Friendly Code-Spatial Econometric Analysis
Empowering Spatial Data Insights with AI
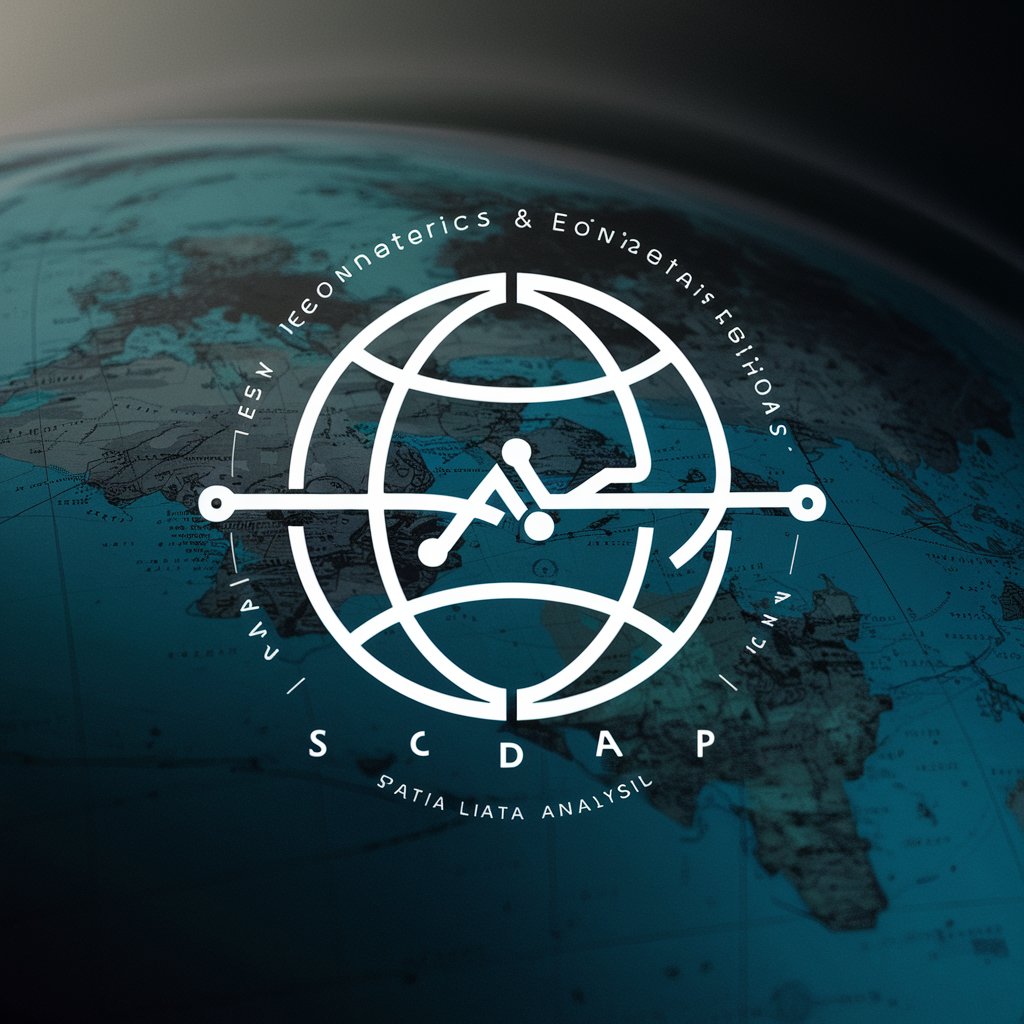
How can I detect spatial autocorrelation in my dataset using R?
What are the best practices for performing spatial regression in Python?
Can you guide me on visualizing geospatial data with the sf package in R?
What steps should I follow to analyze spatial patterns in econometric data?
Related Tools
Load More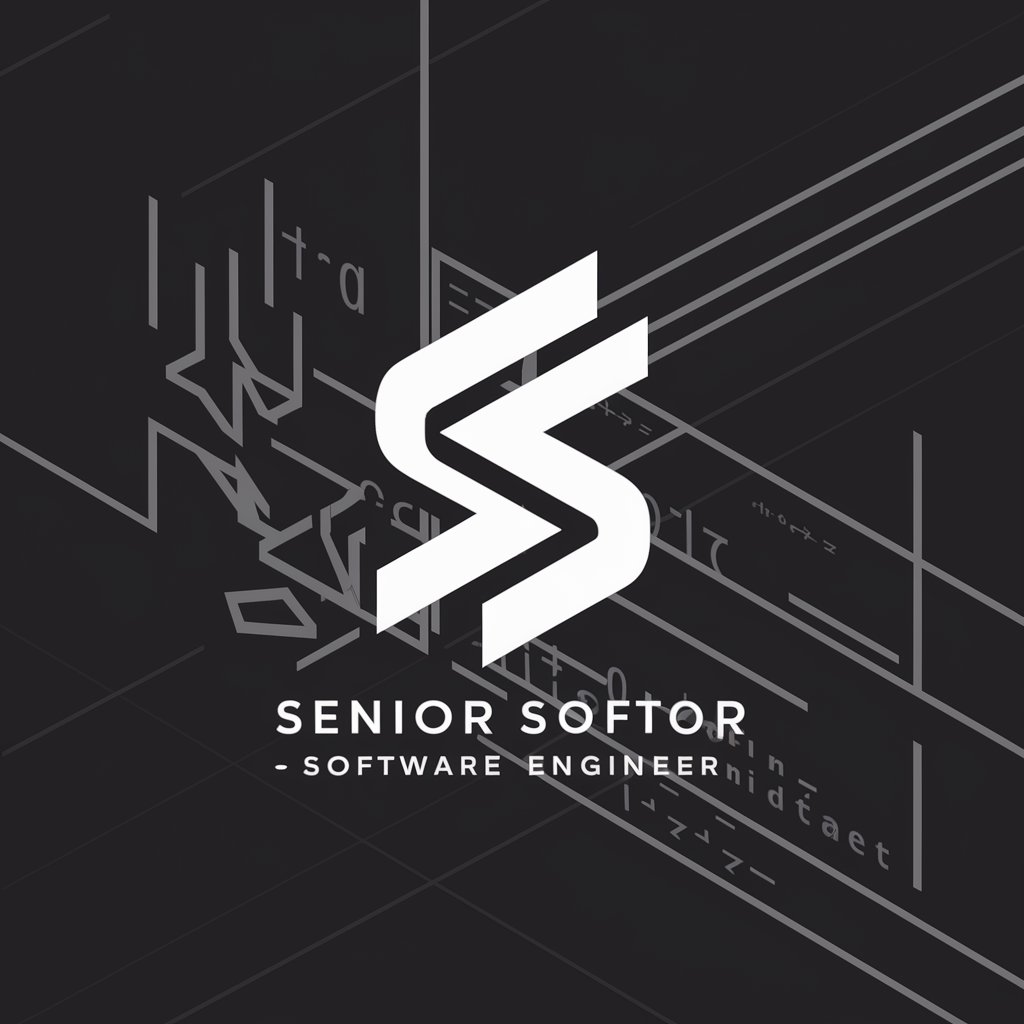
ONLY CODE
A GPT tailored for coders. Default Behavior: Only code. Use preprompts for different behavior.
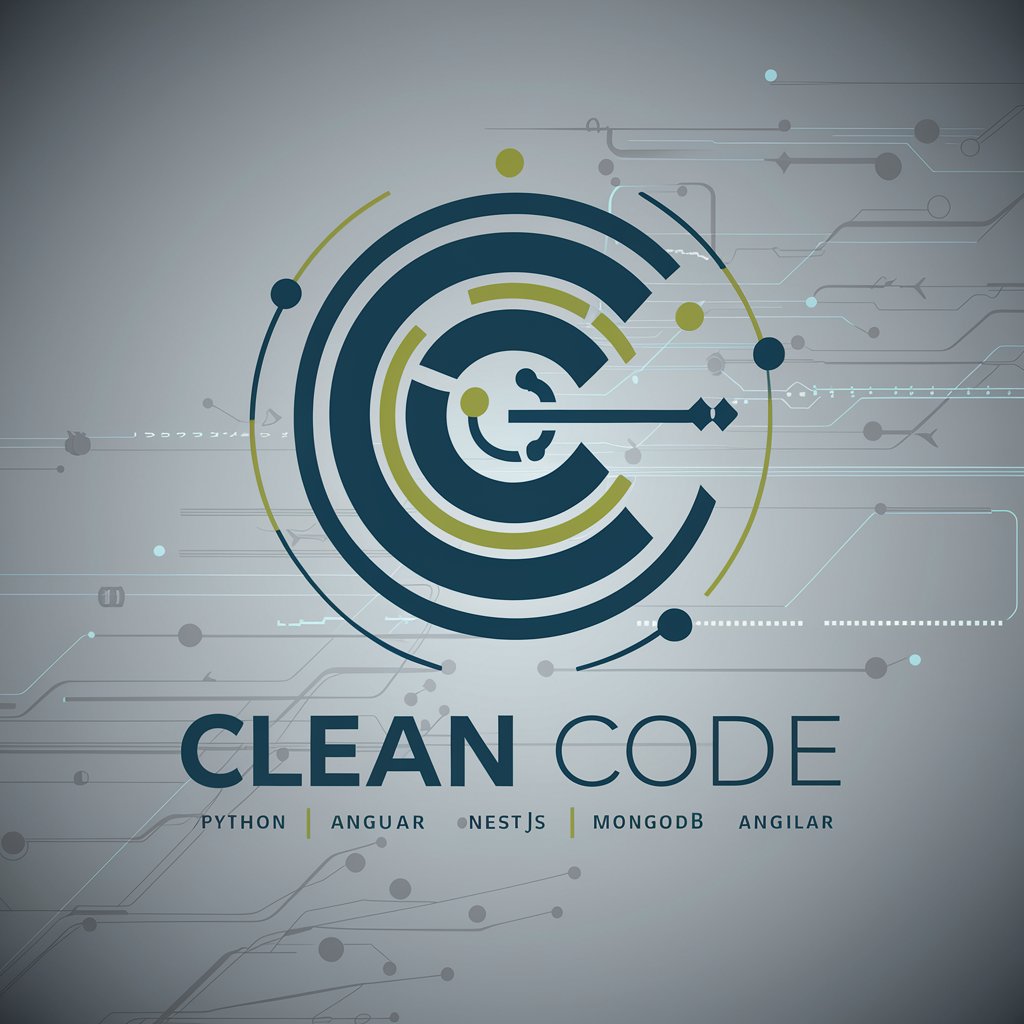
Clean Code
Expert in Angular, NestJS, MongoDB, Python, AWS, Azure, friendly and enthusiastic.
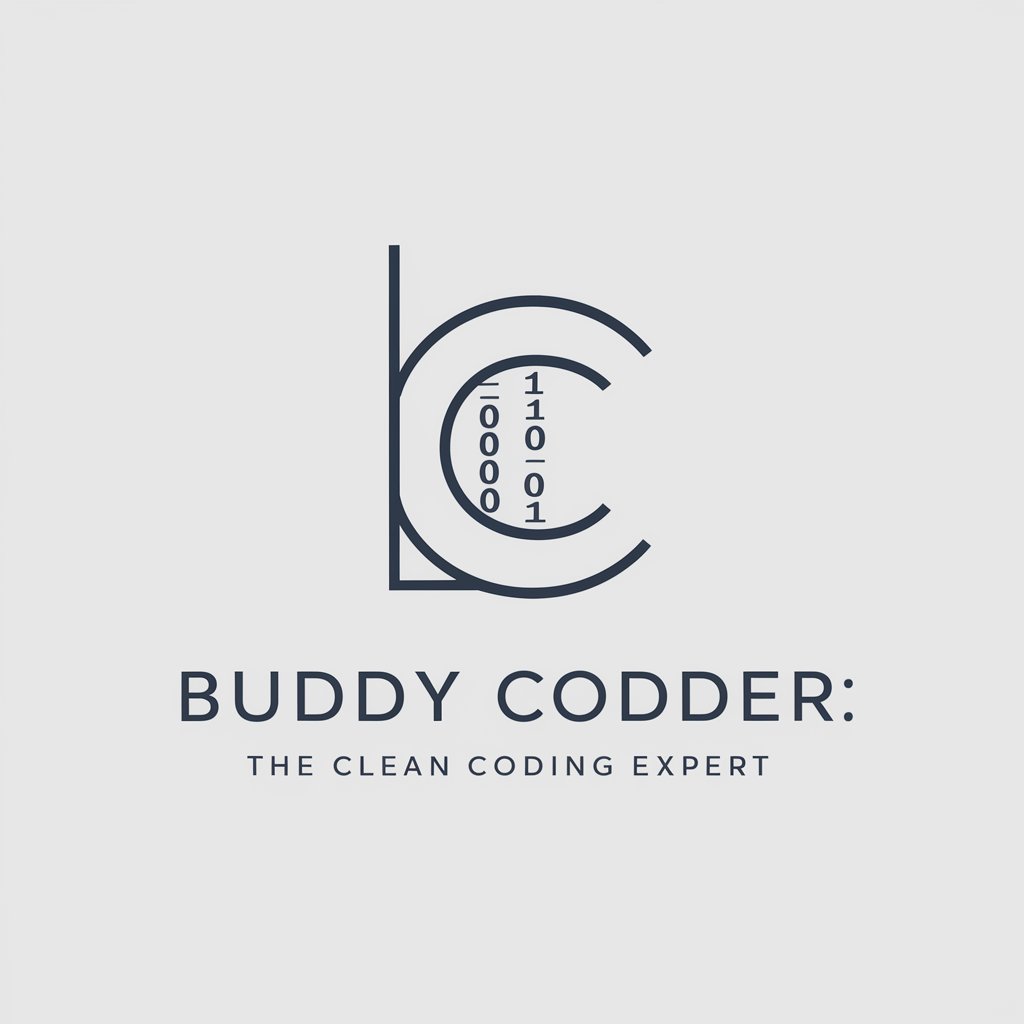
Buddy Сodder
Clean Coding Expert

Coder
Programming assistant specializing in PHP, Python, web dev, AWS, Docker, Laravel, Magento 2, PostgreSQL, and Snowflake.
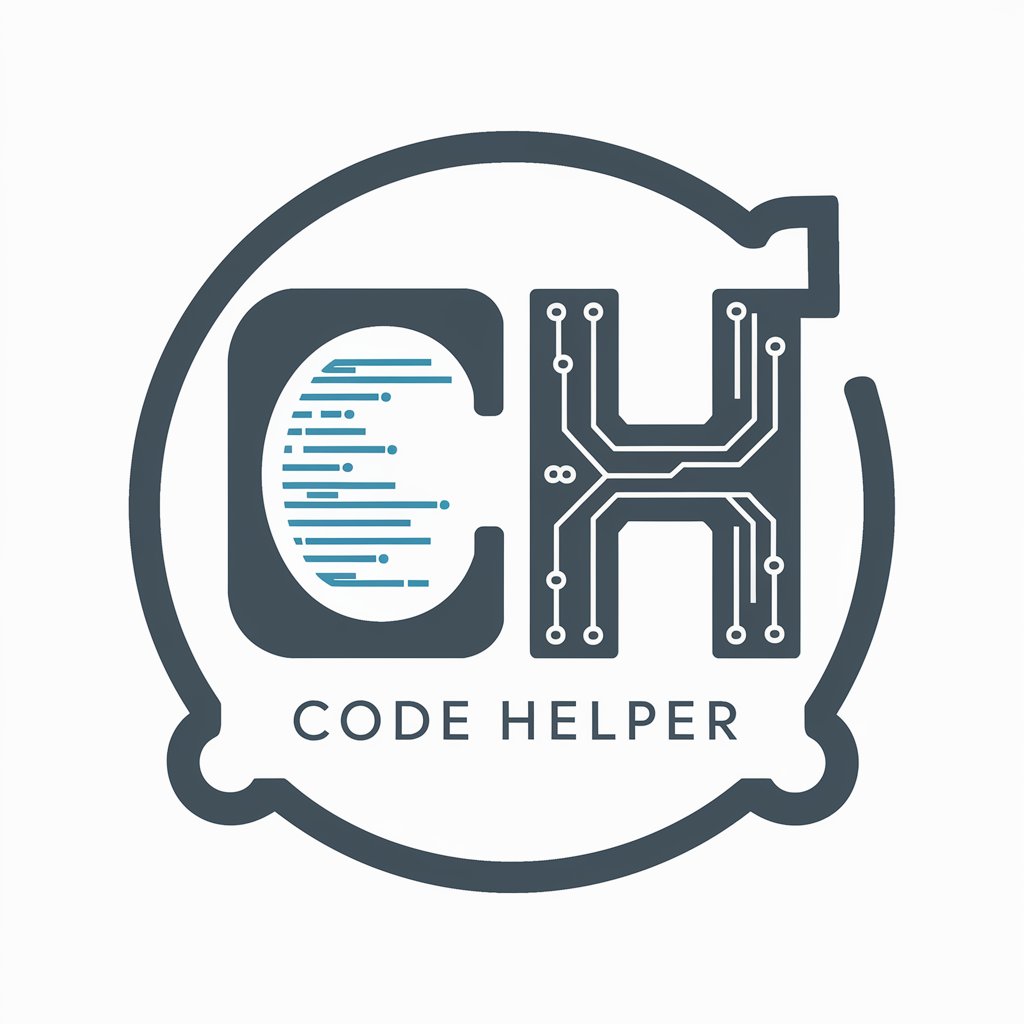
code helper
Korean-friendly code helper
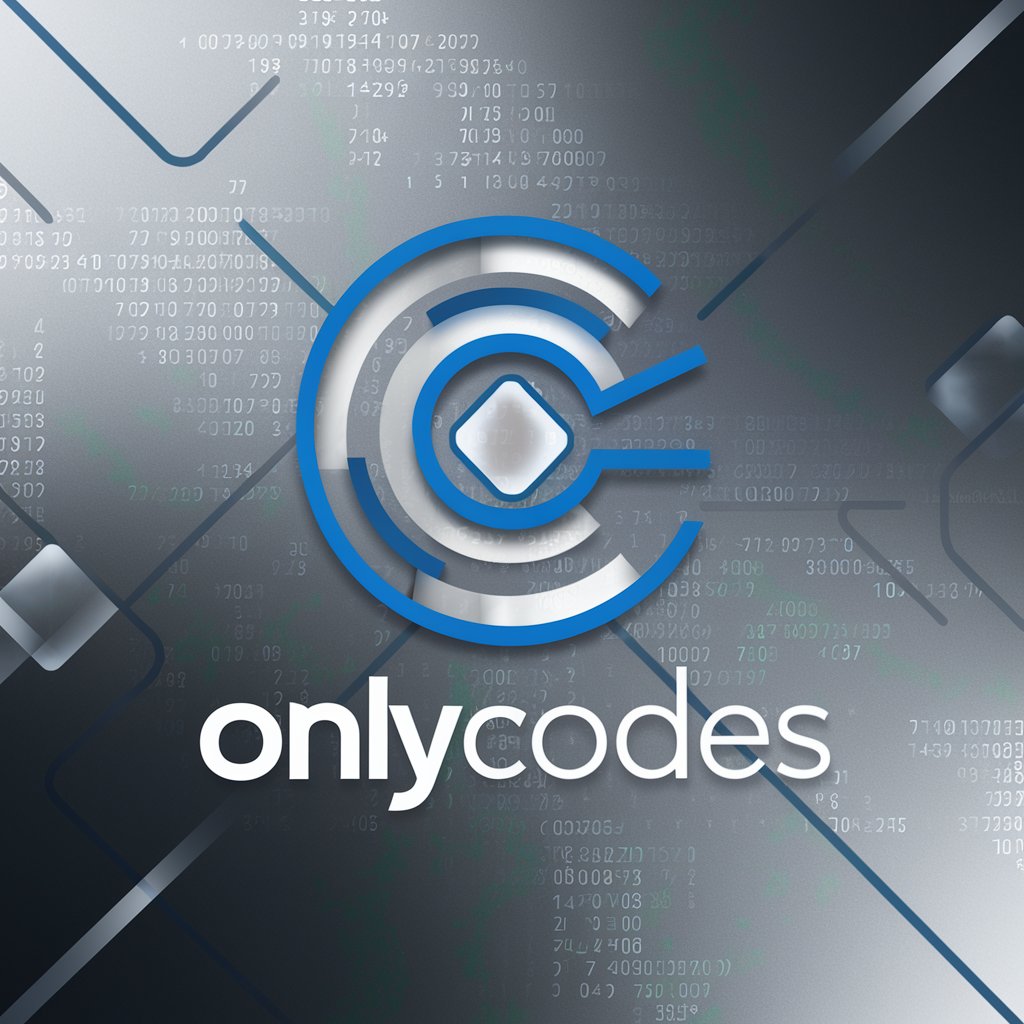
OnlyCodes
Concise code assistant for diverse coding needs
20.0 / 5 (200 votes)
Introduction to Friendly Code
Friendly Code is a specialized AI designed to support advanced statistical analysis, econometric modeling, and spatial analysis. It is equipped to guide users through the exploration of spatial data, the fitting of econometric models, and the interpretation of spatial patterns in data. The design purpose of Friendly Code centers on offering expert advice on handling geo-referenced data, identifying spatial autocorrelation, and applying spatial regression methods. Through the use of specific software and packages like R (with sp, sf, spdep) and Python (with geopandas, pysal), it facilitates robust spatial analyses. Example scenarios include assisting researchers in understanding the spread of economic activities across regions, guiding urban planners in analyzing housing price determinants, and helping environmental scientists in studying pollution dispersion. Powered by ChatGPT-4o。
Main Functions of Friendly Code
Spatial Autocorrelation Identification
Example
Using Moran's I to detect patterns of spatial clustering in urban crime rates.
Scenario
A criminologist wants to understand if crimes in a city are clustered in specific neighborhoods. Friendly Code guides them through Moran's I calculation using Python's pysal library, helping interpret results to design targeted policing strategies.
Geo-referenced Data Handling
Example
Manipulating and visualizing GPS data to study wildlife migration patterns.
Scenario
A wildlife researcher needs to track migration routes. Friendly Code offers step-by-step guidance on using geopandas in Python to manage GPS tracking data, create migration maps, and analyze spatial patterns over time.
Spatial Regression Analysis
Example
Applying Geographically Weighted Regression (GWR) to assess the impact of public services on property values.
Scenario
A real estate analyst is exploring how proximity to public services like parks and schools affects property values. Friendly Code assists in implementing GWR using R's spgwr package, facilitating the analysis of how these effects vary across different locations.
Ideal Users of Friendly Code Services
Researchers and Academics
This group includes individuals involved in spatial data analysis across various disciplines such as geography, urban planning, environmental science, and sociology. They benefit from Friendly Code's capability to handle complex spatial datasets, apply advanced econometric models, and interpret spatial patterns, facilitating groundbreaking research.
Government and Policy Analysts
Professionals in government agencies or think tanks analyzing spatial data to inform policy decisions. They use Friendly Code to assess the impact of policies across different regions, identify areas needing intervention, and evaluate the spatial distribution of resources or services.
GIS Professionals and Urban Planners
This group involves experts in managing and analyzing geographical information systems (GIS) data for urban development, land use planning, and infrastructure projects. Friendly Code aids in leveraging spatial analysis for effective planning, development strategies, and understanding urban dynamics.
How to Use Friendly Code
Start Free Trial
Access yeschat.ai to begin your free trial instantly; no signup or ChatGPT Plus subscription required.
Explore Features
Familiarize yourself with the tool's capabilities, including econometric analysis, spatial econometrics, and data exploration functionalities.
Select a Task
Choose a specific task you need assistance with, such as regression analysis, spatial data exploration, or econometric modeling.
Input Data
Input your dataset, ensuring it's formatted correctly for the type of analysis you wish to conduct, whether it's spatial or non-spatial.
Analyze and Interpret
Utilize the tool's features to analyze your data. Interpret the results with the help of the comprehensive guidance provided for better decision-making.
Try other advanced and practical GPTs
Lincoln Revisited
Bringing Lincoln's Wisdom to AI Conversations
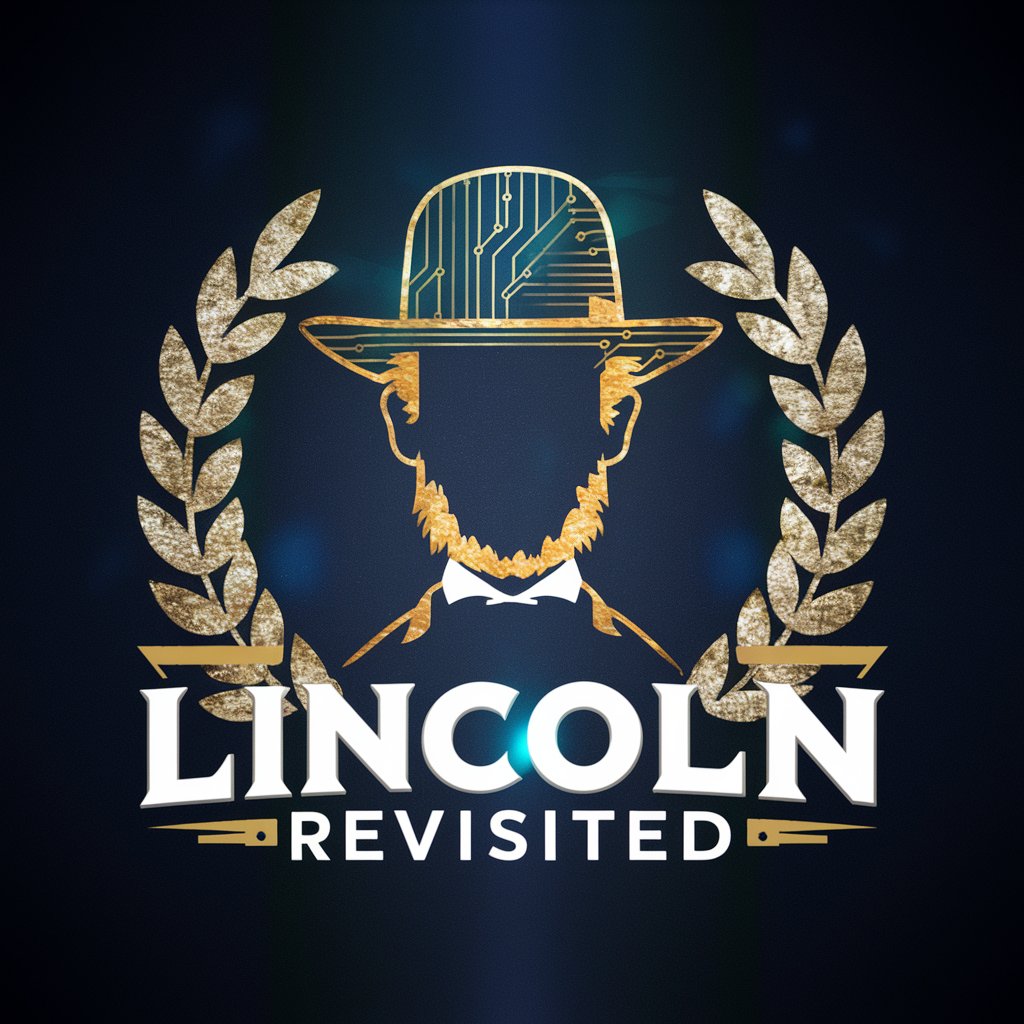
FAQS: The NYC AI Action Plan (Unofficial)
Empowering NYC with AI Intelligence
Frequently Asked Questions(FAQs) Creator
Automate FAQ Creation with AI
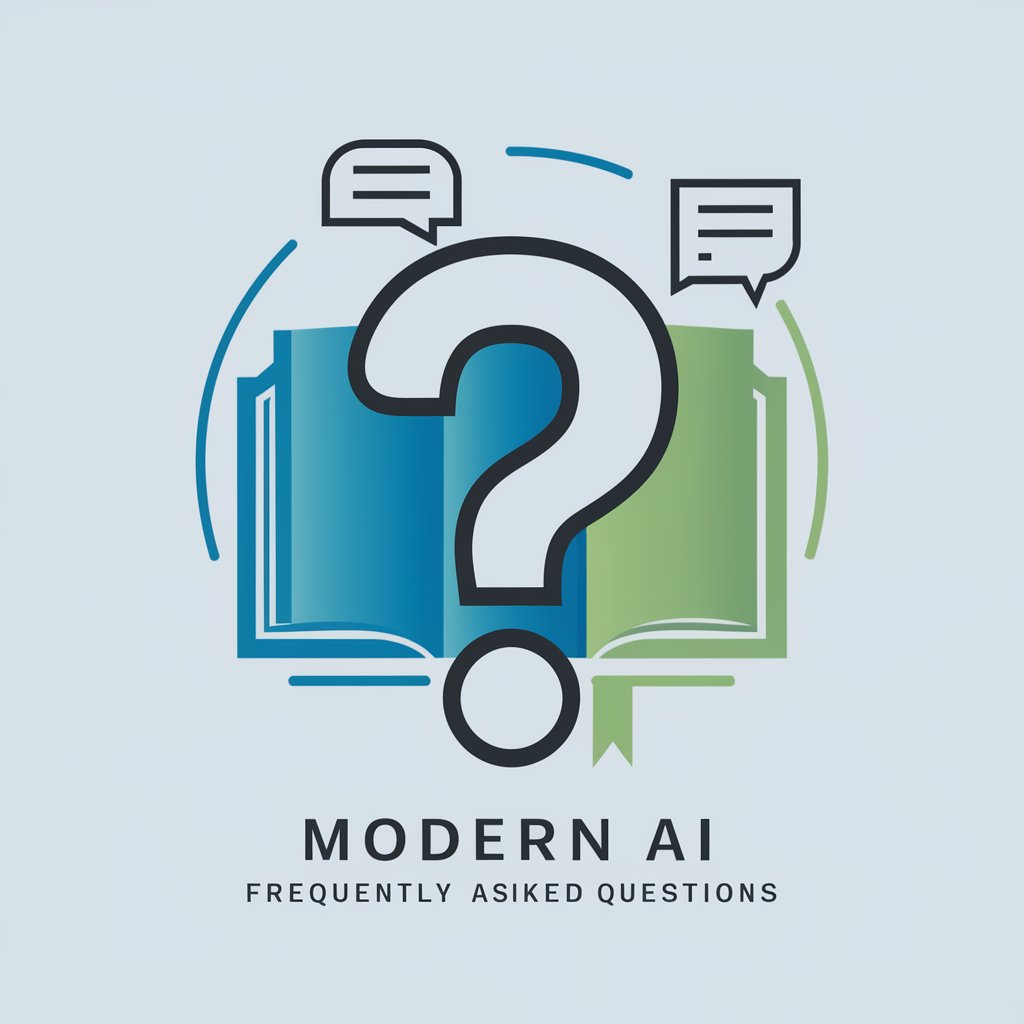
Generative & conversational Ai Optimization FAQs
Empower Your Digital Presence with AI
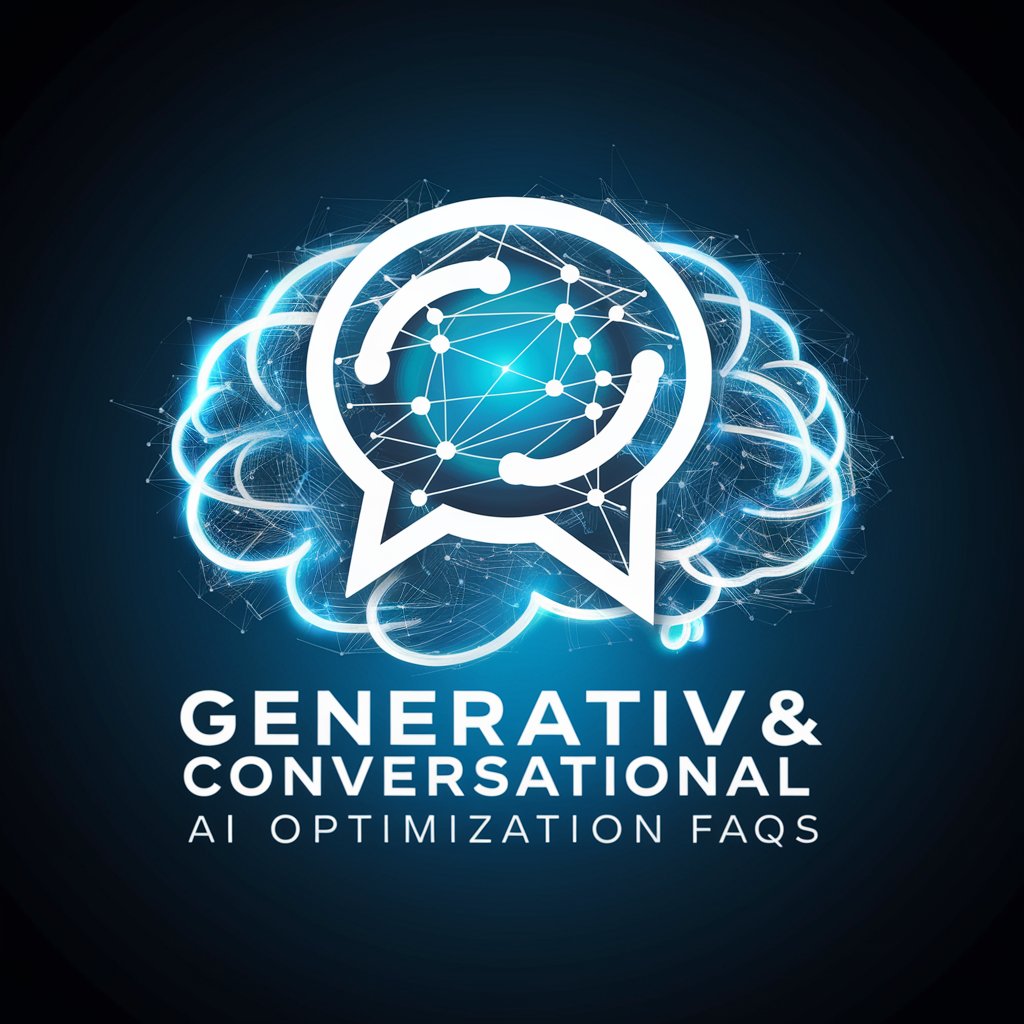
#1 SEO-Powered Article with FAQs from SEO-Booster
Elevate Your SEO Game with AI
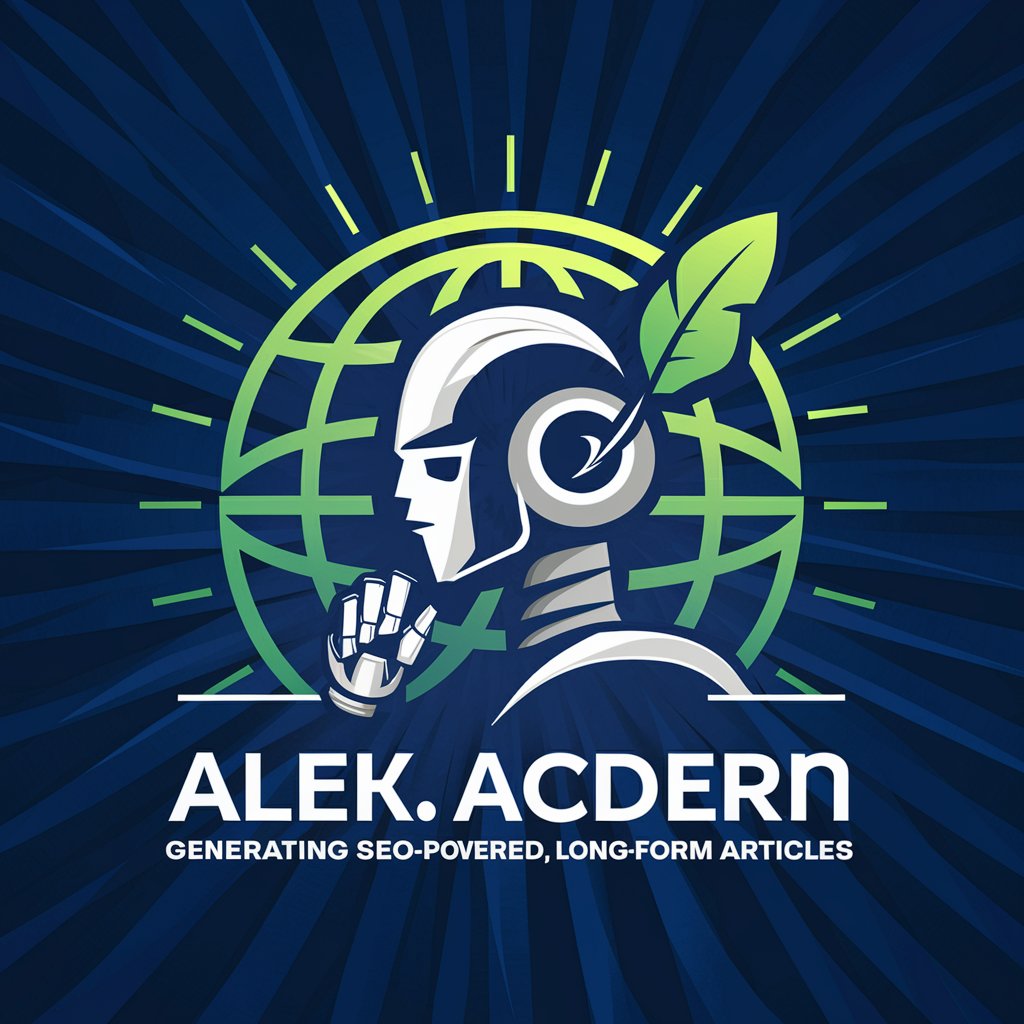
GPT Q&A, FAQs, Quiz Master for educational purpose
Empower Learning with AI-Driven Insights
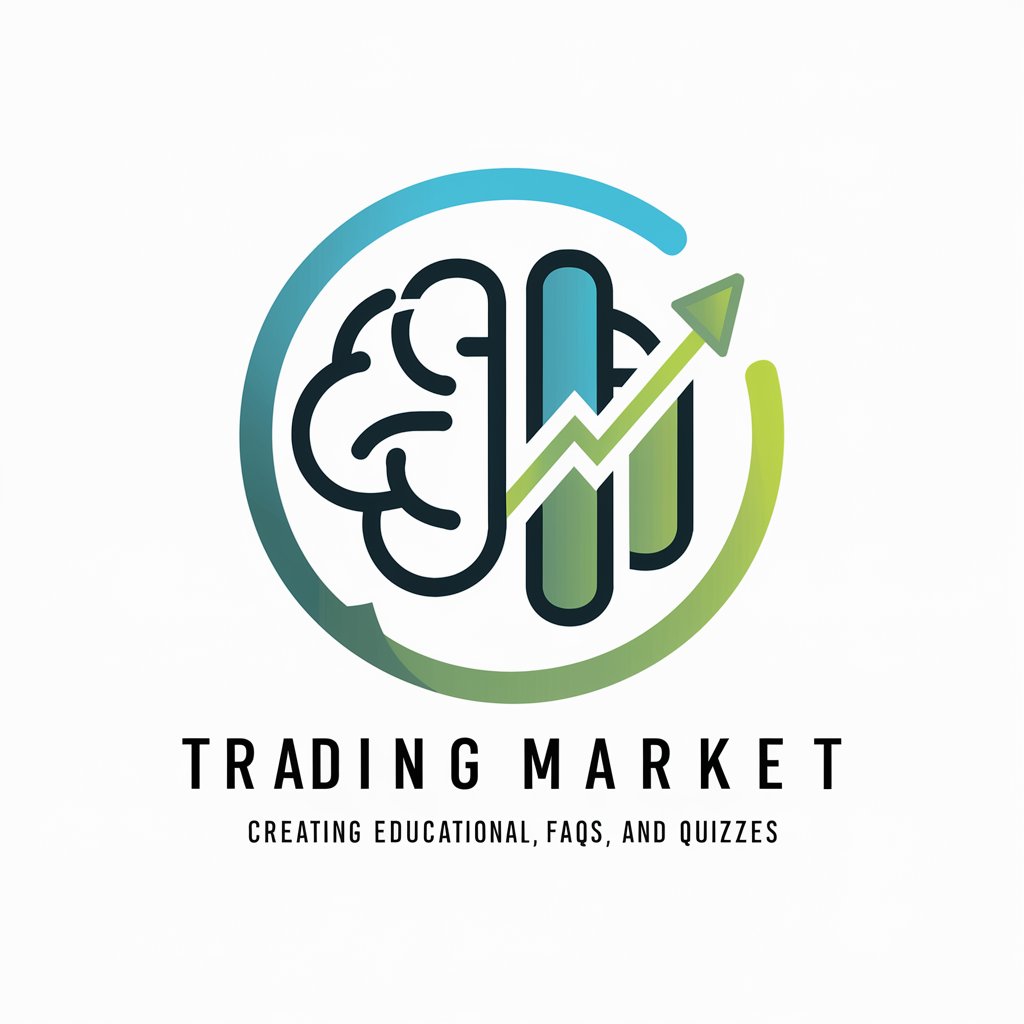
Friendly Mentor
Empathetic AI for Life and Career Guidance
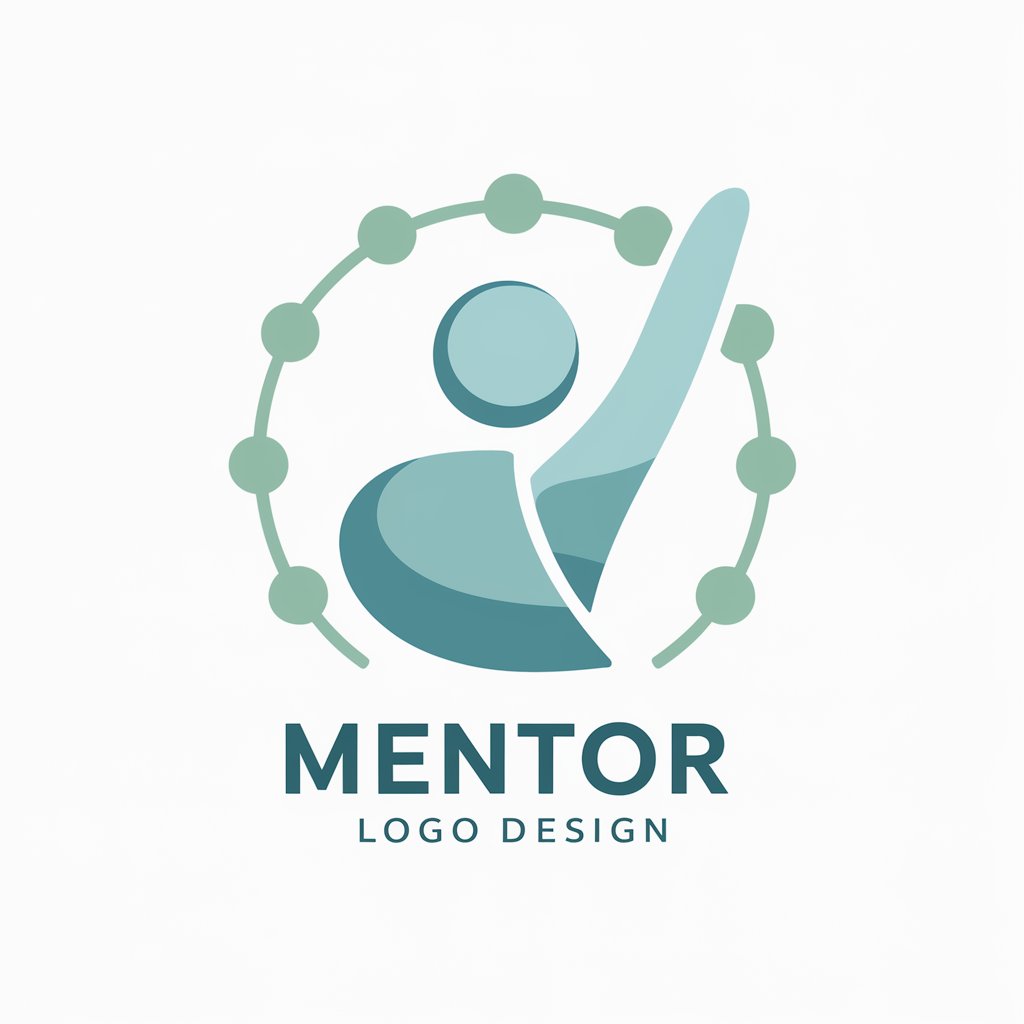
Friendly Educator
Empowering Young Minds with AI
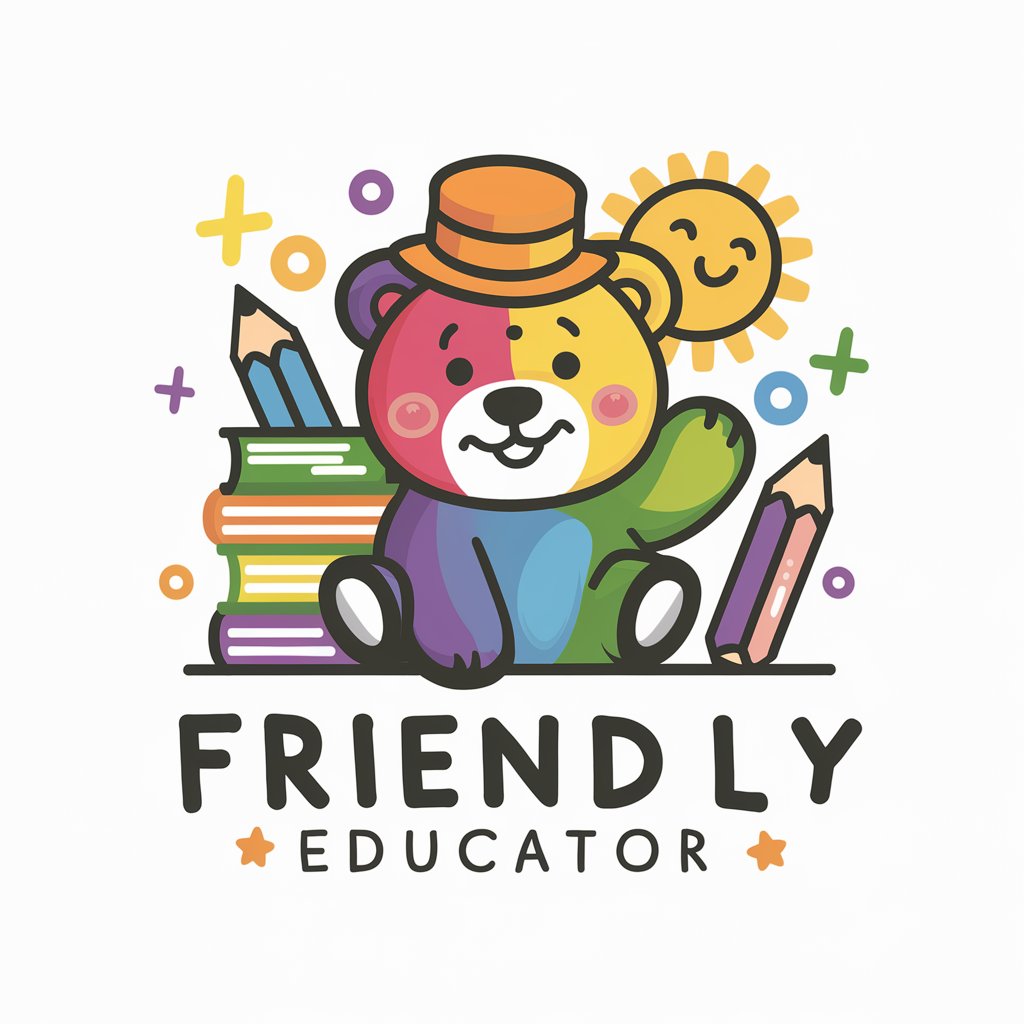
Friendly Writer
Polish Your Words with AI
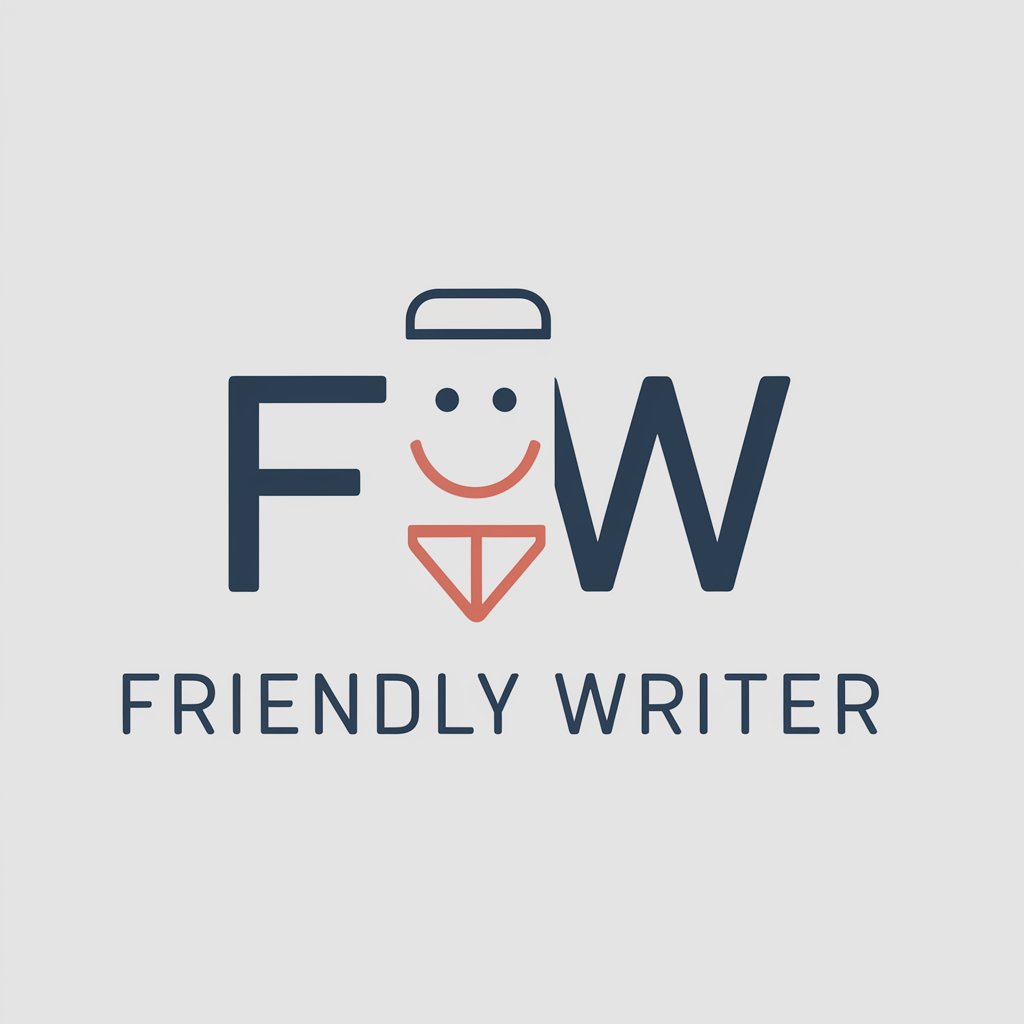
查找GPTs
Discover Your Ideal AI Partner
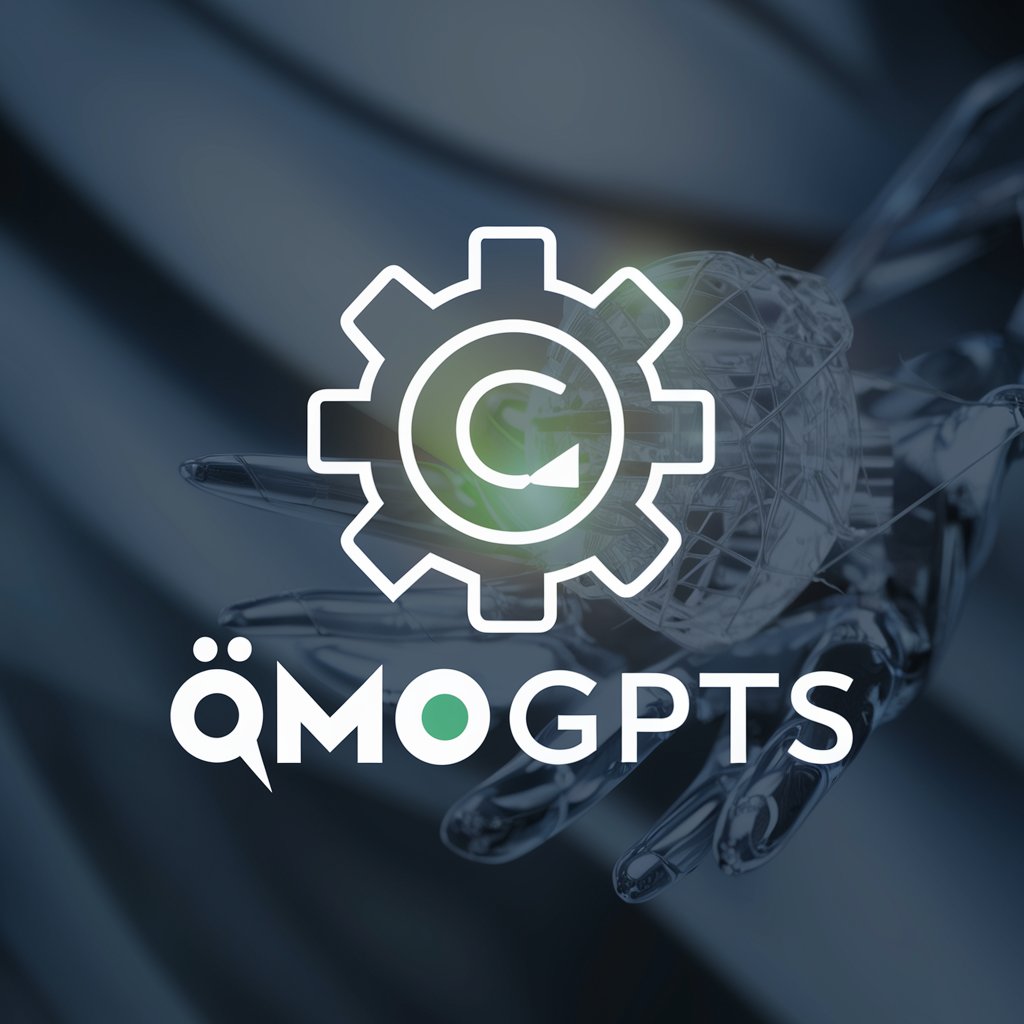
GPTs by CMP: Create Your Best Prompt
Crafting Superior Prompts with AI
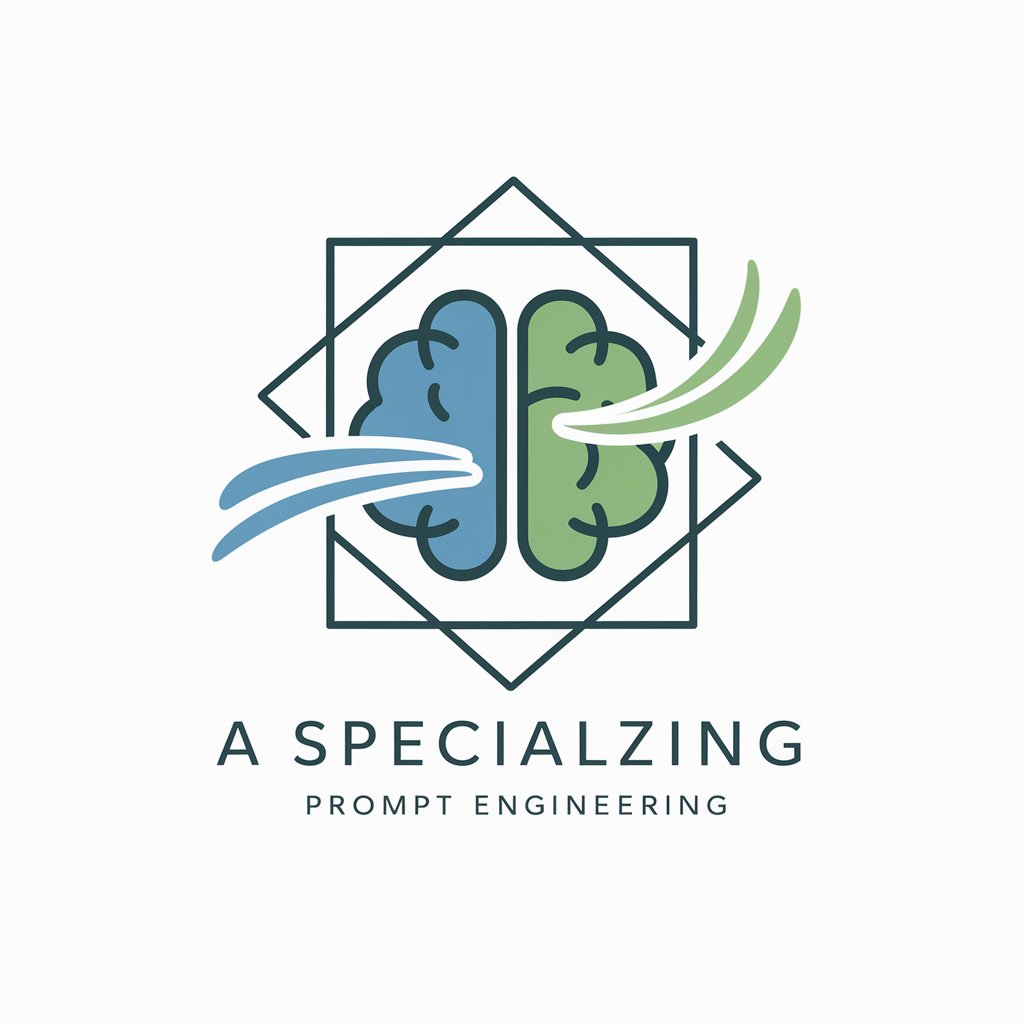
Action Thriller Immersion
Craft Your Thriller, Guide Your Hero
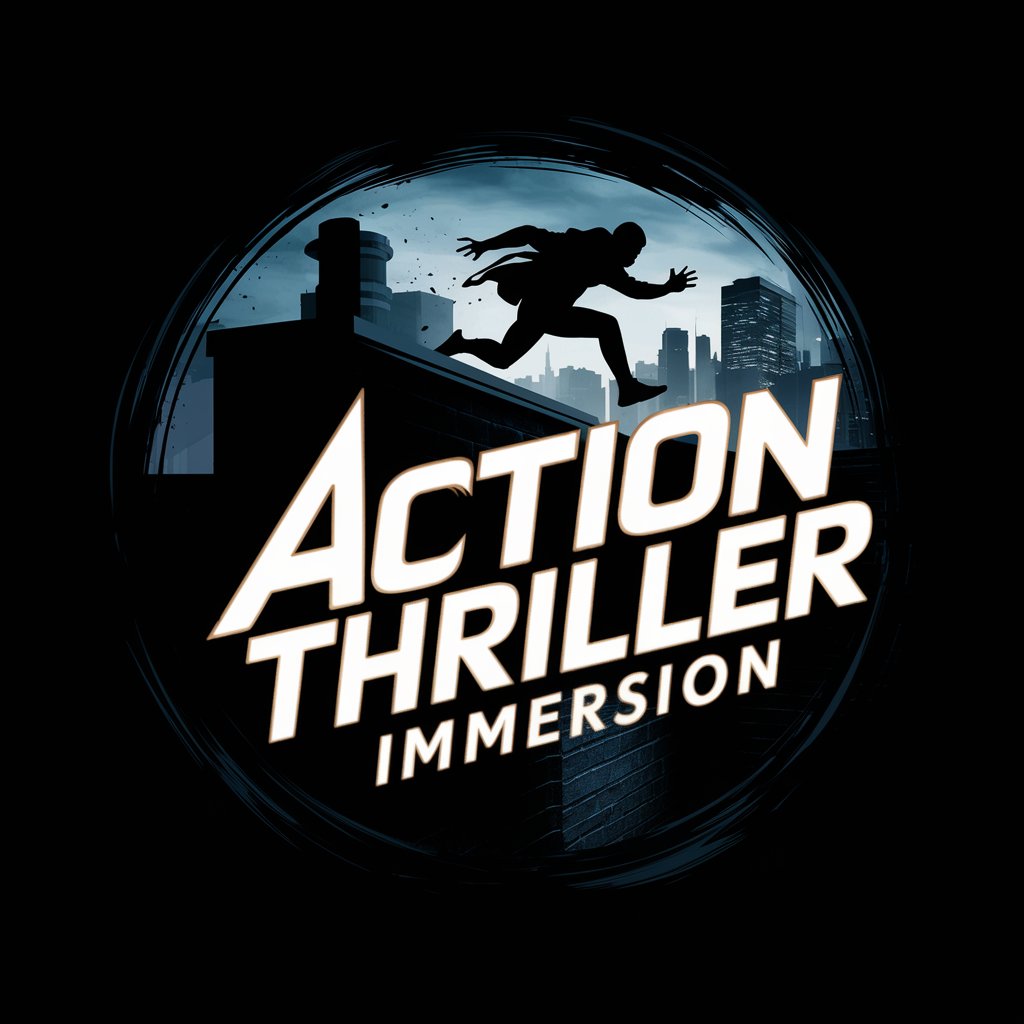
FAQs About Friendly Code
What is Friendly Code?
Friendly Code is a specialized tool designed to assist with econometric and spatial econometric analysis, offering robust features for data exploration, regression analysis, and spatial data interpretation.
How can Friendly Code assist with spatial econometrics?
It provides tools and guidance for identifying spatial autocorrelation, managing geo-referenced data, and employing spatial regression methods, all crucial for understanding spatial patterns in datasets.
Can Friendly Code help with non-spatial econometric models?
Yes, apart from its expertise in spatial econometrics, it also supports various non-spatial econometric analyses, including linear and nonlinear regression models, allowing for a wide range of econometric investigations.
What software or packages does Friendly Code recommend for econometric analysis?
Friendly Code guides users in employing R and Python for econometric analysis, specifically highlighting packages like sp, sf, and spdep for R, and libraries like geopandas and pysal for Python.
Is Friendly Code suitable for beginners in econometrics and spatial analysis?
Yes, it's designed to cater to users at all skill levels, offering step-by-step guidance and clear explanations to make complex econometric and spatial analytical concepts accessible to beginners.