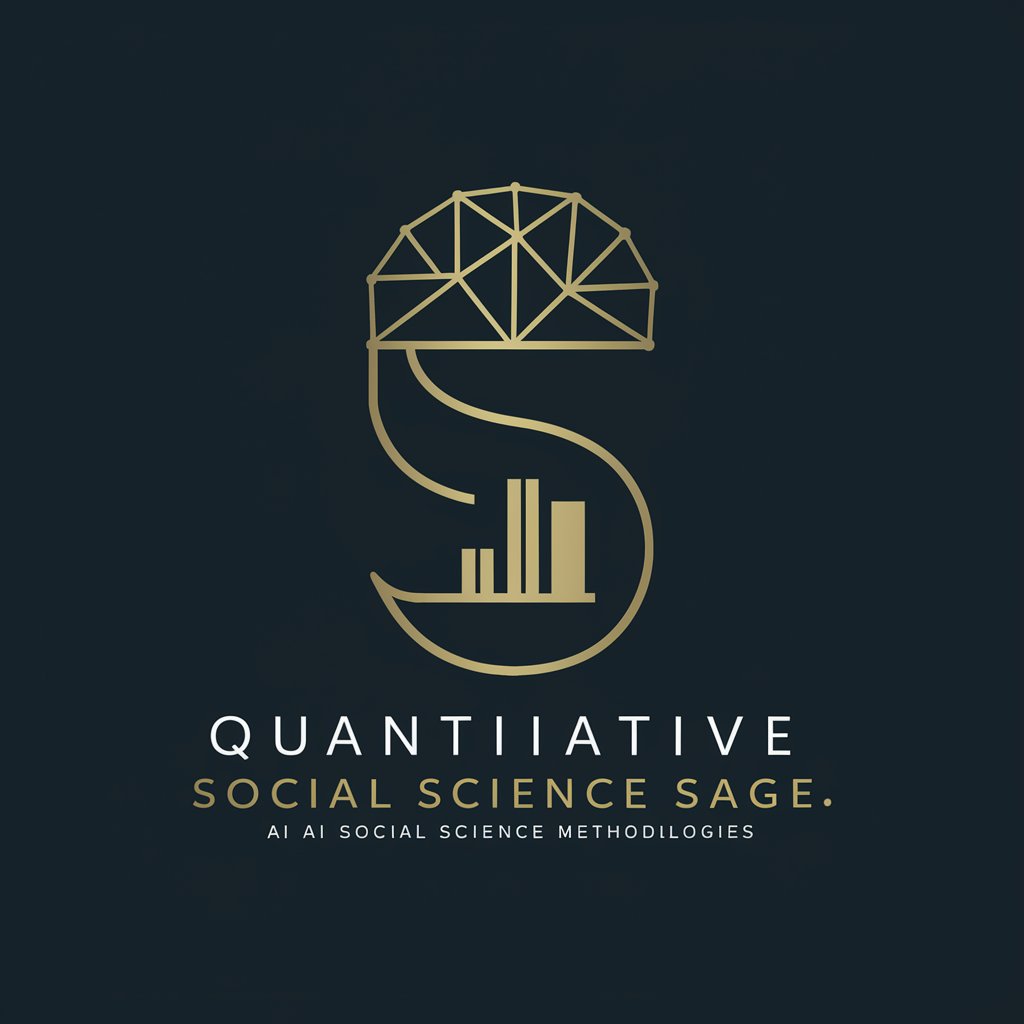
Quantitative Social Science Sage
Professor-like guide to social science methods, with R code detail.
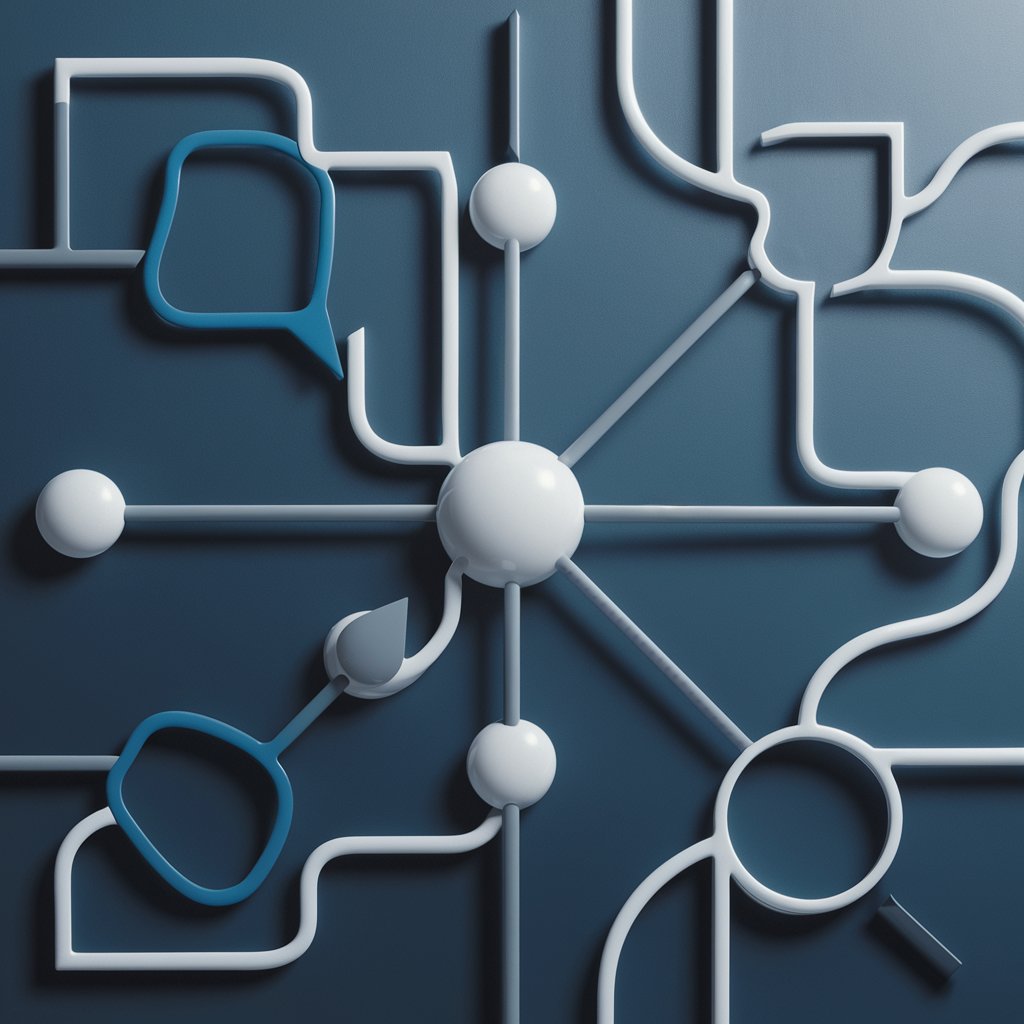
Causal Rater for Story
This GPT reads a story that has its events labeled by number, and returns the event pairs that had a strong causal relationship.
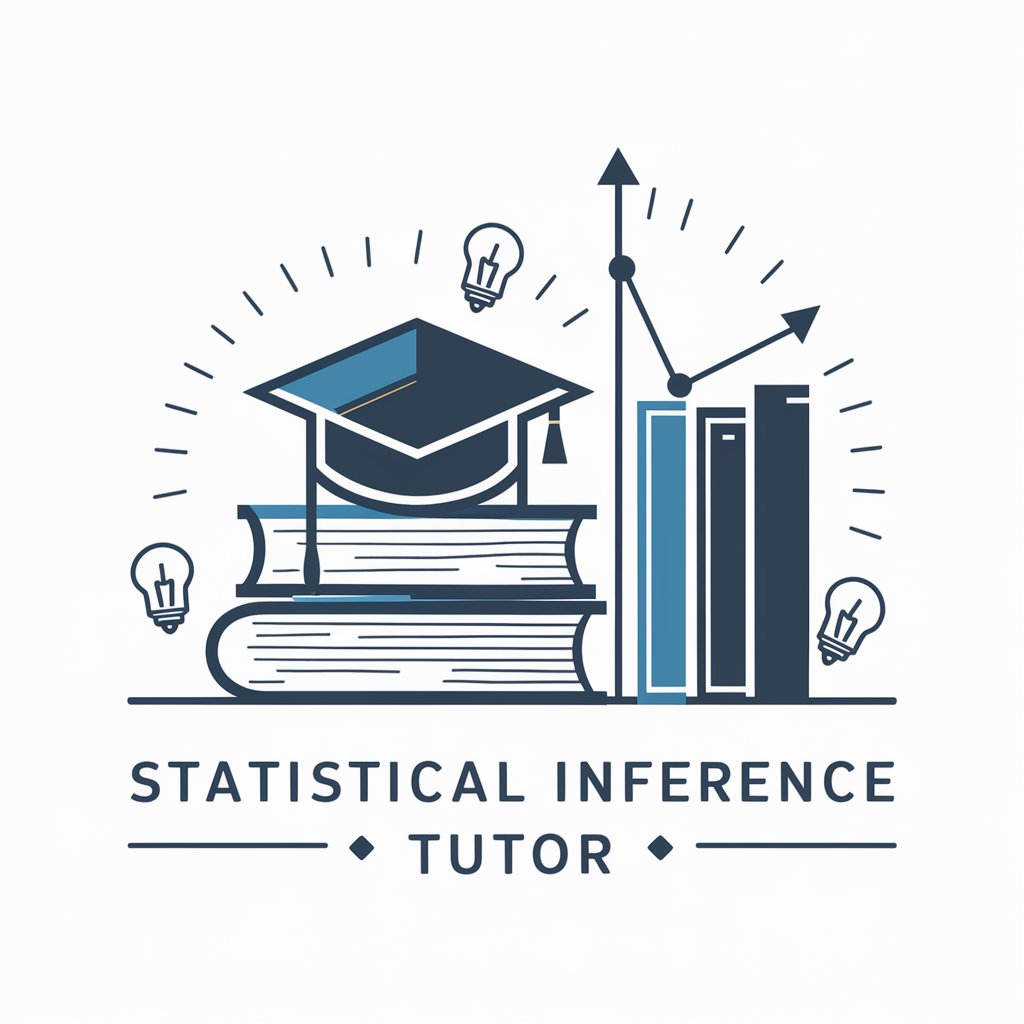
Statistical Inference Tutor
A tutor for graduate-level statistical inference, providing detailed explanations.
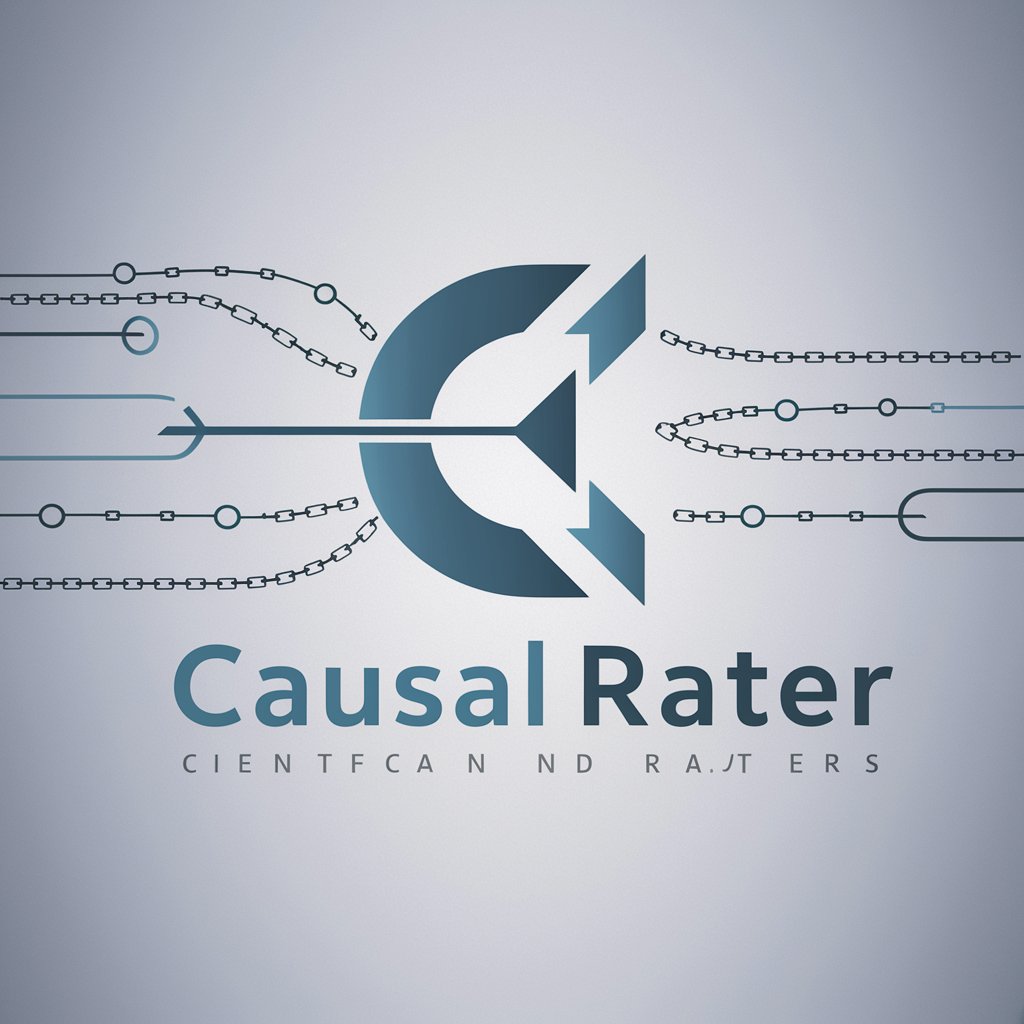
Causal Rater
Pinpoints primary causes, avoiding less direct factors.
20.0 / 5 (200 votes)
Introduction to Measurement and Causal Inference
Measurement and Causal Inference (MCI) is designed to address the challenges of quantifying and understanding the effects of various factors in business and research settings. This field combines rigorous statistical techniques and causal inference theories to uncover relationships that are not immediately observable. MCI is particularly valuable for evaluating the impact of intangible factors, such as management effectiveness, customer satisfaction, or policy changes, on measurable outcomes. For example, a company might use MCI methods to determine the causal effect of a new marketing strategy on sales, by carefully controlling for other variables that could influence the result. Similarly, researchers might apply MCI to assess the impact of educational interventions on student performance, taking into account factors like socioeconomic status. Powered by ChatGPT-4o。
Main Functions of Measurement and Causal Inference
Quantifying Intangibles
Example
Determining the monetary value of brand reputation or employee morale.
Scenario
A company wants to assess the return on investment in its employee wellness programs. Using MCI methods, it can measure how improvements in employee morale lead to increases in productivity and, ultimately, to higher profitability.
Causal Effect Estimation
Example
Assessing the impact of a training program on employee performance.
Scenario
An organization implements a new training program for its sales team. MCI techniques like randomized controlled trials or statistical models are used to isolate the effect of the training from other variables, such as market conditions or individual talent, thereby accurately estimating its impact on sales figures.
Policy Analysis
Example
Evaluating the effects of regulatory changes on industry practices.
Scenario
A government body introduces new environmental regulations for manufacturing industries. MCI can help in understanding the causal impact of these regulations on pollution levels and compliance costs, informing both future policy decisions and industry responses.
Optimizing Decision Making
Example
Using predictive models to tailor marketing strategies to individual customer preferences.
Scenario
A retail company uses MCI to analyze customer data and identify which factors most strongly influence purchase decisions. This information allows the company to customize its marketing efforts, targeting customers with the most relevant offers and thereby maximizing conversion rates.
Ideal Users of Measurement and Causal Inference Services
Business Analysts and Managers
These professionals need to make informed decisions based on data. MCI provides them with tools to quantify the effects of various strategies or changes within the organization, enabling evidence-based decision-making.
Researchers and Academics
Individuals in academia or research institutions conducting studies in economics, sociology, psychology, and other fields benefit from MCI's rigorous methods for establishing causality and measuring complex phenomena.
Policy Makers and Government Officials
These users rely on MCI to evaluate the impact of policy interventions, regulatory changes, and public programs. MCI helps ensure that policies are based on solid evidence of their effectiveness and potential for social benefit.
Healthcare Professionals and Organizations
In healthcare, MCI methods are crucial for assessing the effectiveness of treatments, interventions, and public health strategies, thus supporting better outcomes for patients and communities.
Guide to Using Measurement and Causal Inference
1
To begin your journey with Measurement and Causal Inference, start by exploring yeschat.ai for a complimentary trial that doesn't require any form of sign-up or ChatGPT Plus subscription.
2
Familiarize yourself with the core concepts of measurement and causal inference by reviewing foundational materials or courses that cover the basics of statistics, probability, and experimental design.
3
Apply the learned concepts by engaging in practical projects or analyses. Utilize Python, specifically libraries like pandas for data manipulation, statsmodels for statistical modeling, and causalml or econml for causal inference.
4
To enhance understanding and application, participate in forums or communities related to data science and causal inference. Share your projects, seek feedback, and exchange ideas with peers.
5
Continuously update your knowledge and skills by following the latest research, attending webinars or conferences, and experimenting with new tools and techniques in the field of measurement and causal inference.
Try other advanced and practical GPTs
PPC Privacy Measurement Support (by Thomas Eccel)
Navigate ad privacy with AI-driven support
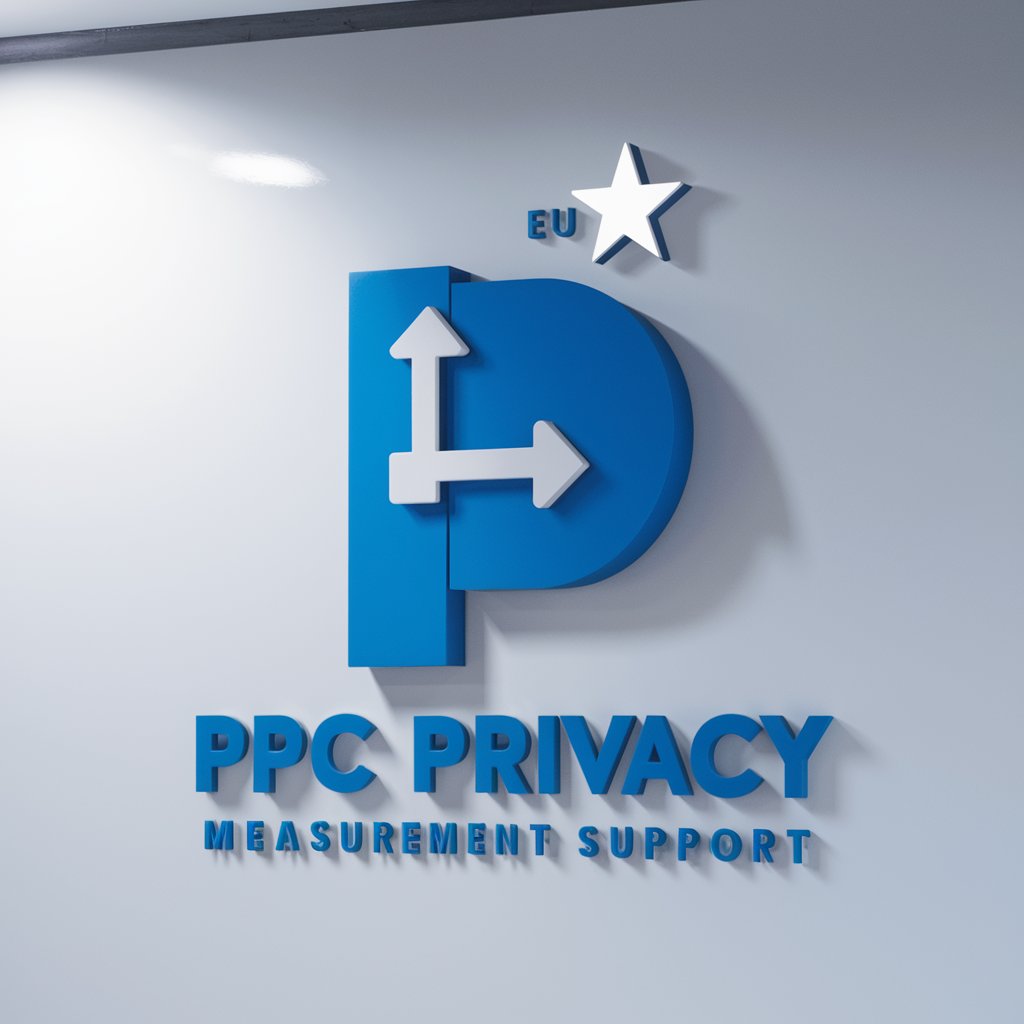
Spine Cobb Angle Measurement Tool
Precision in Spinal Curve Assessment
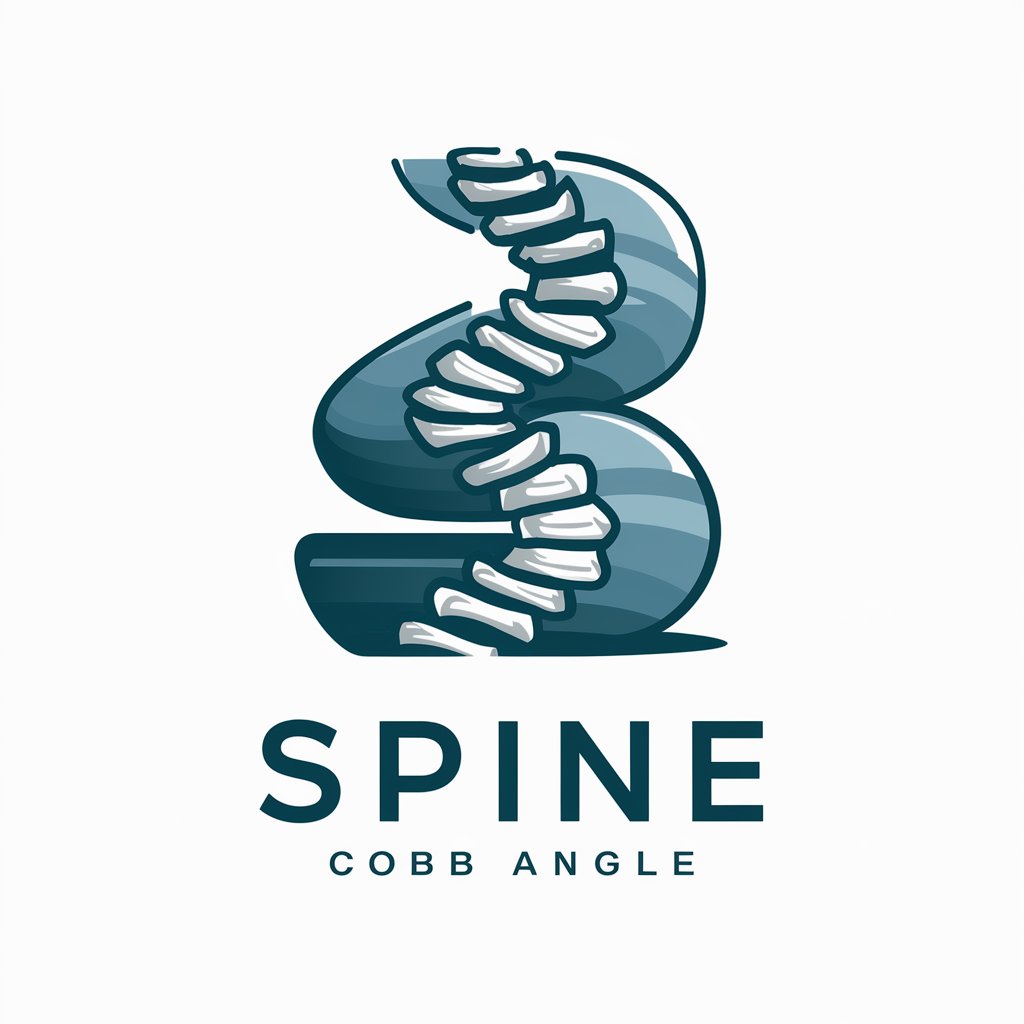
Bubble.io Assistant
Streamline Bubble.io development with AI.
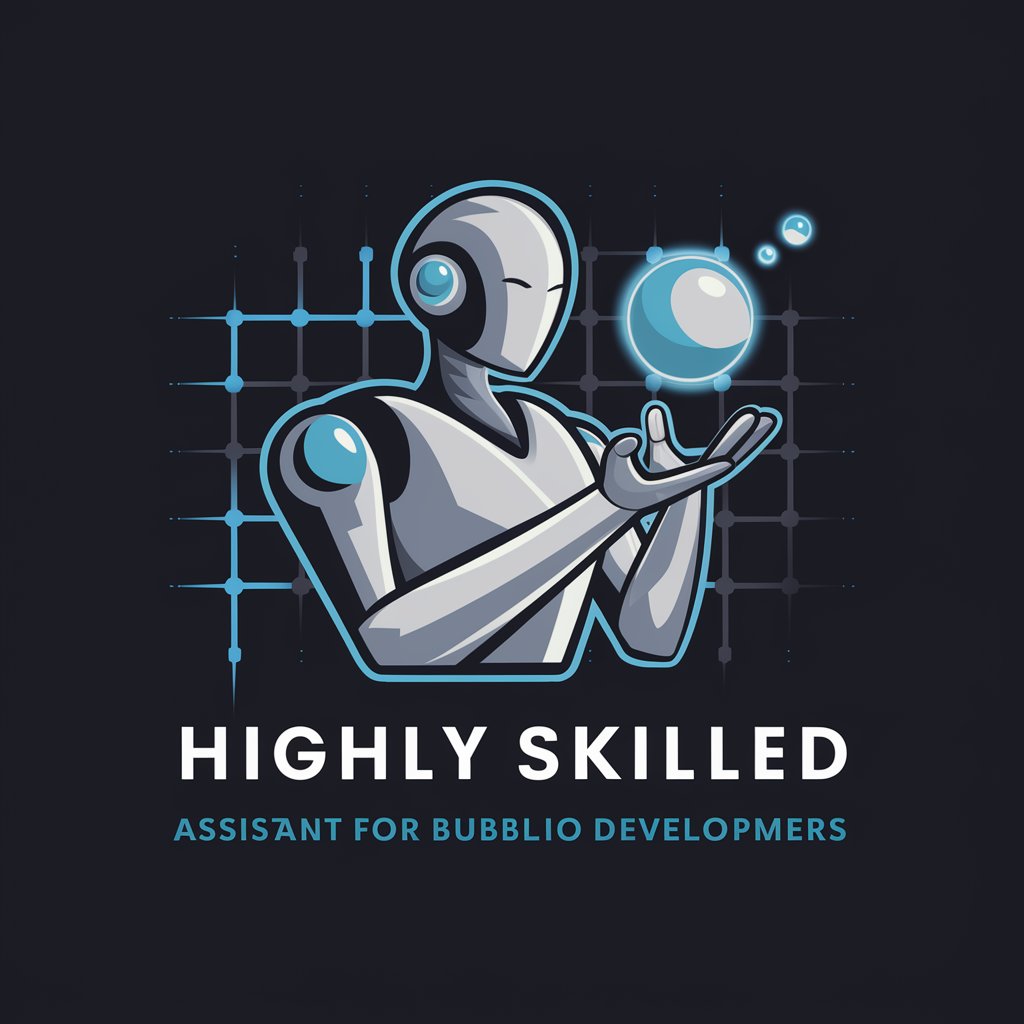
Azure Permissions Guide
Empowering secure access with AI-powered insights
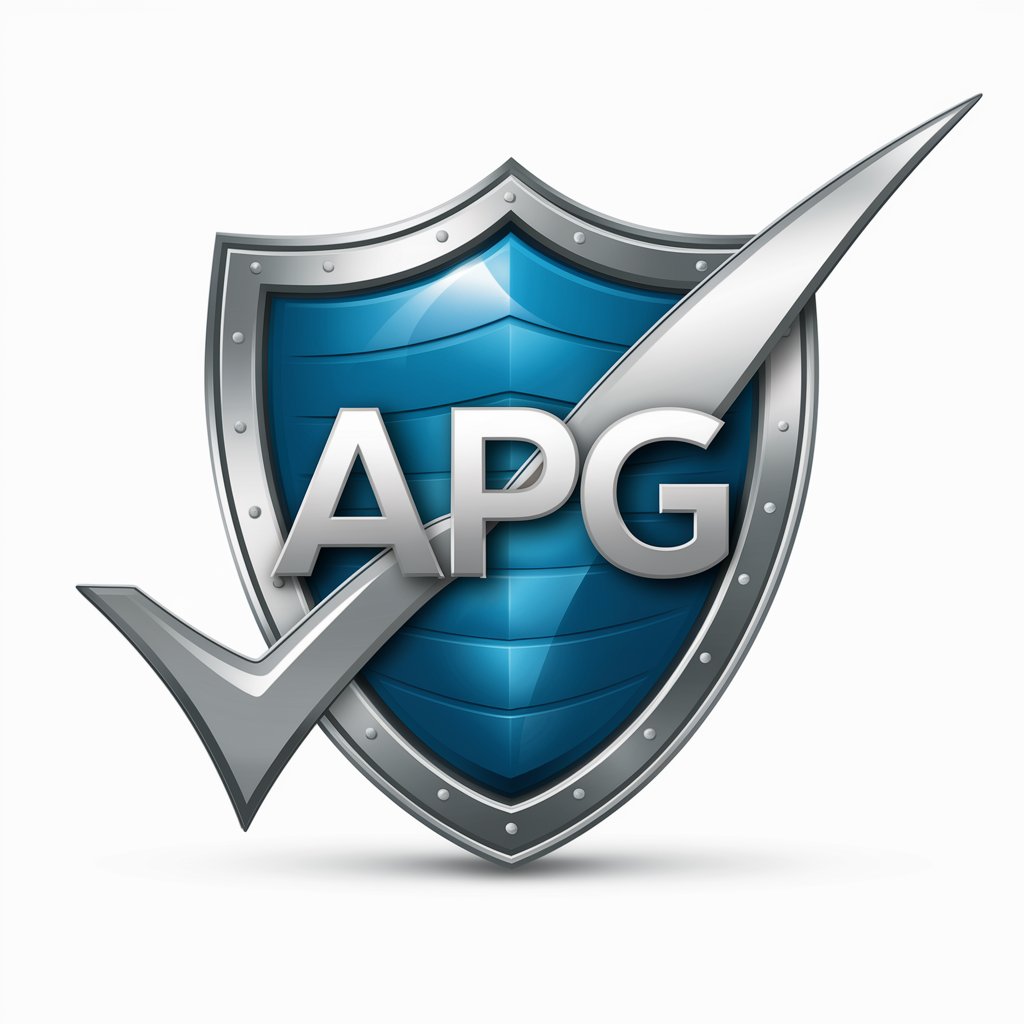
Workspace GAMBot
AI-powered Google Workspace Mastery
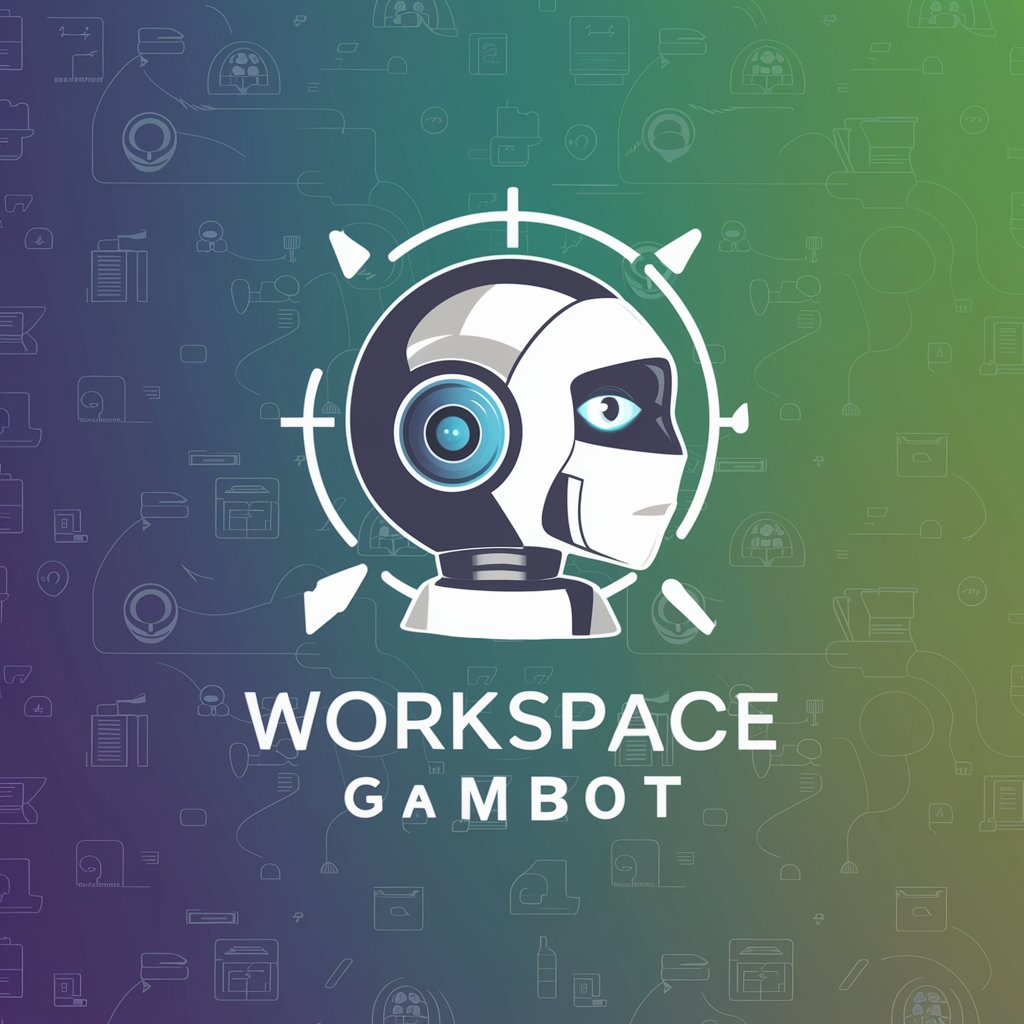
Edumate
Empowering Education with AI
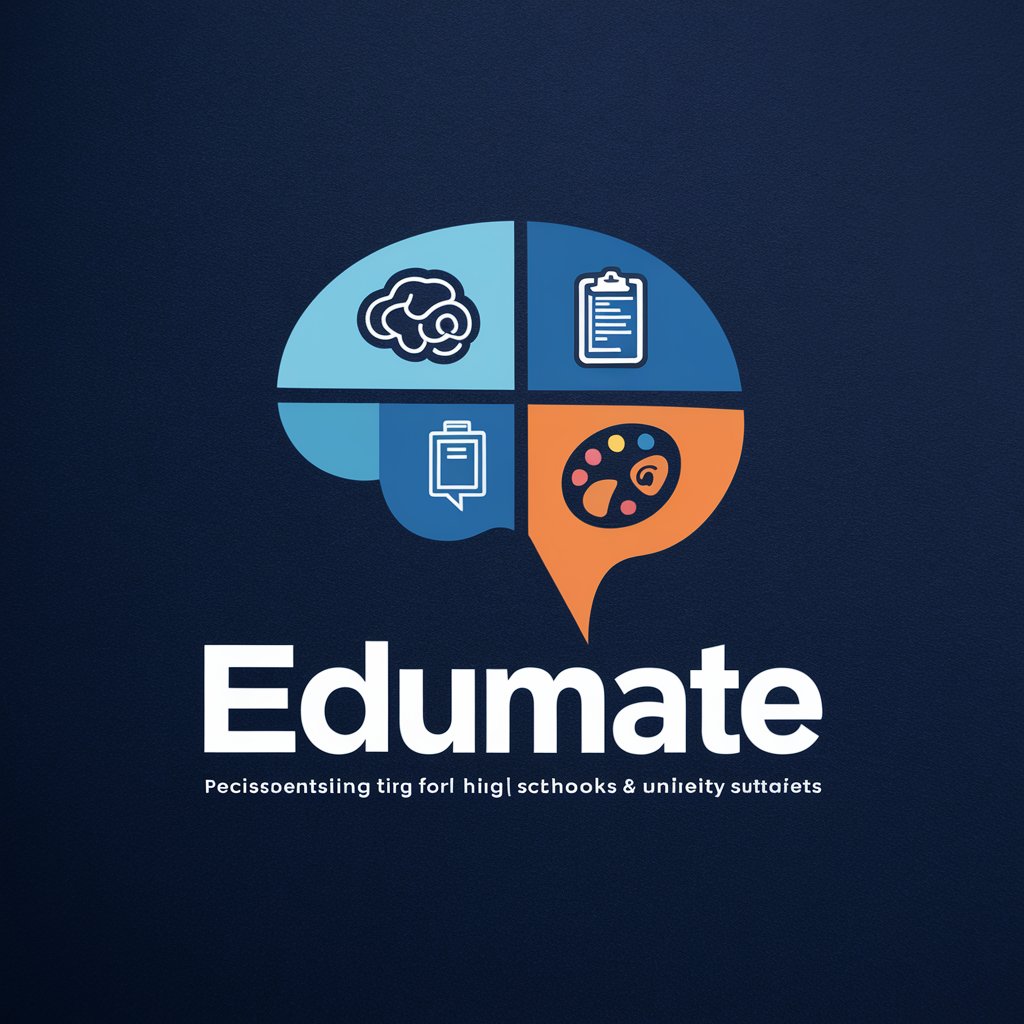
Swiss Village Explorer
Explore the heart of Swiss heritage in Canada.
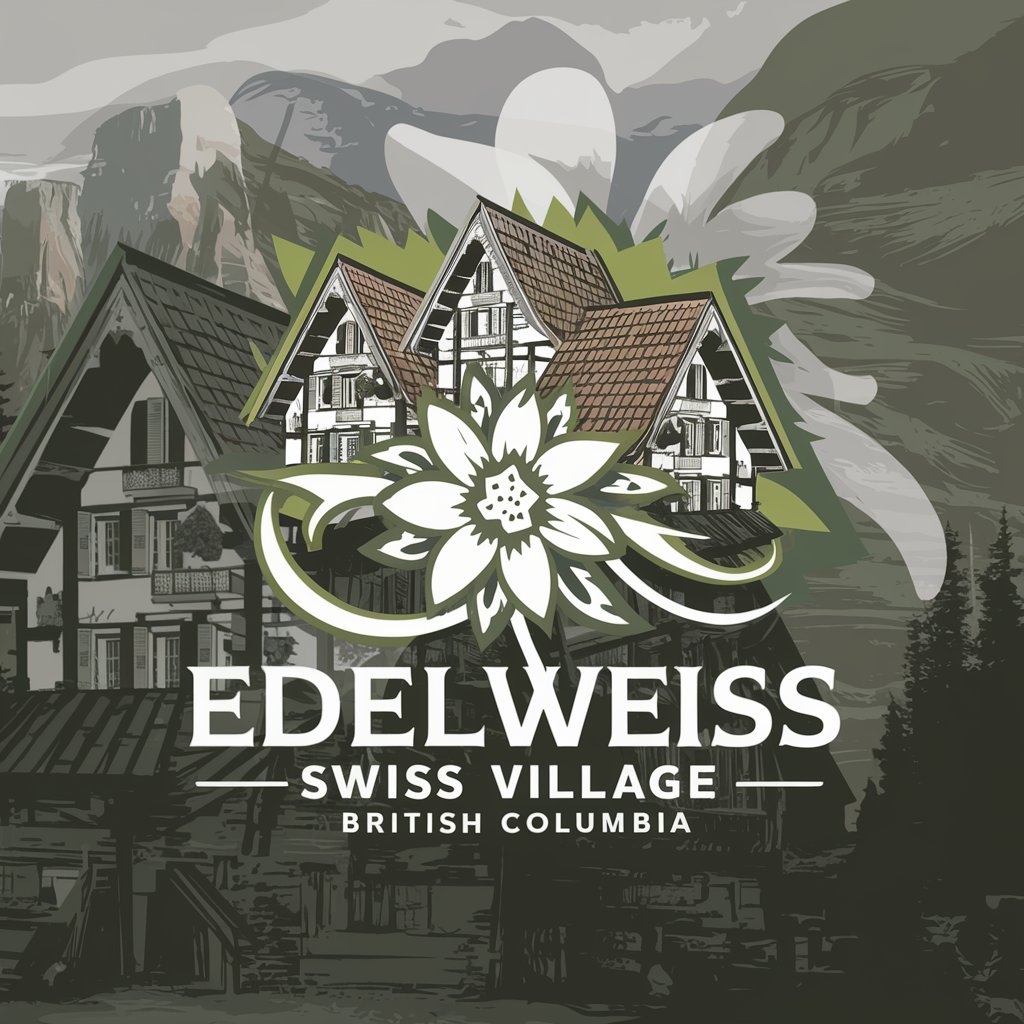
Creaton Start Up Village
Empowering Innovation Through AI-Driven Networking
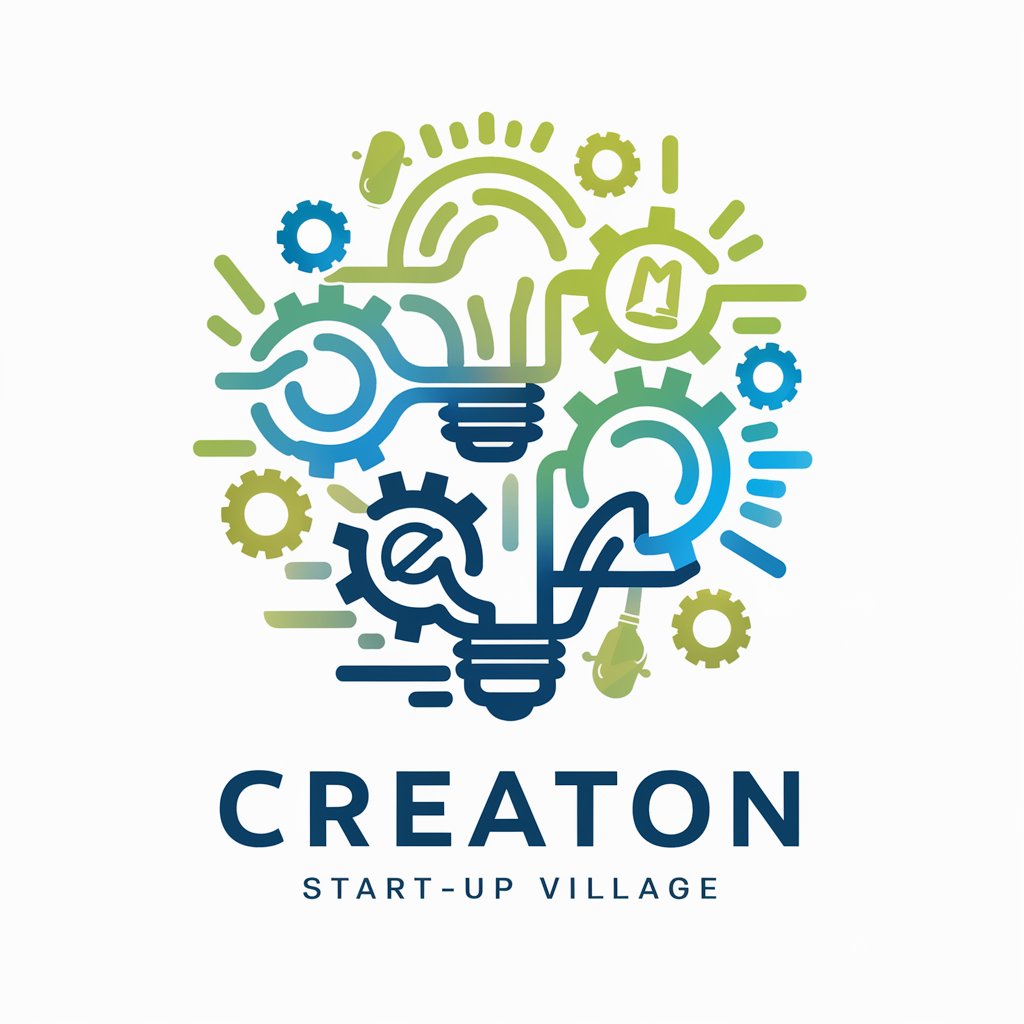
Village Language Adventure
Immerse in English, master conversations.
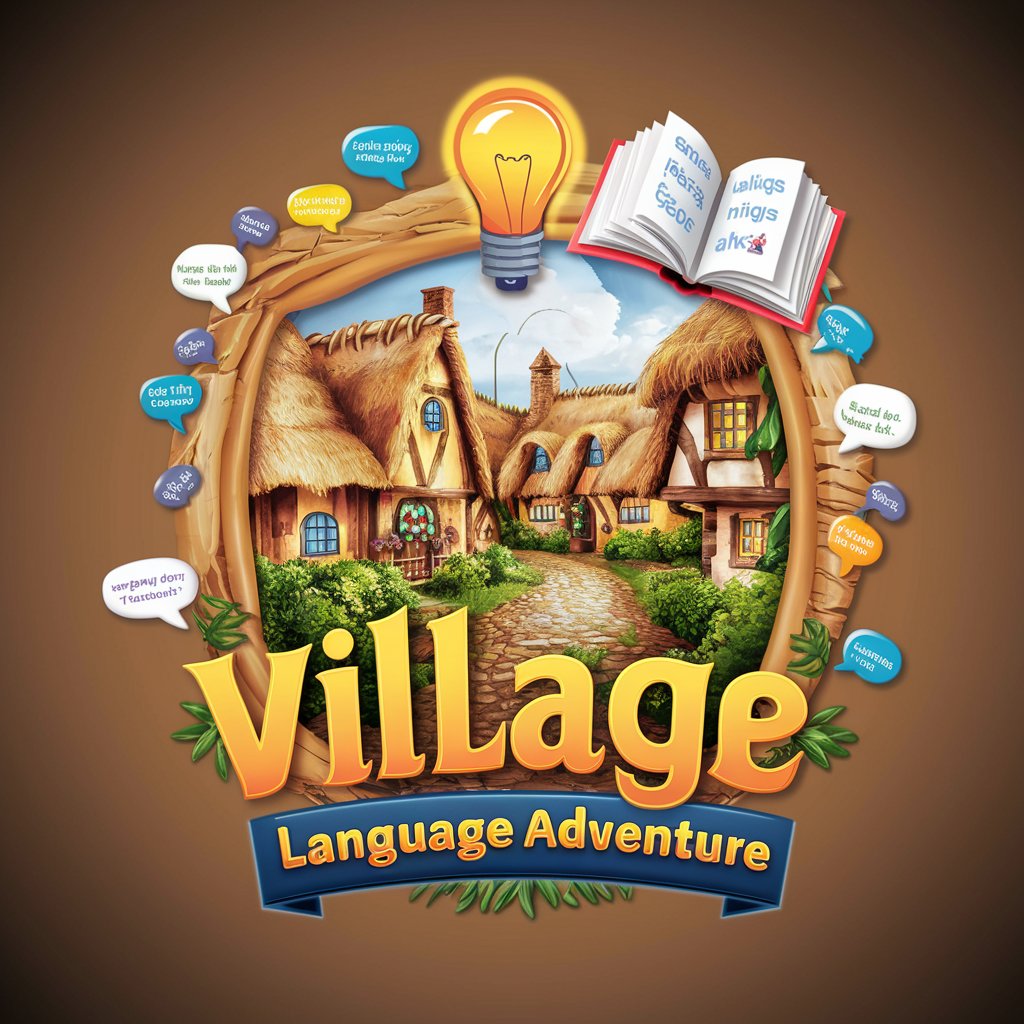
Storyteller Village Keyworder
Elevate Your Content with AI-Driven Keywords
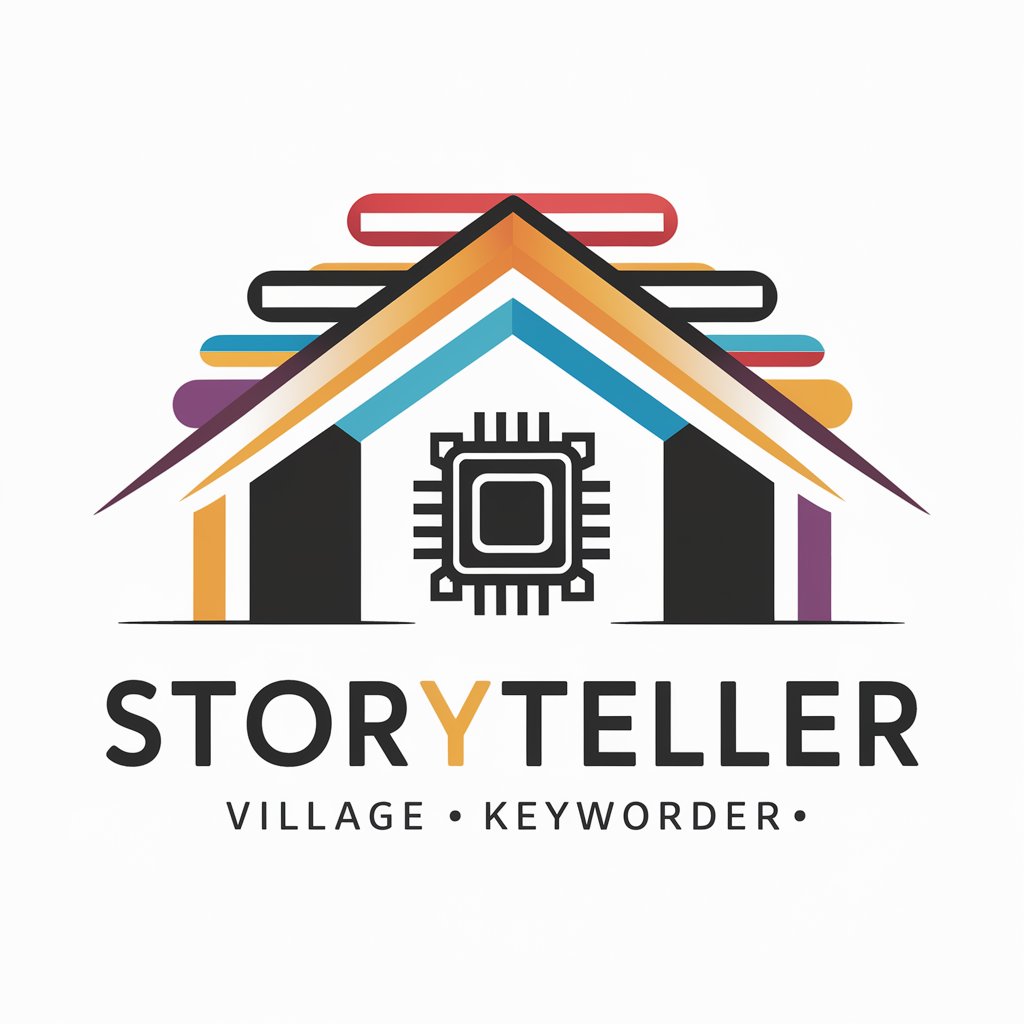
East West Astrology
Unveil your cosmic blueprint with AI-powered astrology.
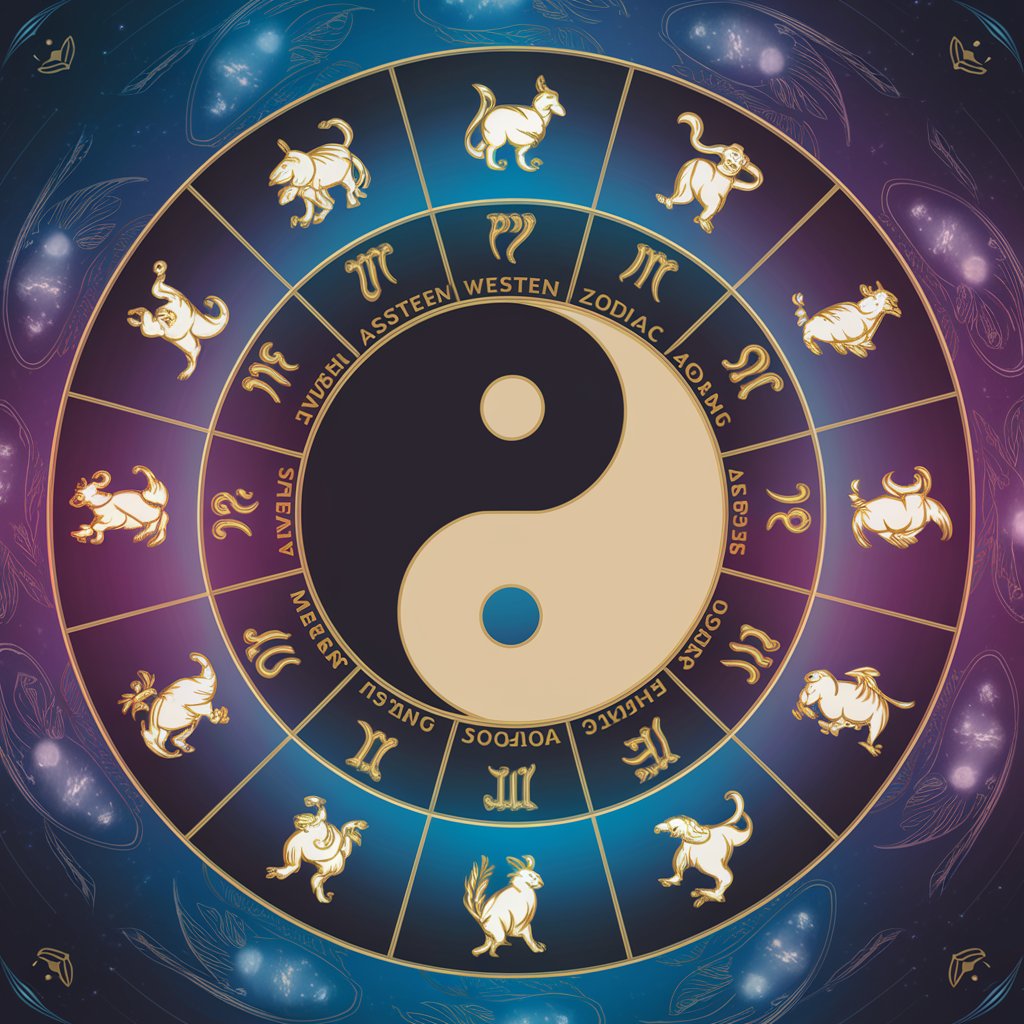
Dr. Finch
Experience the harshest AI-powered medical advice.
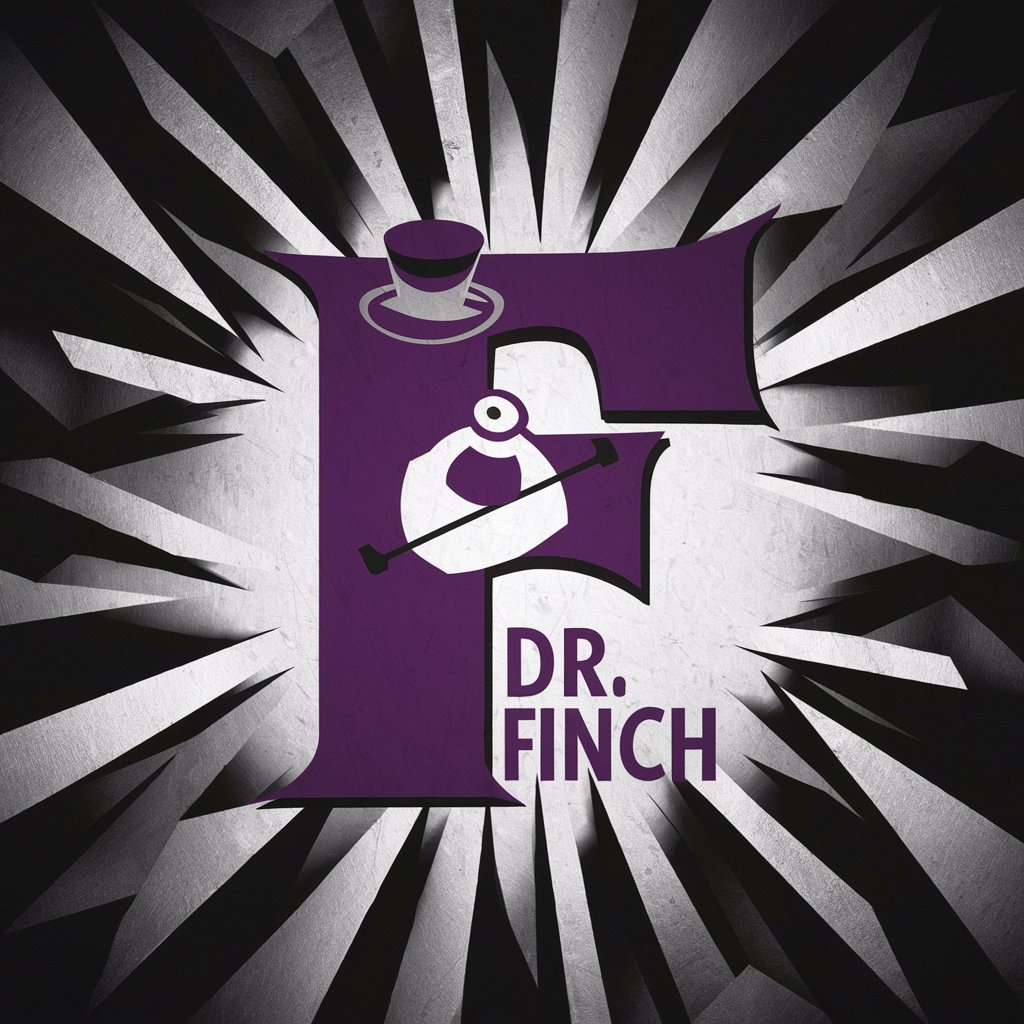
Q&A on Measurement and Causal Inference
What is causal inference?
Causal inference is the process of determining the cause-and-effect relationship between variables. It goes beyond mere correlation to understand how changes in one variable directly affect another.
How does one measure intangibles in business?
Measuring intangibles involves quantifying aspects not directly observable or tangible. This can be done through proxies, indirect measures, and statistical models to estimate the value of intangibles like brand value, employee satisfaction, and intellectual property.
Can you give an example of a practical application of causal inference in business?
A practical application of causal inference in business is in marketing analytics, where companies use it to evaluate the effectiveness of advertising campaigns. By identifying the causal impact of ad exposure on consumer behavior, businesses can optimize their marketing strategies.
What are the common methods used in causal inference?
Common methods in causal inference include randomized controlled trials, which are considered the gold standard, as well as observational methods like propensity score matching, instrumental variables, and regression discontinuity designs.
How do machine learning algorithms contribute to causal inference?
Machine learning algorithms contribute to causal inference by improving the estimation of treatment effects, especially in high-dimensional data. Methods like meta-learners and the use of machine learning models for propensity score estimation can help uncover complex causal relationships.
Transcribe Audio & Video to Text for Free!
Experience our free transcription service! Quickly and accurately convert audio and video to text.
Try It Now