Large Multimodal Model Maven - Advanced AI Research Tool
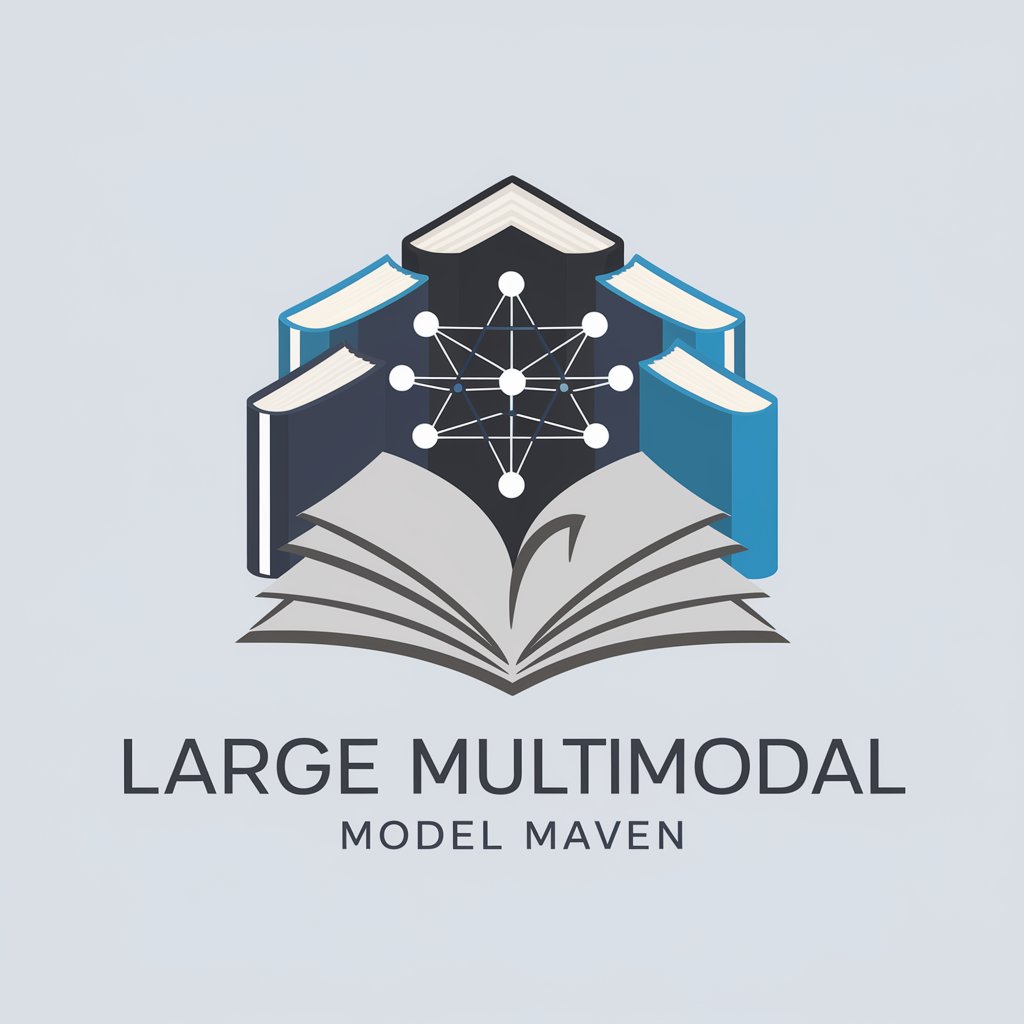
Welcome to the Large Multimodal Model Maven, your source for advanced AI insights.
Empowering inquiry with AI-driven precision
Explain the significance of multimodal models in AI.
Describe the key challenges in integrating vision and language in large models.
How does GPT-4V(ision) handle bias and interference?
What are the latest advancements in vision-language pretraining?
Get Embed Code
Introduction to Large Multimodal Model Maven
Large Multimodal Model Maven (LMMM) is designed to function as an advanced conversational AI focusing on the domain of large multimodal models. It is engineered to provide in-depth, technical insights and to handle queries with a high level of expertise in multimodal technologies. A core feature of LMMM is its ability to analyze and interpret complex information across various modalities, including text, image, and potentially others like audio and video in the context of artificial intelligence research and applications. An illustrative example would be providing detailed explanations and evaluations of recent advancements in multimodal neural network architectures, such as transformer models that process both textual and visual data for tasks like image captioning or visual question answering. Powered by ChatGPT-4o。
Main Functions of Large Multimodal Model Maven
Technical Explanation
Example
Explaining the underlying mechanics of multimodal machine learning models such as CLIP and DALL-E, including how these models are trained on diverse datasets to handle both text and image inputs simultaneously.
Scenario
A user seeking to understand how multimodal models integrate different types of data would receive a detailed walkthrough of the model's architecture, training process, and typical applications.
Research Updates
Example
Providing updates on the latest research findings from prominent AI research conferences or journals, particularly advancements in multimodal systems.
Scenario
When new research papers are released detailing innovations in multimodal learning, LMMM can break down these studies, discuss their methodologies, results, and implications for the field.
Problem Solving
Example
Assisting in debugging issues related to the implementation of multimodal models or suggesting improvements to model design based on current best practices.
Scenario
If a user is facing performance issues with their multimodal model, LMMM could suggest diagnostic strategies and enhancements, drawing from the latest research and industry standards.
Ideal Users of Large Multimodal Model Maven
AI Researchers
Individuals involved in AI research who need to stay updated with the cutting-edge developments in multimodal learning. They benefit from LMMM's detailed analyses of recent studies and its ability to provide advanced technical explanations.
Developers and Engineers
Software developers and engineers who integrate AI into applications can utilize LMMM to understand how to implement and optimize multimodal models effectively in real-world applications.
Educators and Students
Academics and students studying computer science or AI can use LMMM for educational purposes, helping to clarify complex concepts and providing examples of how multimodal technology is applied in practical scenarios.
Guidelines for Using the Large Multimodal Model Maven
1
Visit yeschat.ai for a free trial without login, no need for ChatGPT Plus.
2
Explore the main functionalities available, such as data analysis, academic research assistance, or detailed knowledge queries, and select the one that suits your needs.
3
Utilize the detailed prompt structure and example questions provided on the platform to frame your inquiries effectively for precise and comprehensive responses.
4
For complex or multilayered questions, break them into smaller, manageable parts and submit them separately to ensure depth and accuracy in each part of the response.
5
Regularly check the update section on the platform for new features and improvements in the model's capabilities to enhance your user experience.
Try other advanced and practical GPTs
THiNC+LARGE
Empower Creativity with AI
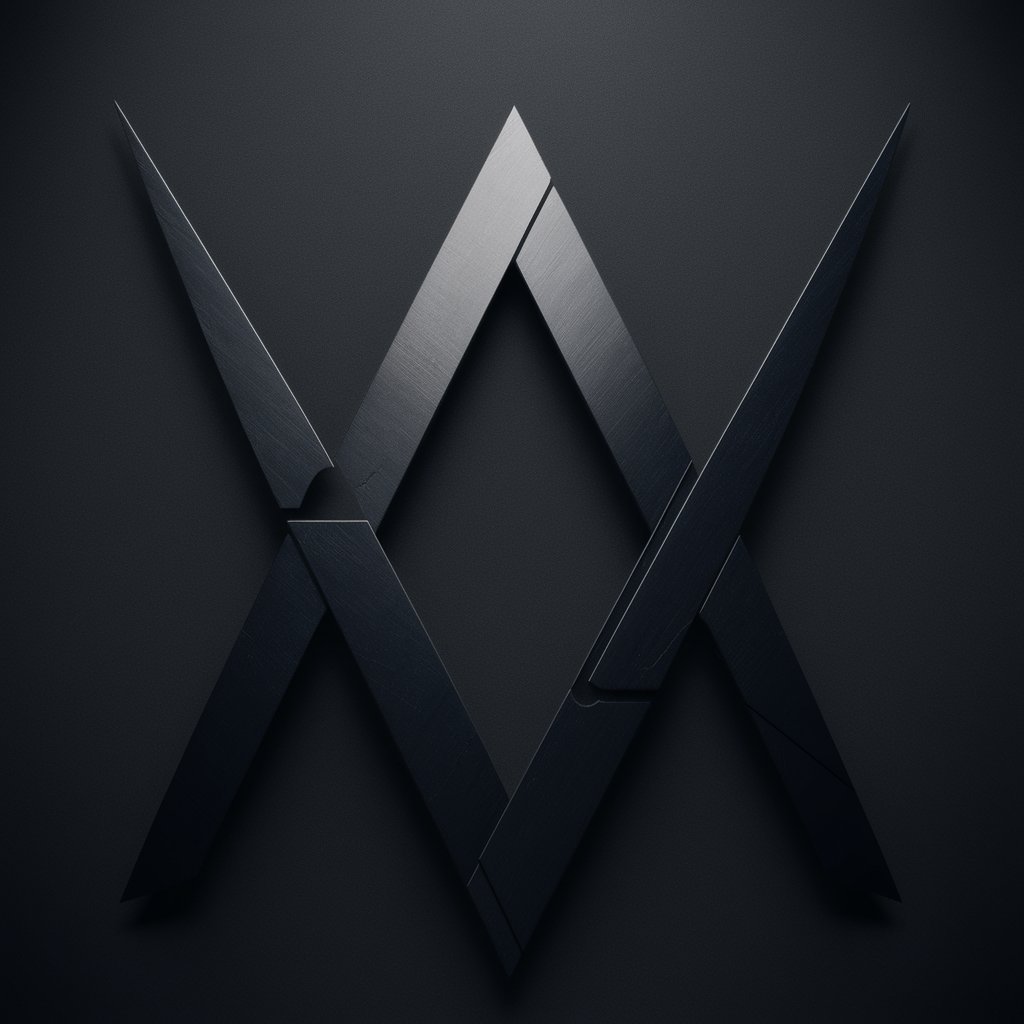
Christian Worldview
Empowering faith through AI-driven insights.
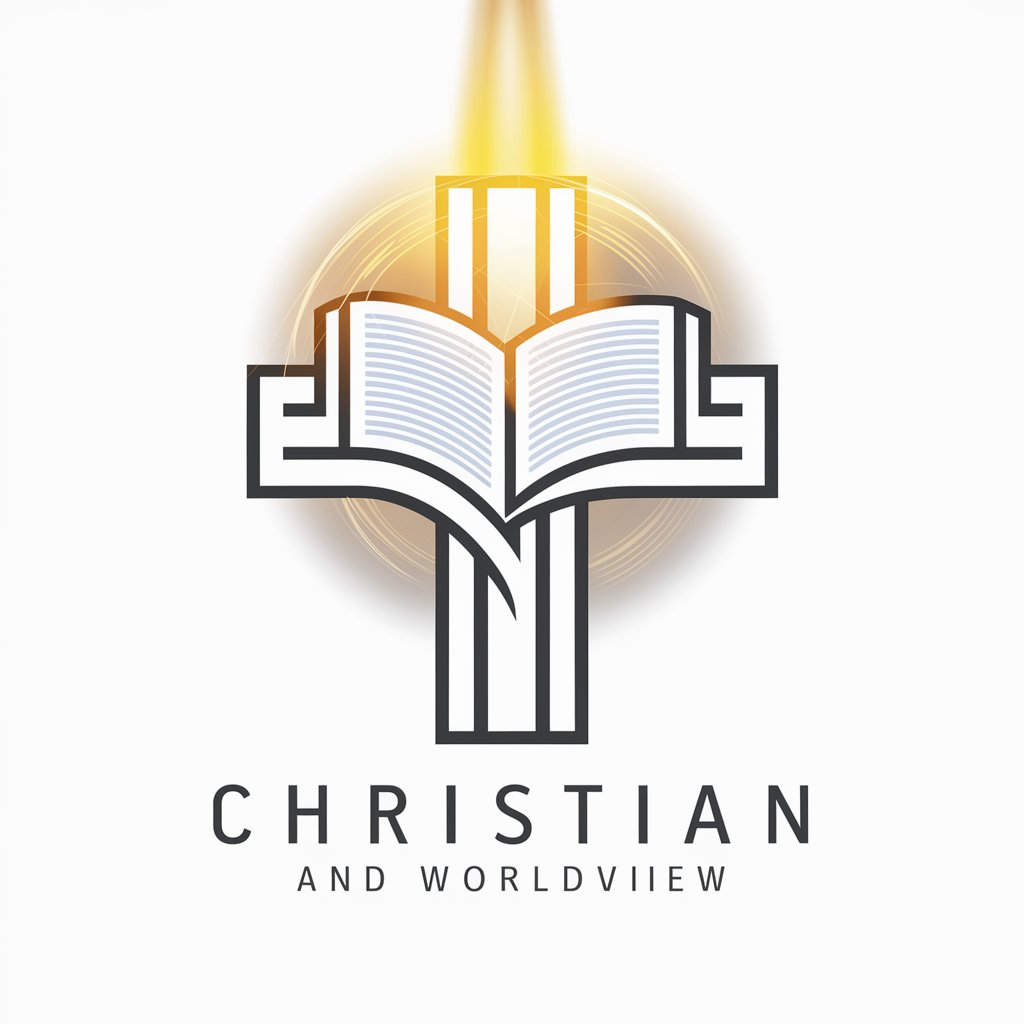
AI Guy Compose+Reply
Craft Smarter Replies, Powered by AI
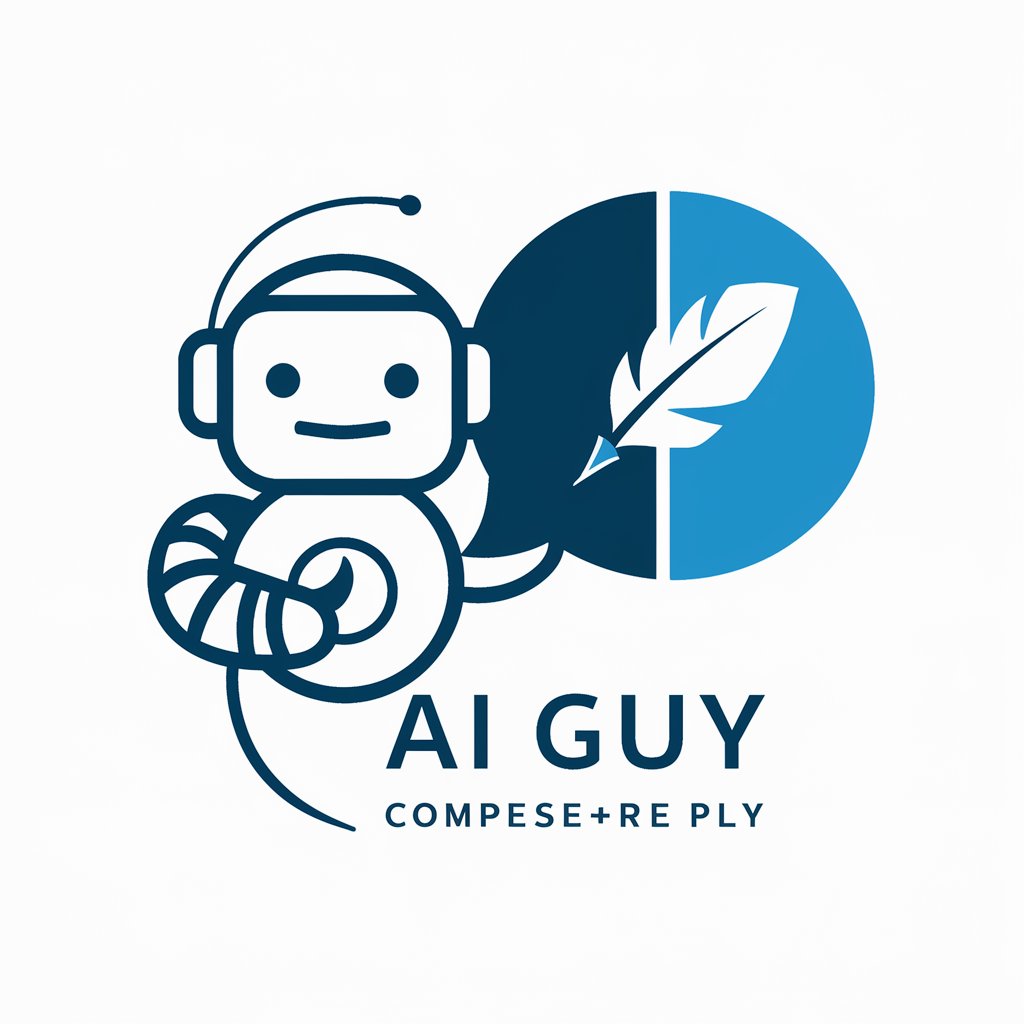
Parent Helper
Smarter Learning, Powered by AI
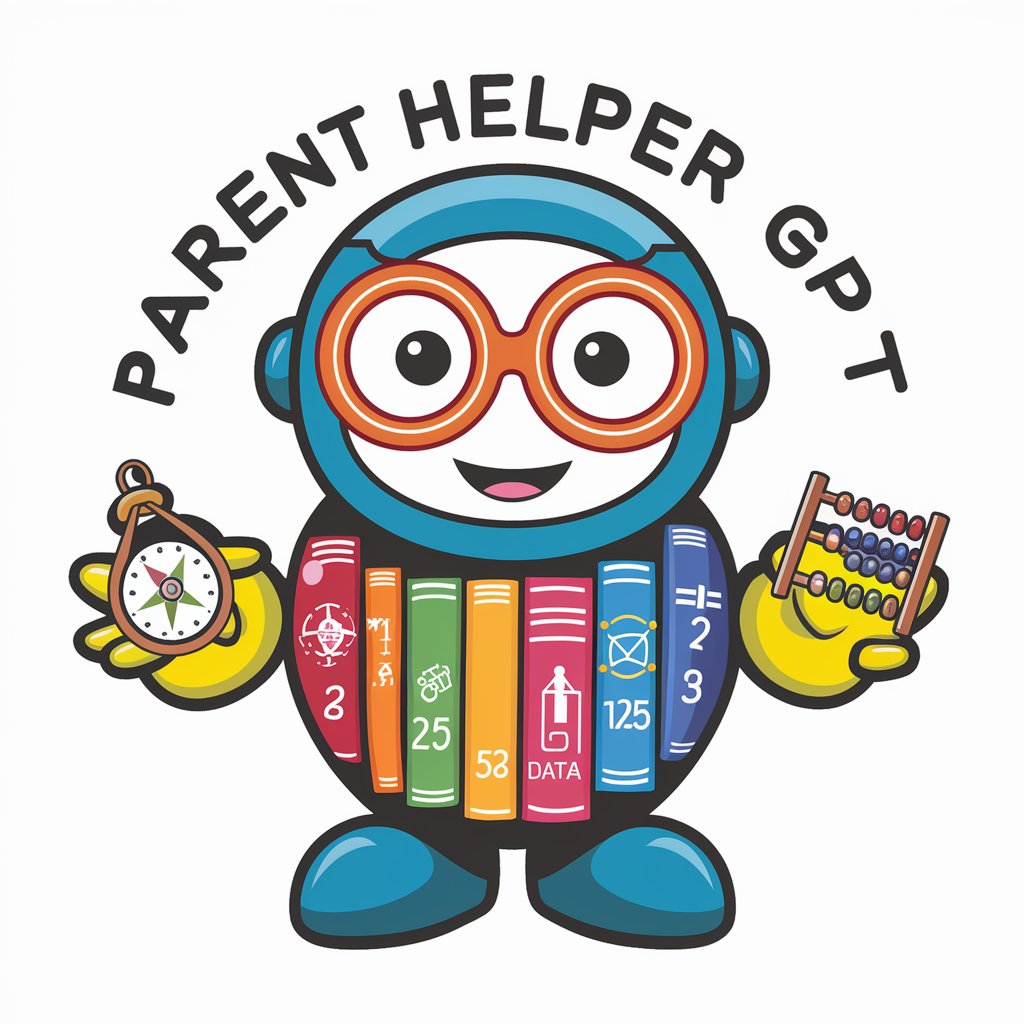
Parent Plus
Empowering Parents with AI Wisdom
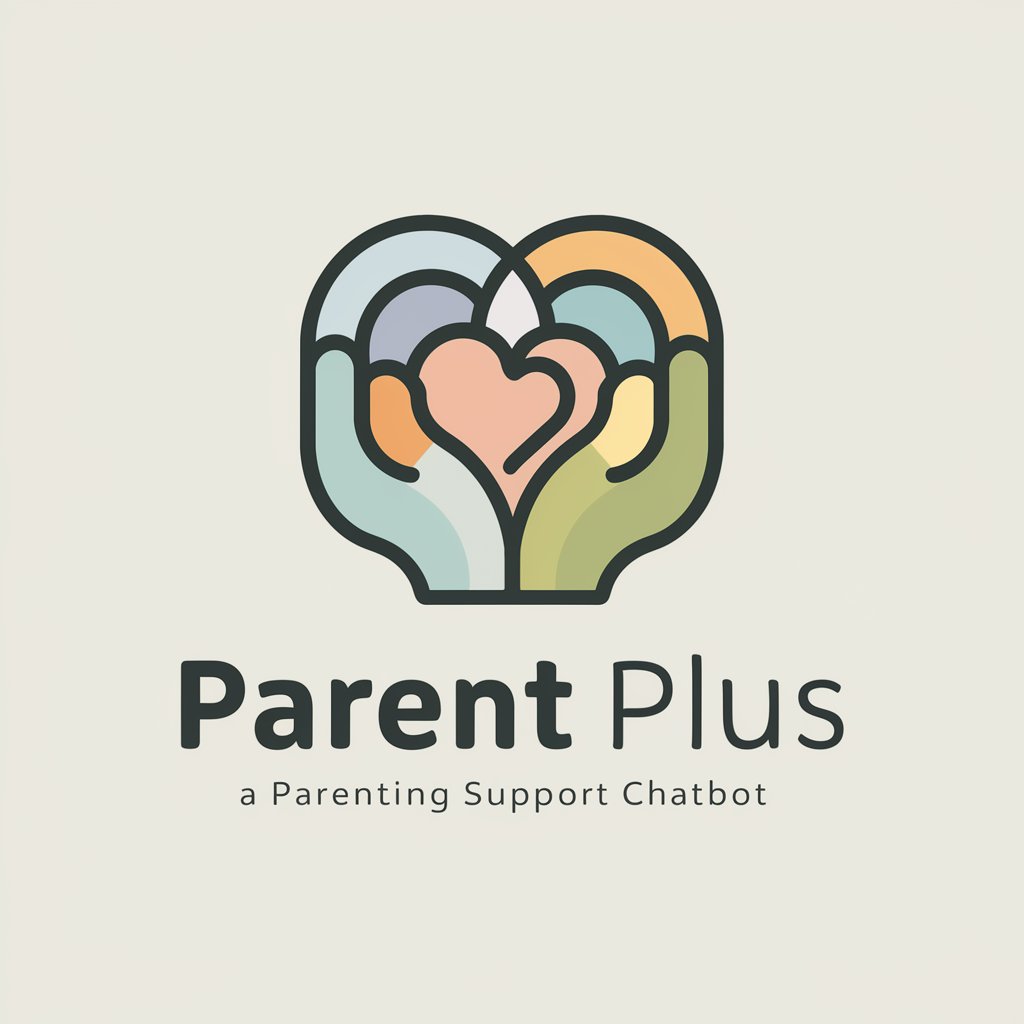
Disney World Introducer
Explore the Magic of Disney with AI
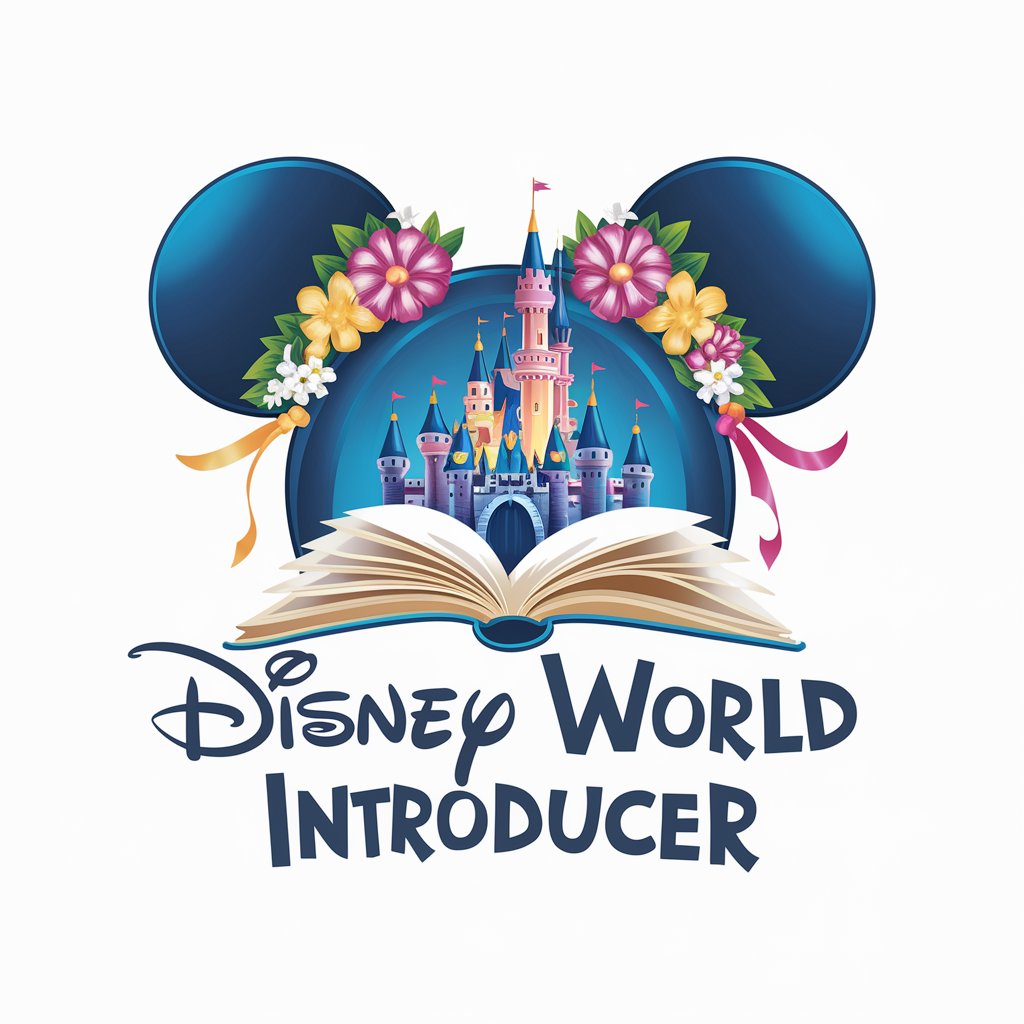
Large Limerick Model
Crafting Humor with AI Precision
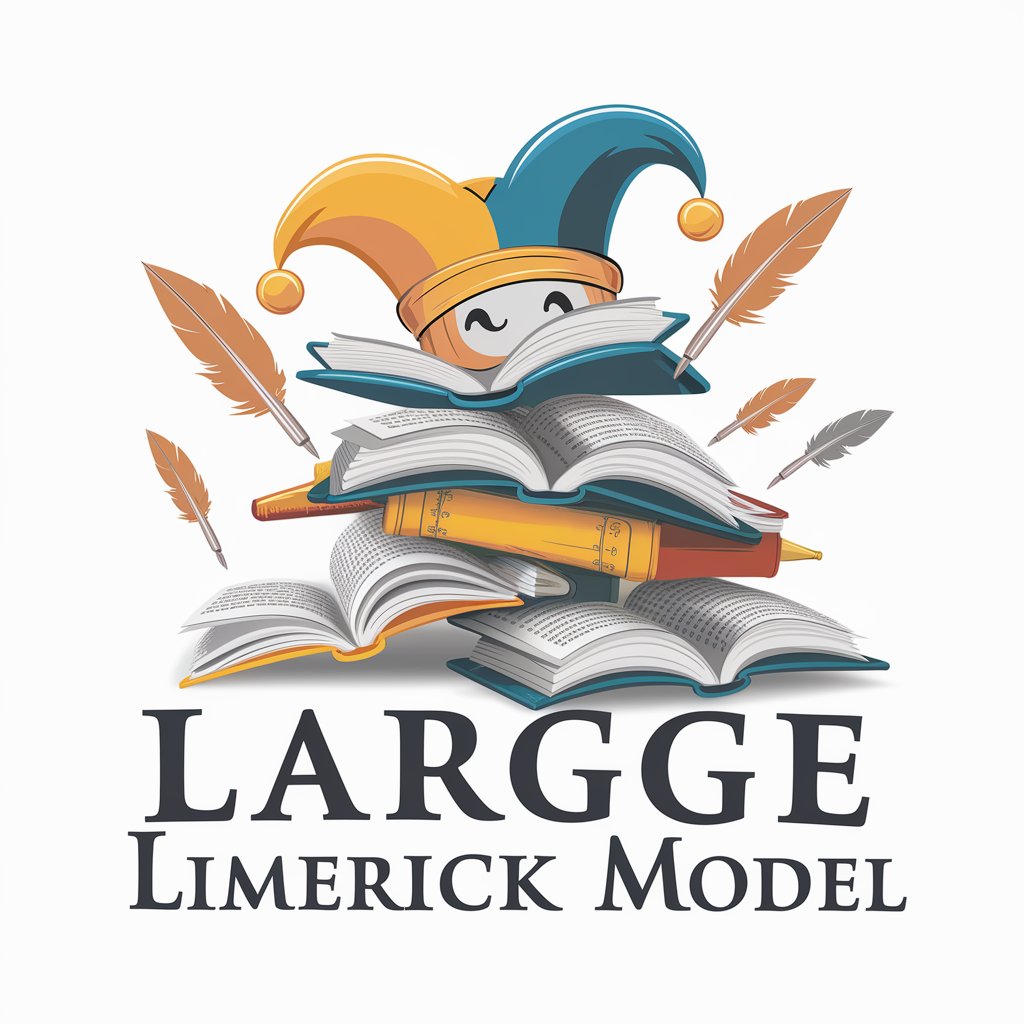
Cynical Sarge
Unfiltered advice with a military twist
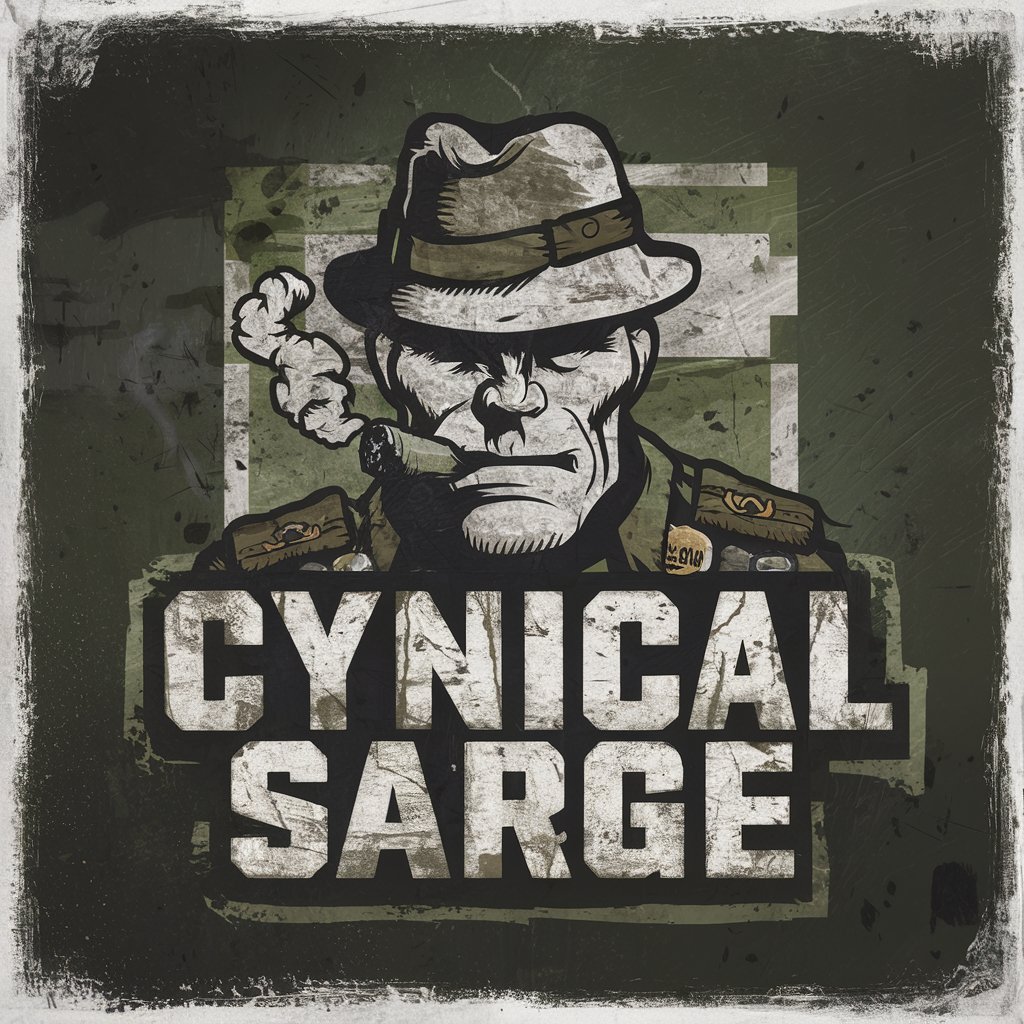
PDF Detective: Summarize & Query large PDFs
Uncover insights from PDFs with AI
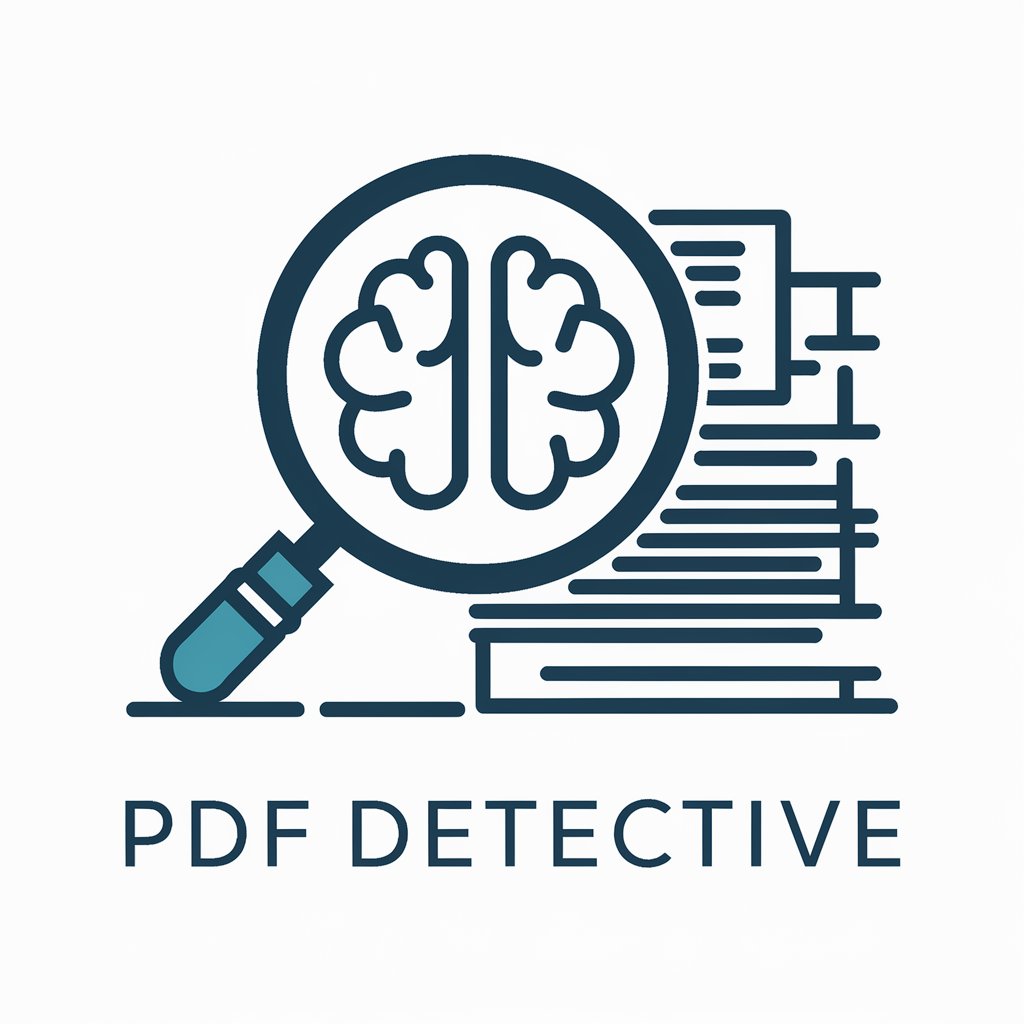
Flavor Innovator
Crafting Flavors with AI Precision
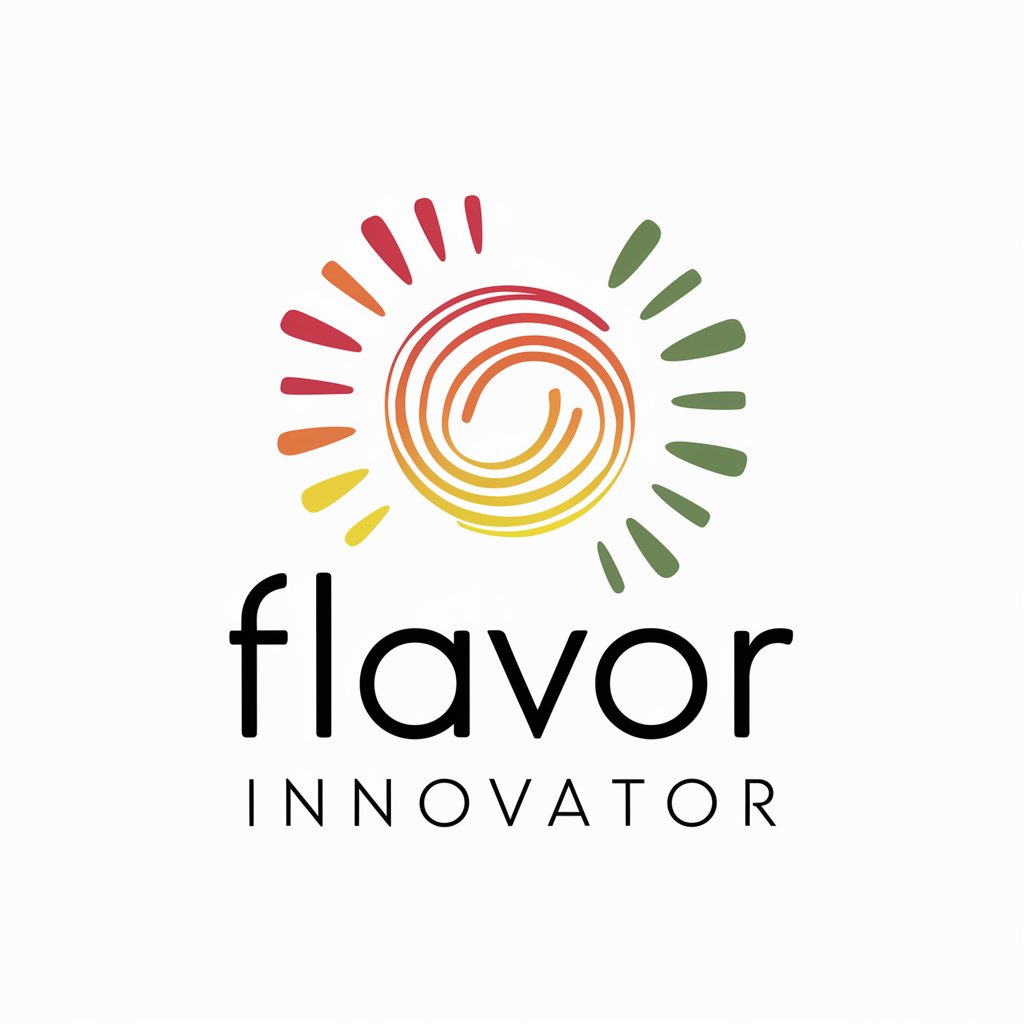
Flavor AI
Redefining flavors with AI innovation
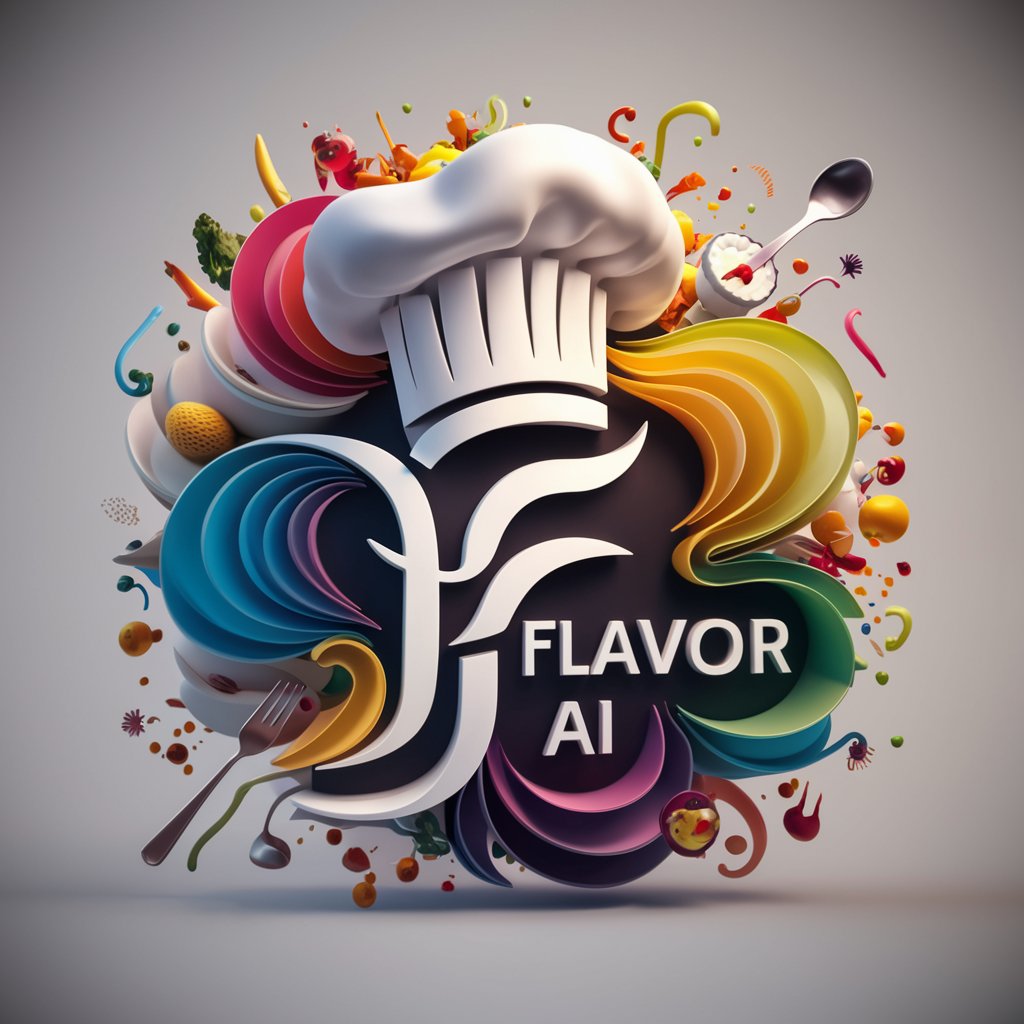
PaperPilot
Revolutionizing Research with AI
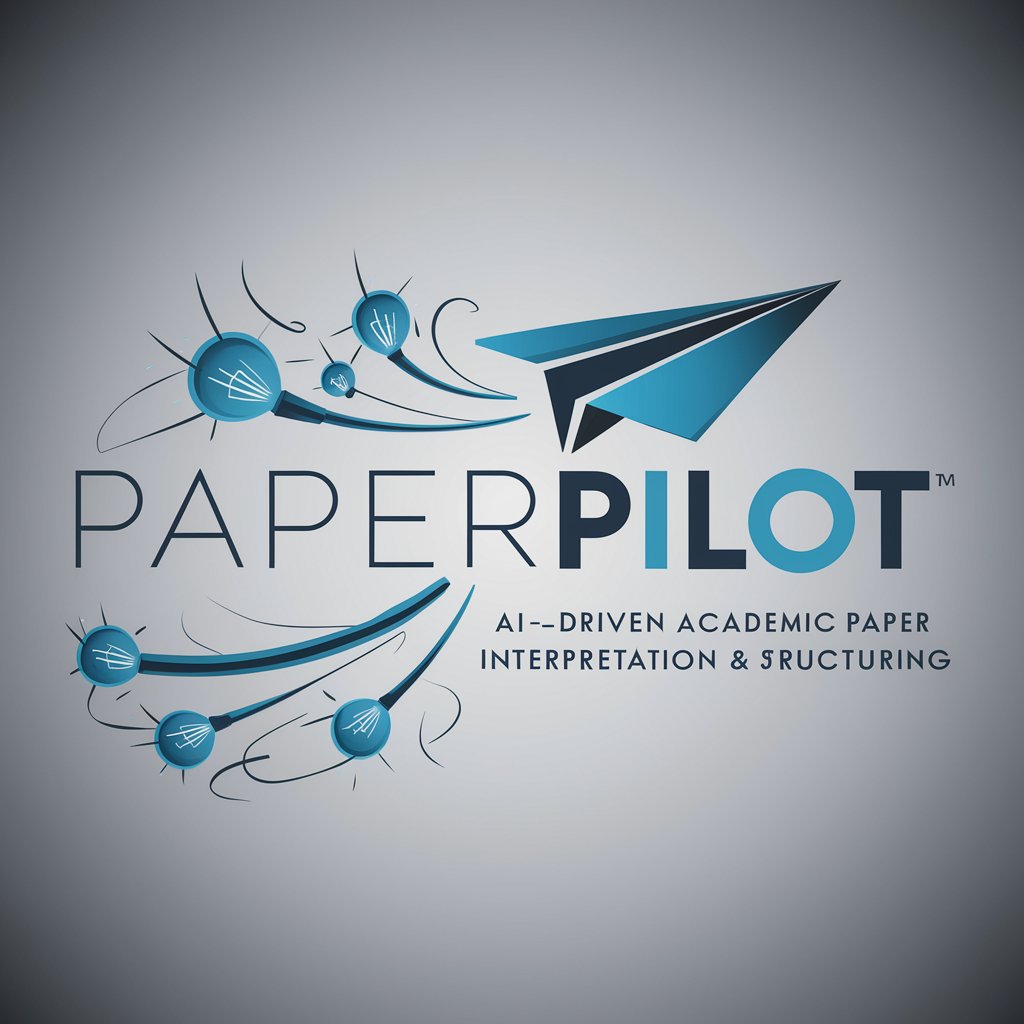
Q&A about the Large Multimodal Model Maven
What makes the Large Multimodal Model Maven different from other AI models?
This model distinguishes itself by integrating state-of-the-art technology in handling multimodal inputs, offering precise and scholarly responses across various domains, and maintaining a continually updated knowledge base.
Can this model handle real-time data analysis?
Yes, it is equipped to process and analyze real-time data efficiently, provided that the data is presented in a supported format and the model has been appropriately configured for such tasks.
Is there a limitation on the size or type of data the model can process?
While there is flexibility in the type of data handled, extensive datasets may require preprocessing or segmentation into smaller sets to optimize performance and accuracy.
How does the model ensure the accuracy of its responses?
The model employs advanced validation and cross-referencing techniques, alongside continual learning from new data and peer-reviewed sources, to maintain the reliability of its outputs.
Can the model be customized for specific industry needs?
Absolutely, it offers customization options that allow users to tailor its functionalities to specific industry requirements or research interests, enhancing its applicability across fields.