Chain Of Though Prompt - Chain of Thought Prompt Enhancement

Welcome! Let's explore your query with clarity and friendliness. How can I assist?
Elevating reasoning with AI-powered thought chains.
Reformat this math problem:
How would I approach this programming task?
Explain this scientific concept using steps:
Structure this business strategy:
Get Embed Code
Introduction to Chain Of Thought Prompt
The Chain Of Thought Prompt technique significantly enhances the complex reasoning capabilities of large language models (LMs). By generating a series of intermediate reasoning steps, this method encourages models to tackle complex tasks more effectively. For instance, in solving arithmetic problems or executing multi-step reasoning, the prompt guides the model to sequentially break down the problem, reason through each part, and integrate these insights to arrive at a conclusion. An example is solving math word problems, where the model first identifies the quantities involved, calculates intermediate results, and combines these results to find the final answer. This approach not only boosts performance on various reasoning tasks but also provides a transparent window into the model's thought process, making it easier to understand how conclusions are reached. Powered by ChatGPT-4o。
Main Functions of Chain Of Thought Prompt
Enhanced Complex Reasoning
Example
Solving arithmetic commonsense and symbolic reasoning tasks with high accuracy
Scenario
When presented with math word problems, the Chain Of Thought Prompt method allows LMs to generate step-by-step solutions that lead to the correct answer, surpassing the performance of even fine-tuned models without this prompting strategy.
Transparency and Interpretability
Example
Providing understandable reasoning paths for predictions
Scenario
For tasks requiring logical deductions, such as determining if a statement is plausible based on commonsense knowledge, this method offers an interpretable chain of reasoning that elucidates how the model arrived at its conclusion.
Improved Performance across Different Tasks
Example
Achieving state-of-the-art results on benchmarks like GSM8K
Scenario
By applying the Chain Of Thought Prompt, LMs can achieve new performance highs on various benchmarks, demonstrating its versatility and effectiveness across a broad spectrum of reasoning and comprehension tasks.
Ideal Users of Chain Of Thought Prompt Services
Researchers and Developers in AI and NLP
This group benefits significantly from the Chain Of Thought Prompt's ability to enhance LMs for complex reasoning tasks, facilitating the development of more capable and interpretable AI systems.
Educational Technologists
Educators and technologists can leverage this technique to create intelligent tutoring systems that provide step-by-step problem-solving guidance, making learning more interactive and understandable for students.
Data Scientists and Analysts
Professionals in data science and analysis can utilize Chain Of Thought Prompt-enhanced models for data exploration, hypothesis testing, and complex problem-solving, benefiting from the method's structured reasoning capabilities.
How to Use Chain Of Thought Prompt
Starting Point
Begin by visiting yeschat.ai for a complimentary trial, accessible without registration or ChatGPT Plus subscription.
Understanding Chain of Thought
Familiarize yourself with the chain of thought concept—intermediate reasoning steps leading to a solution, enhancing problem-solving skills.
Practical Application
Apply chain of thought by breaking down complex problems into smaller, manageable steps, integrating these to arrive at a solution.
Experimentation
Experiment with various types of prompts and observe how chain of thought enhances the reasoning abilities of language models.
Reflect and Adapt
Evaluate the effectiveness of applied strategies, refine your approach based on insights, and adapt your use of chain of thought prompts for broader applications.
Try other advanced and practical GPTs
Your automobile mechanic
AI-powered car care companion

Edward Doty History
Discover Your Pilgrim Roots with AI

Assistance & Compensation for EU Flight Passengers
Navigate flight disruptions with AI-powered assistance.

Ship's Computer
Navigating the Future with AI Assistance

Air Passenger refund Assistant
Empowering passengers with AI-driven rights knowledge.

Choice Under Uncertainty Tutor
Navigating Uncertainty with AI

To you who I miss even though I can't draw anymore
Empathy through art, language, and shared memories.

Student (Neuro)
Empowering neuroscience learning with AI

Student buddy
Empowering students with AI-driven learning support

Bible Student
Unlocking the Bible with AI

WTB-student
Elevate Your Engineering Projects with AI
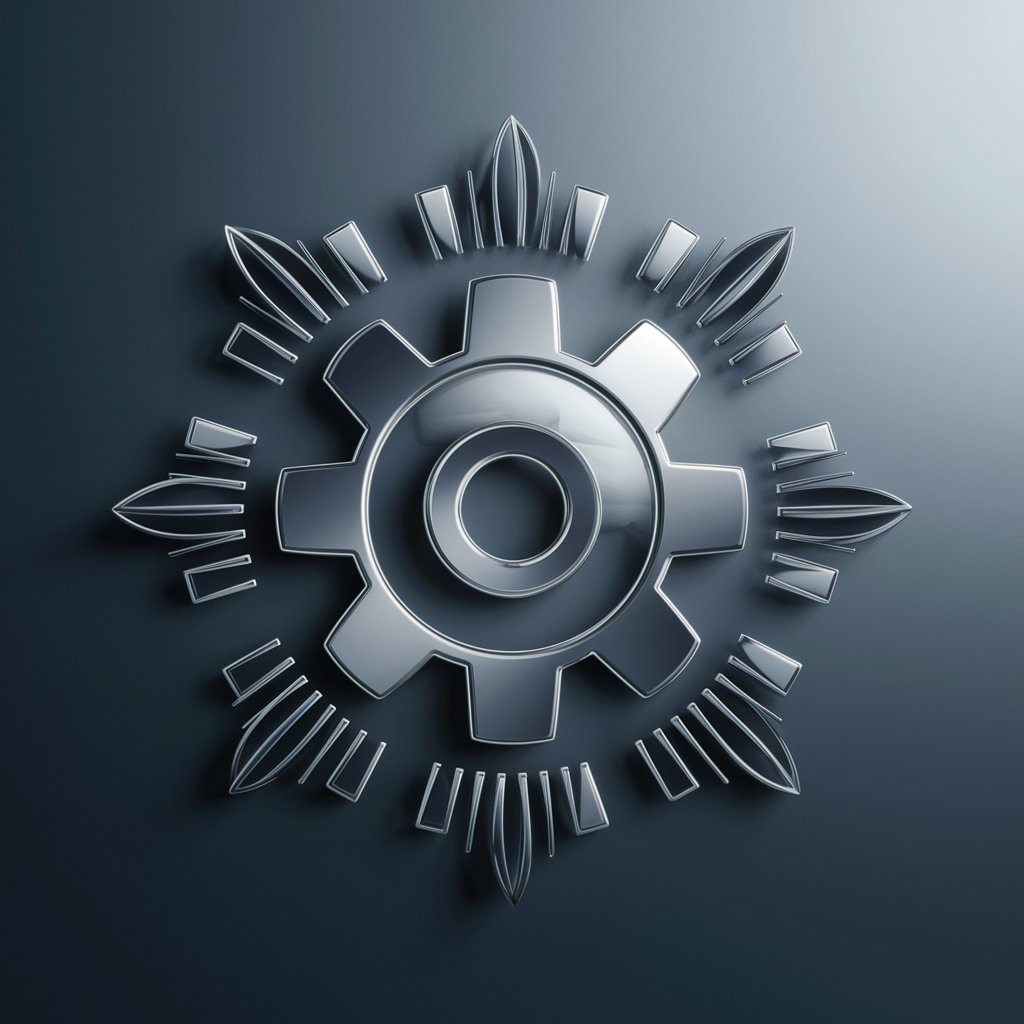
Medical Student Tutor
Empowering Medical Learning with AI
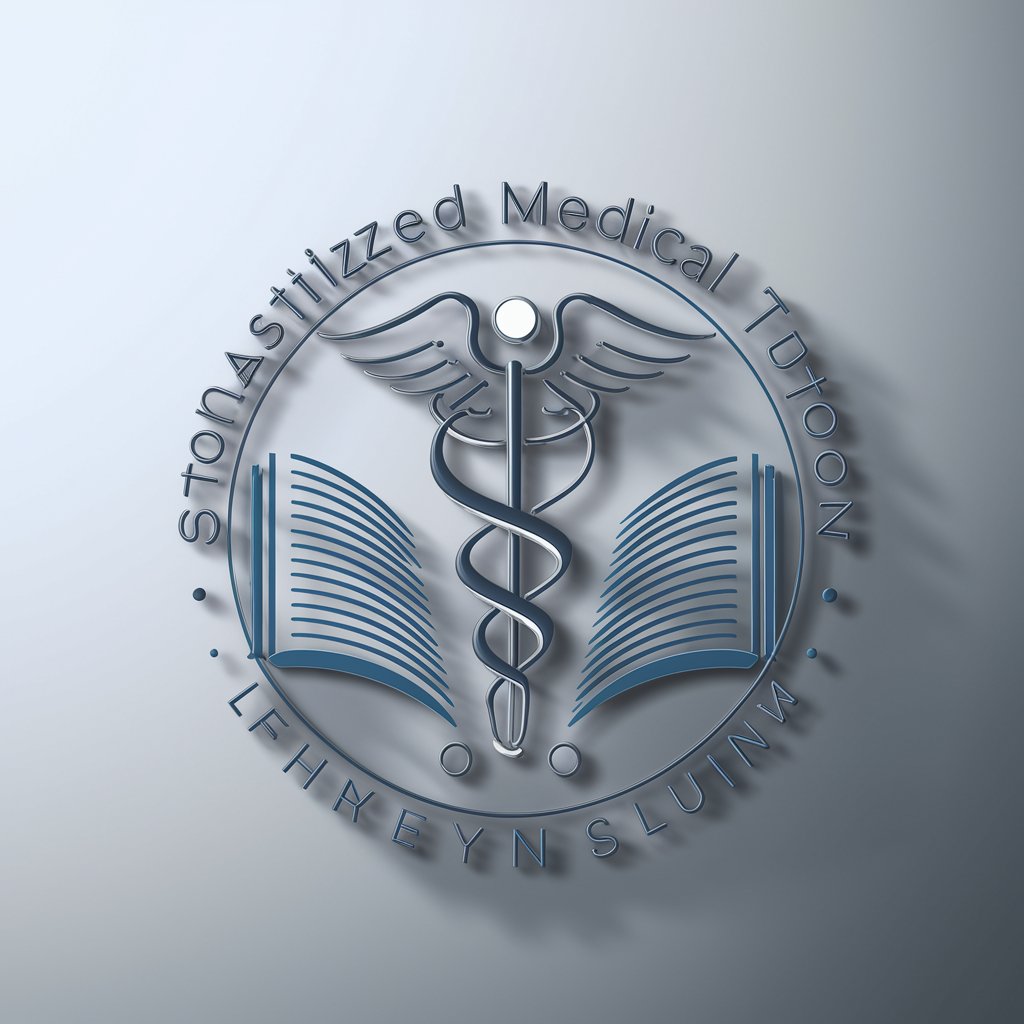
Chain Of Thought Prompt Q&A
What is Chain Of Thought Prompt?
It's a prompting technique for language models, eliciting a series of intermediate reasoning steps to improve complex problem-solving abilities.
How does Chain Of Thought Prompt improve model performance?
By breaking down tasks into intermediate steps, it enhances models' understanding and reasoning, leading to more accurate solutions.
Can Chain Of Thought Prompt be applied to any language model?
Yes, it's versatile and can be applied to various models, significantly enhancing their reasoning capabilities without specific tuning.
What types of problems benefit most from Chain Of Thought Prompt?
Problems involving complex reasoning, such as arithmetic, commonsense, and symbolic reasoning tasks, see notable performance improvements.
How can I effectively create Chain Of Thought Prompts?
Identify key components of the problem, break it down into logical steps, ensure clarity in each step, and integrate these to form a coherent solution path.