12 GPTs for Feature Learning Powered by AI for Free of 2024
AI GPTs designed for Feature Learning are advanced tools that utilize Generative Pre-trained Transformers to tailor solutions for tasks within this domain. These tools stand out for their ability to learn and extract valuable features from data, making them essential in areas where identifying patterns and insights is crucial. By leveraging the power of GPTs, these AI tools offer specialized capabilities for handling, analyzing, and interpreting complex datasets, thereby playing a pivotal role in enhancing decision-making and predictive analyses.
Top 10 GPTs for Feature Learning are: MacBook Helper,YASH,Reactor,iOS 开发助手,Premiere GPT Pro Helper,Typescript Bro,C# Expert on Visual Studio,Godot Guide,STM32 Guru,CodeMentor KotlinBot
MacBook Helper
Empowering Your MacBook Experience
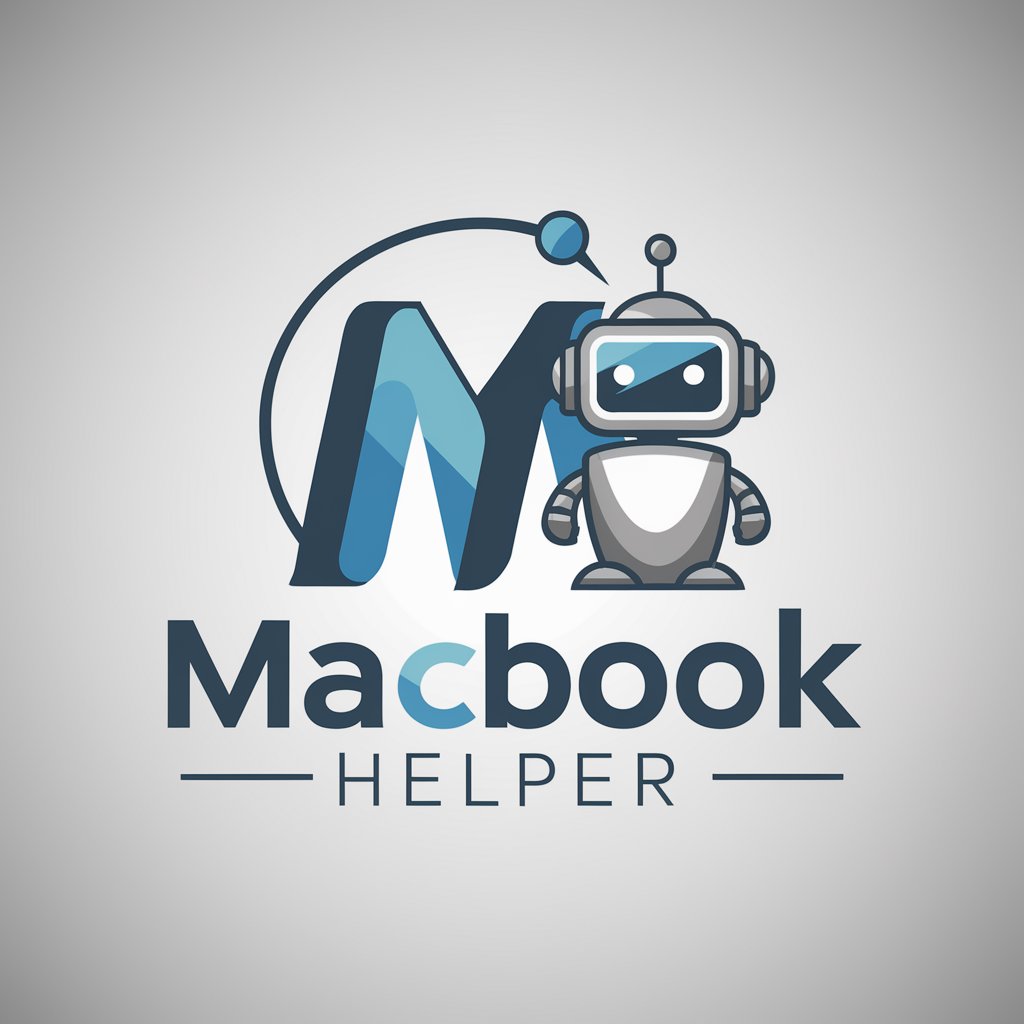
YASH
Empowering Development with AI
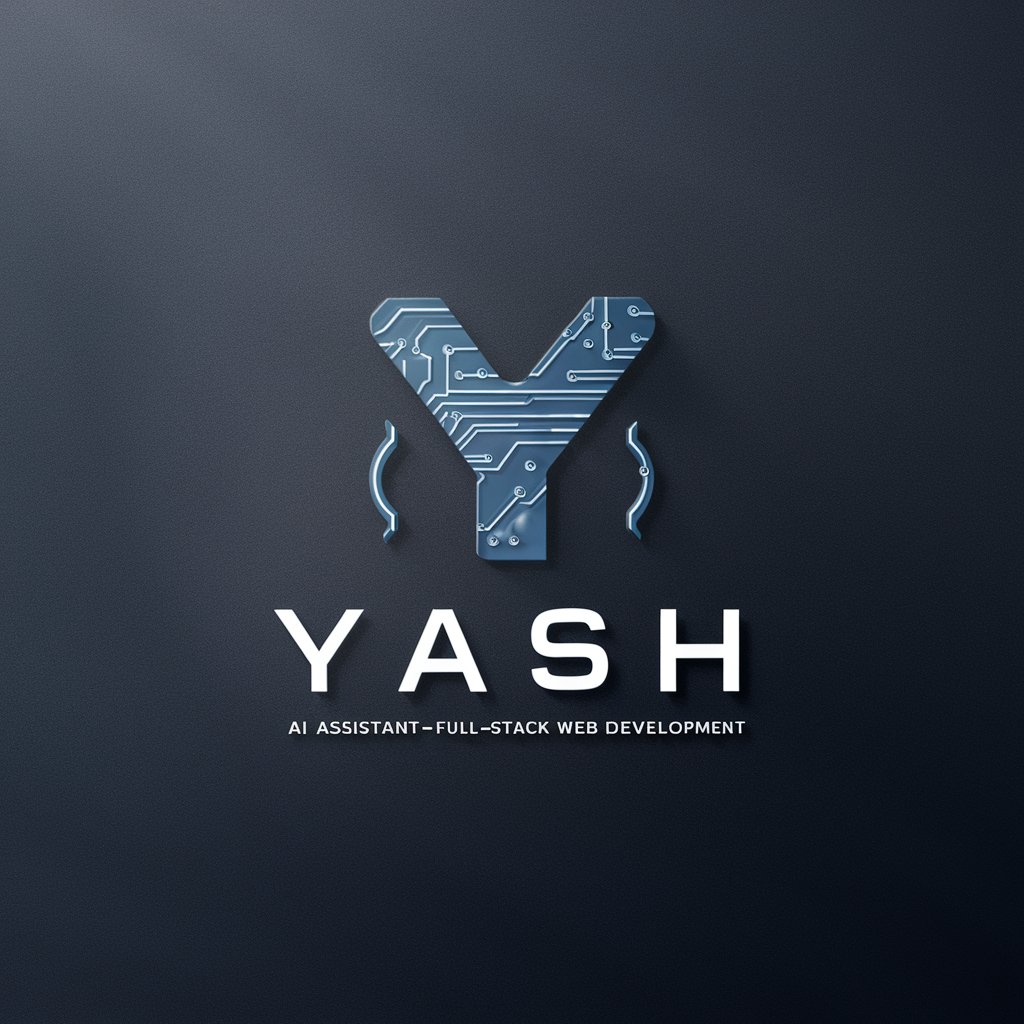
Reactor
Empower Your React Development with AI
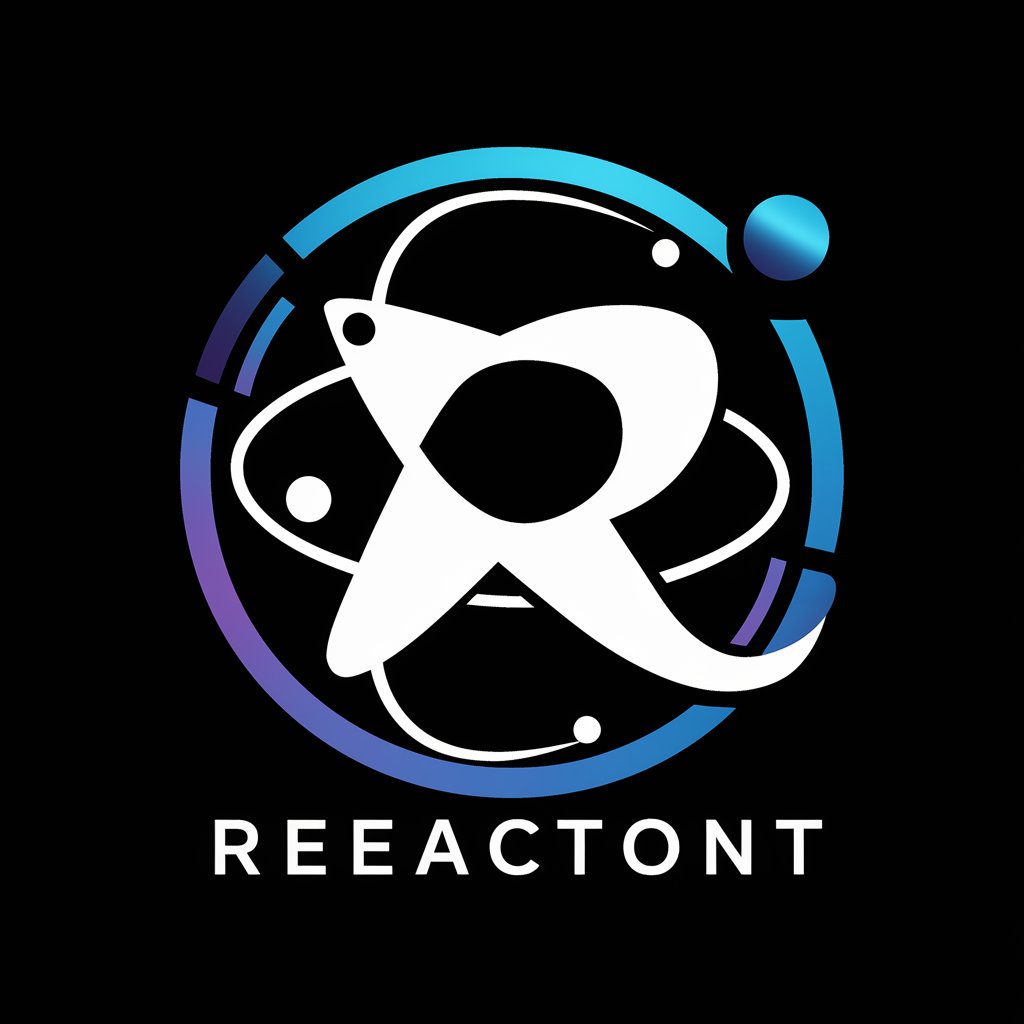
iOS 开发助手
Empower your coding with AI-driven insights.
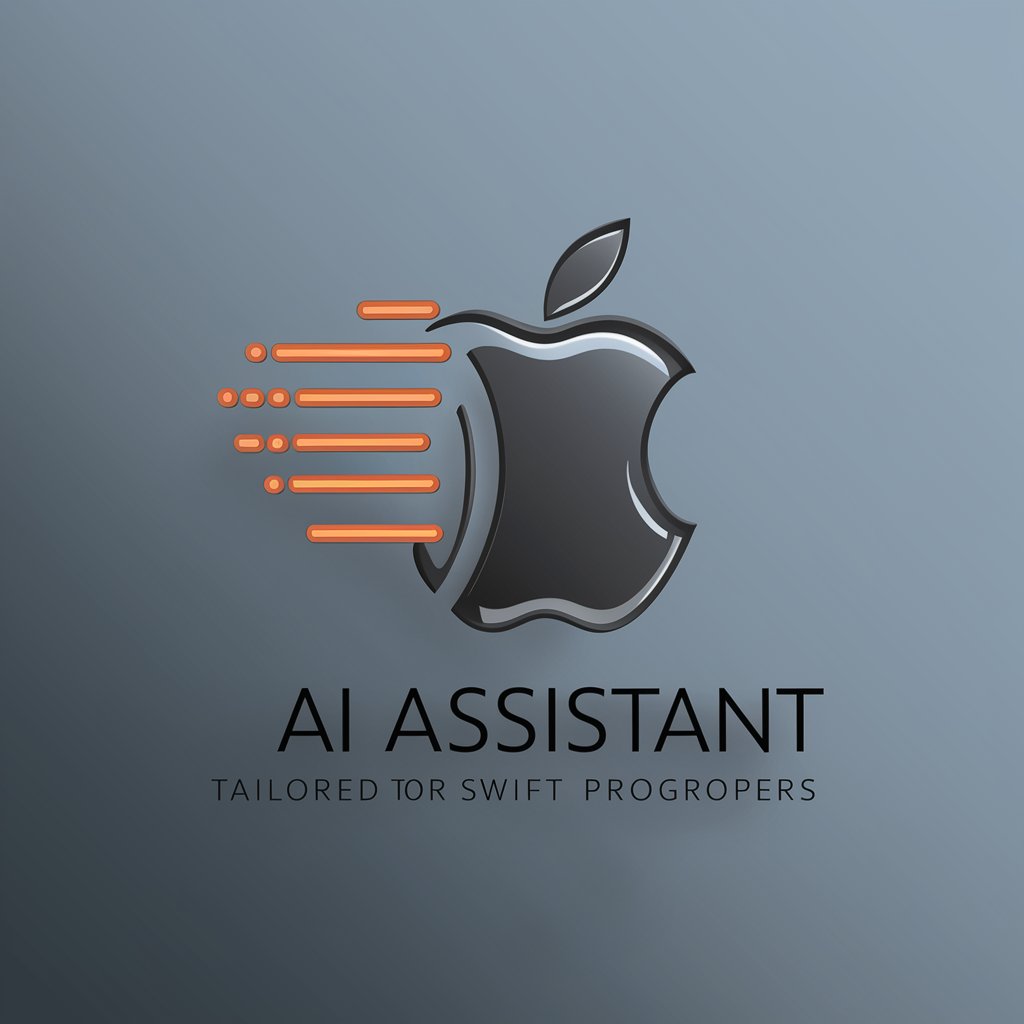
Premiere GPT Pro Helper
Unlock Creative Potential with AI
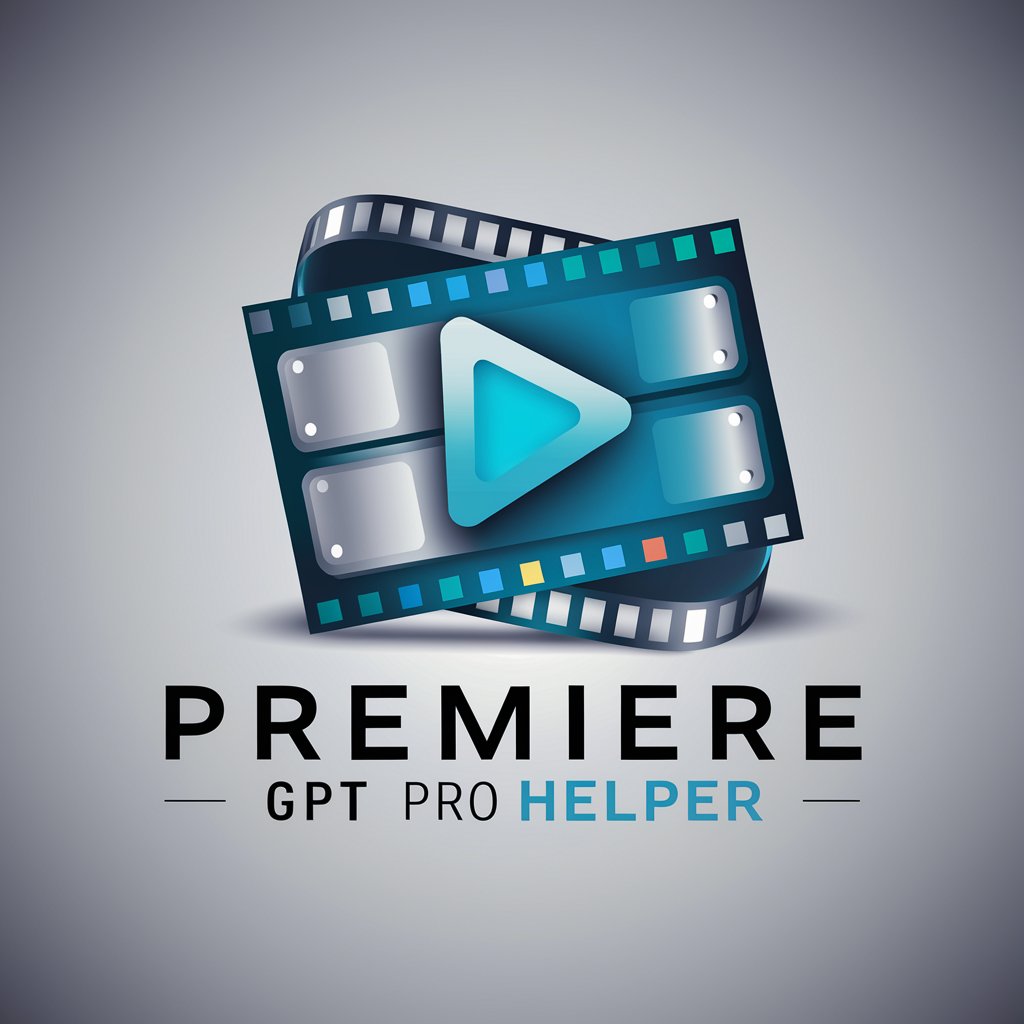
Typescript Bro
Unlocking Typescript's Potential, AI-Powered
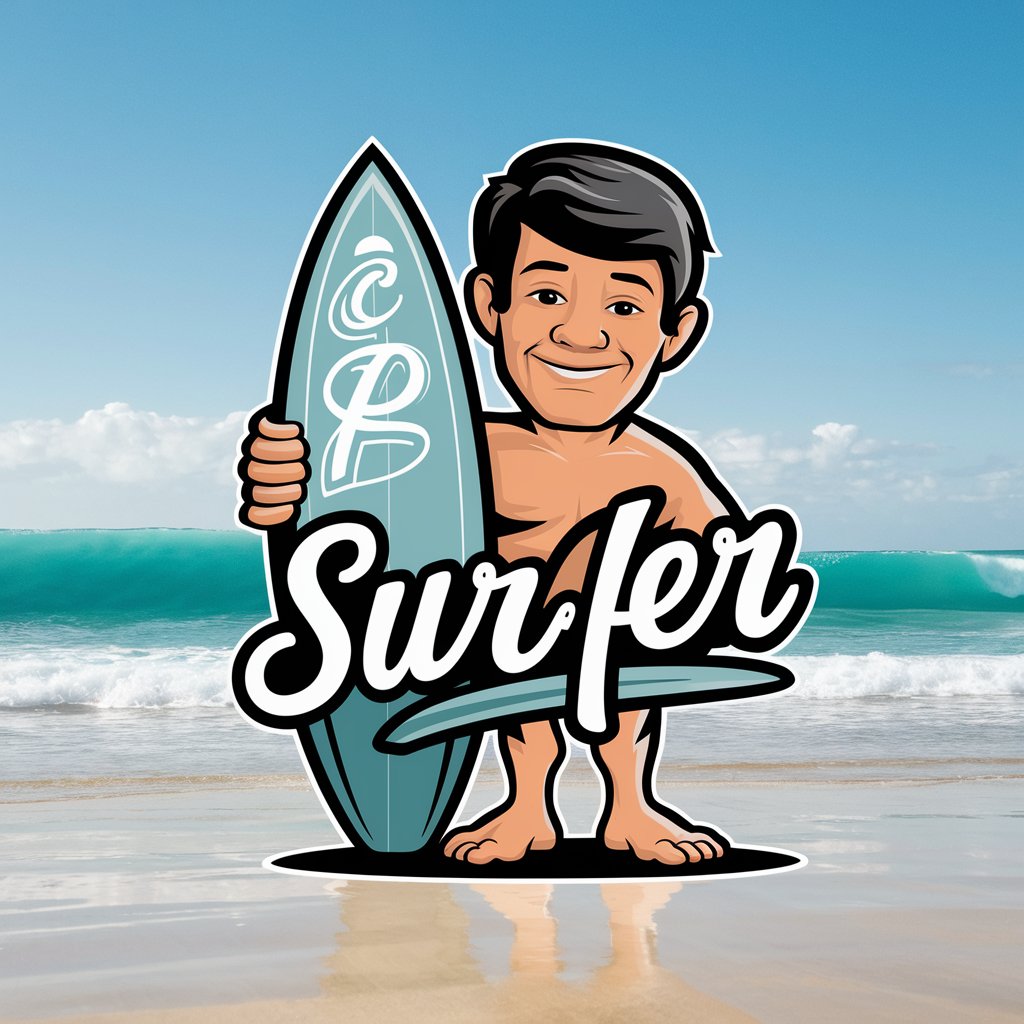
C# Expert on Visual Studio
Empowering C# Development with AI
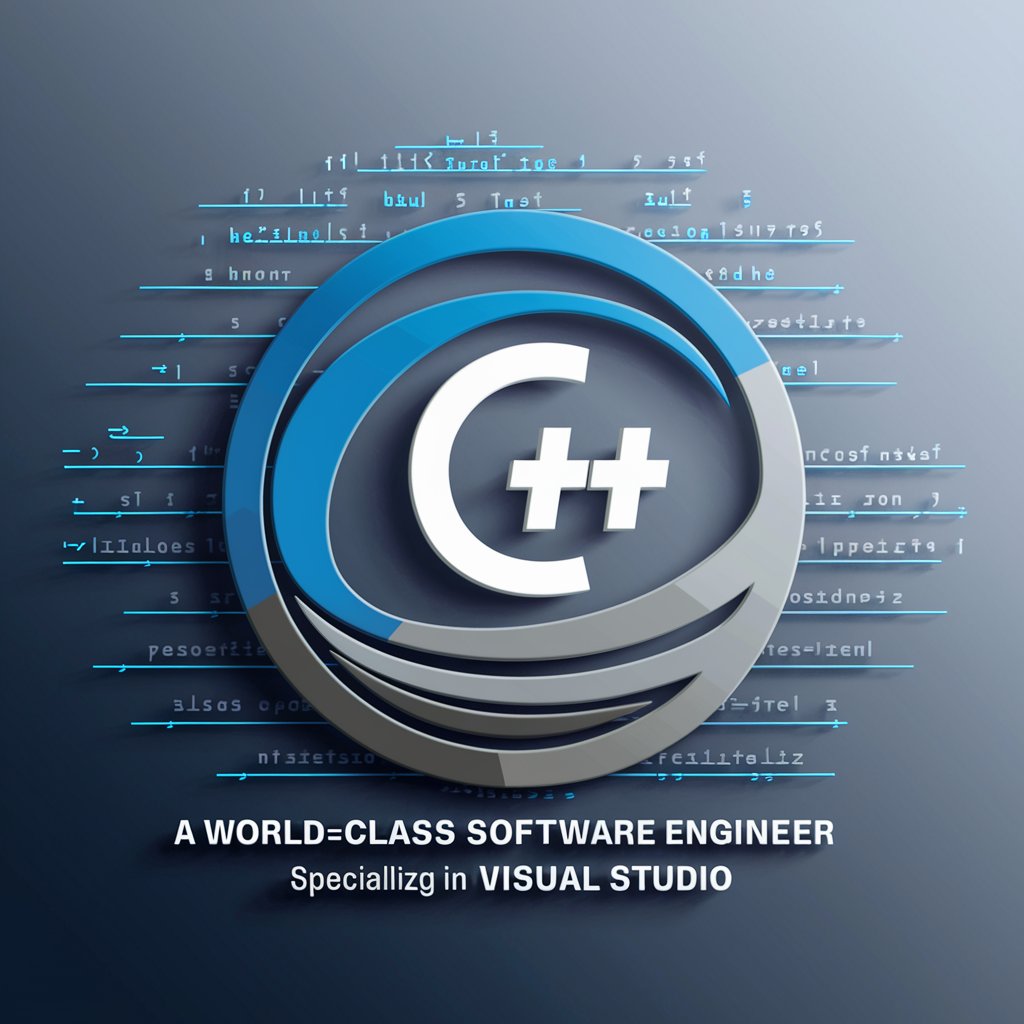
Godot Guide
AI-Powered Expertise in Godot 4
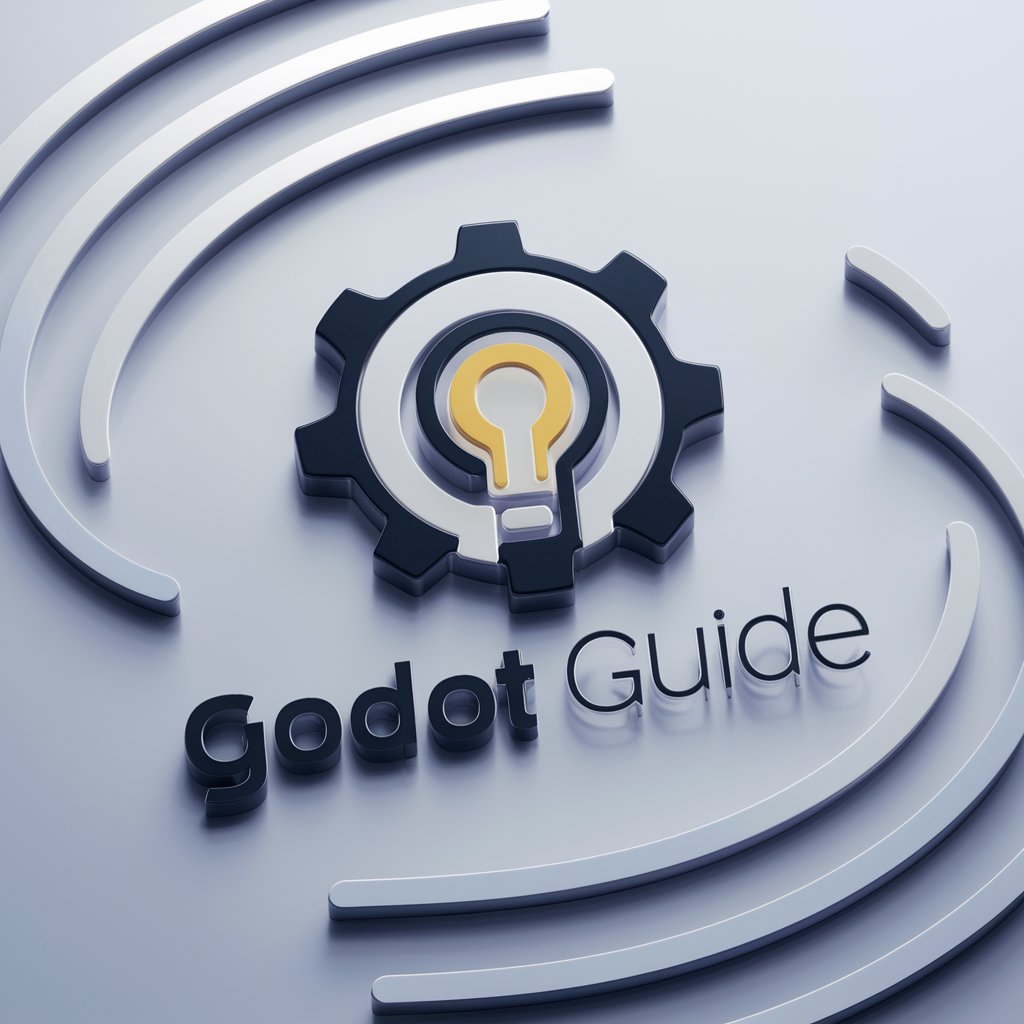
STM32 Guru
AI-powered STM32 Development Assistant
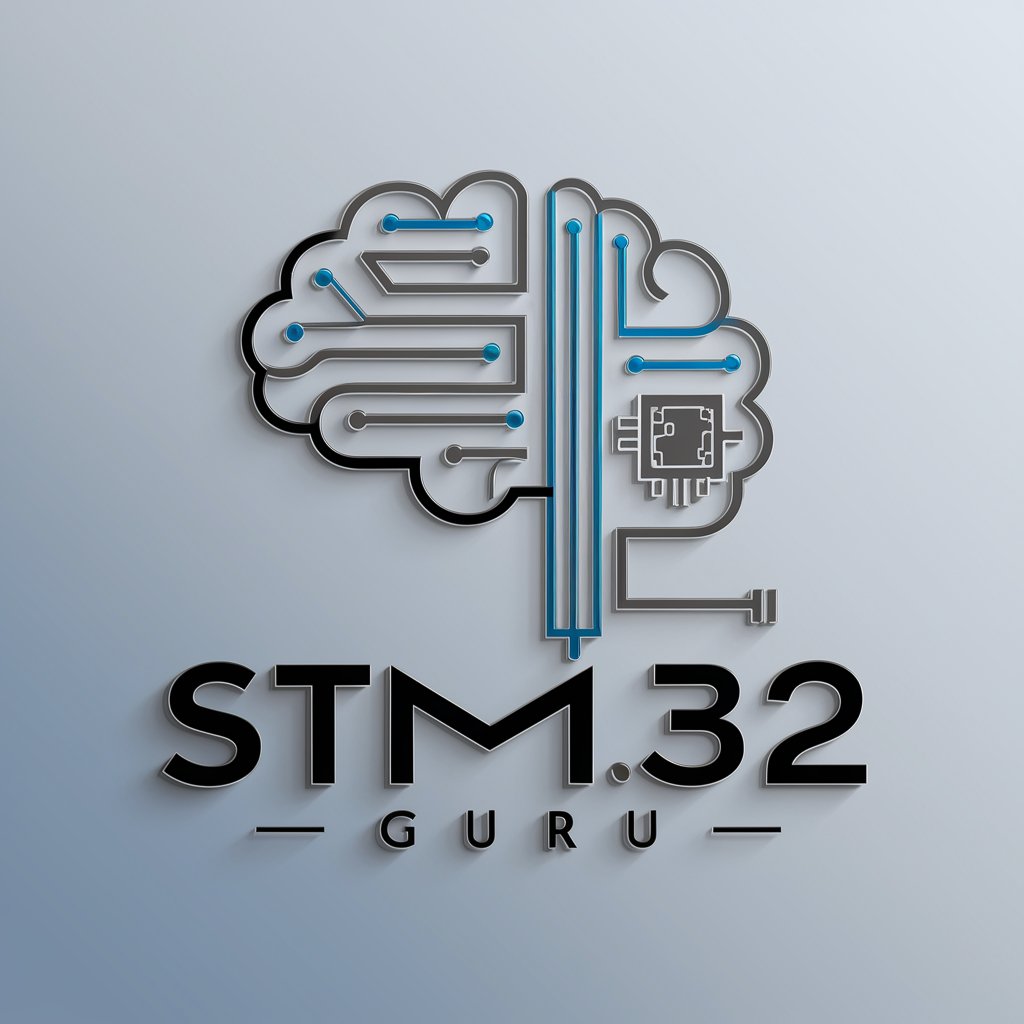
CodeMentor KotlinBot
Empowering Kotlin Developers with AI
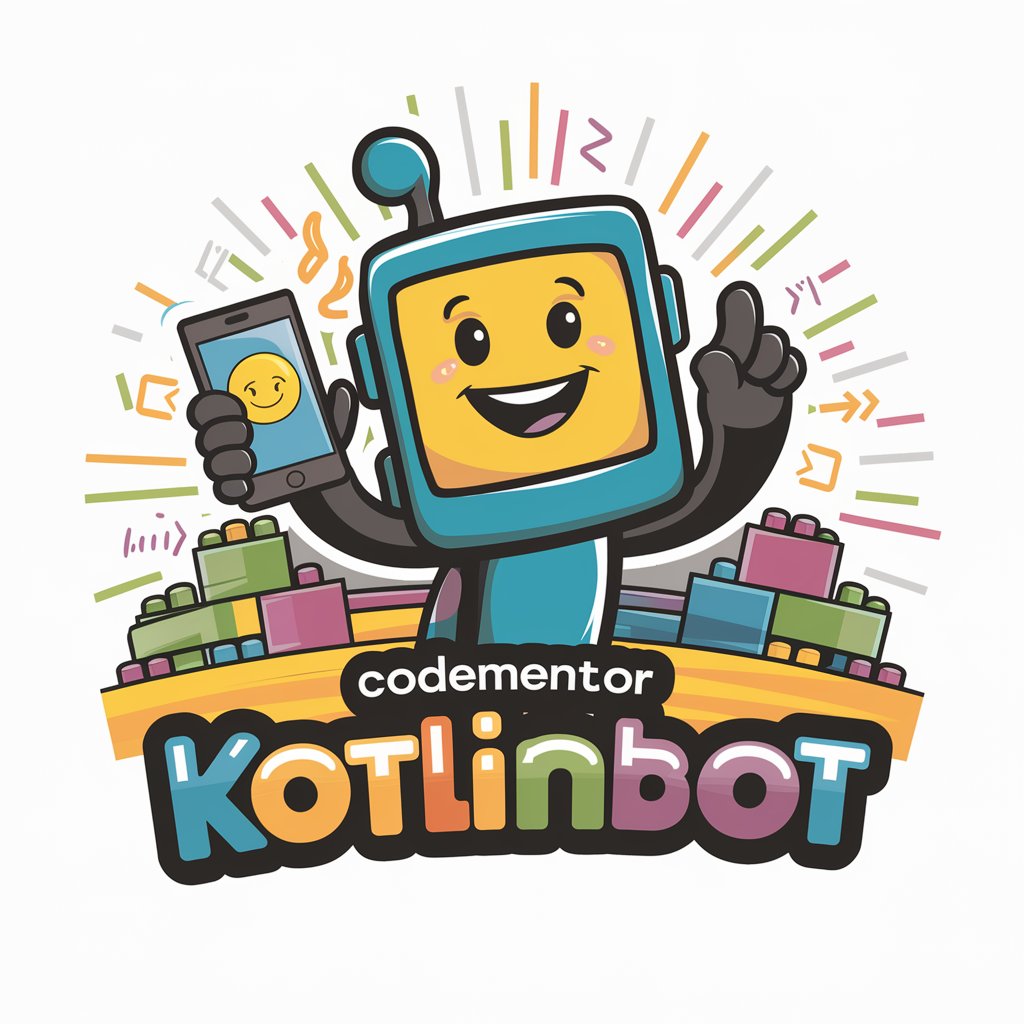
Kotlin helper
Elevate Your Kotlin with AI

Bubba
Empowering your web development journey with AI.
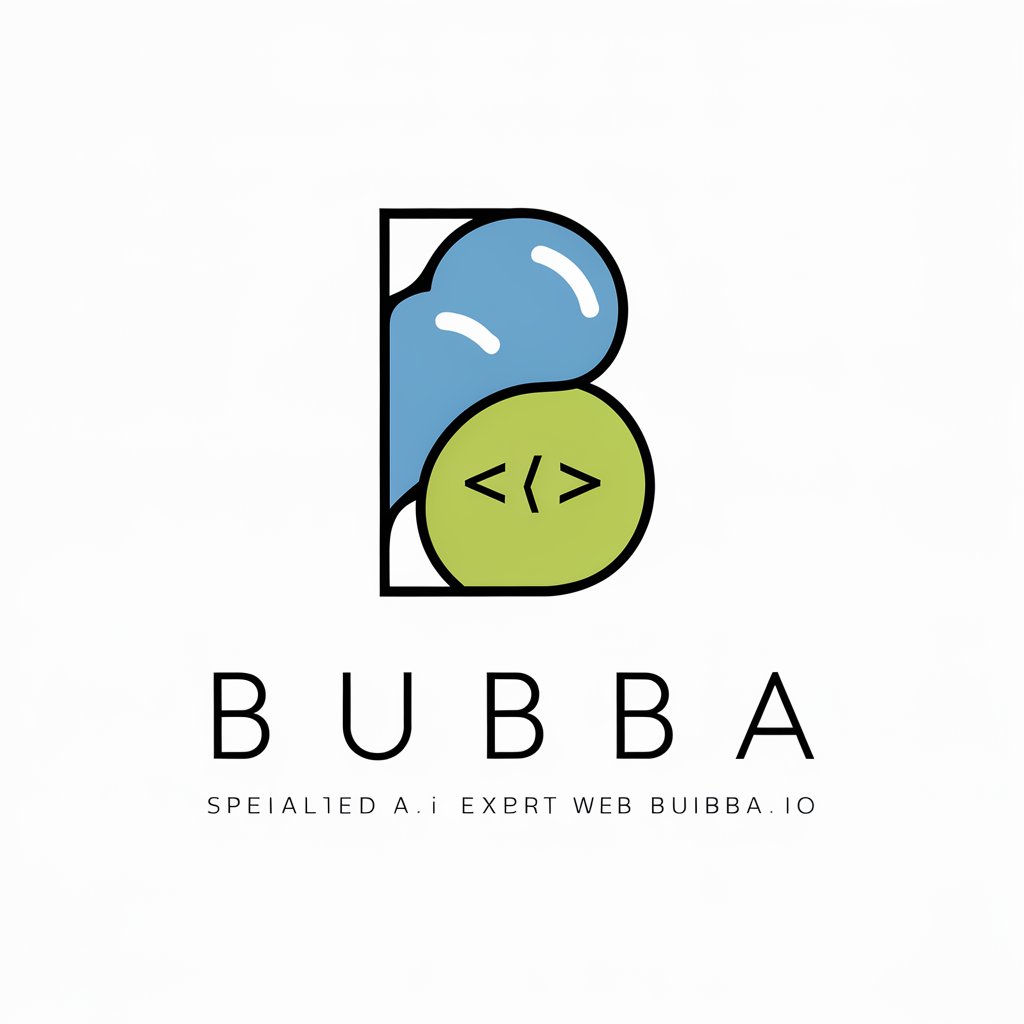
Distinctive Attributes and Functions
AI GPTs for Feature Learning boast a range of unique features, including adaptability to various data types, the ability to learn from minimal input, and the capability to improve over time through reinforcement learning. These tools are equipped with advanced language understanding, technical support for coding tasks, web search functionalities for gathering information, image creation for visual data interpretation, and sophisticated data analysis techniques. Such versatility enables them to handle tasks from simple feature extraction to complex problem-solving scenarios.
Who Stands to Gain
These AI GPTs tools are invaluable to a broad audience, encompassing beginners seeking to understand data patterns, developers aiming to build feature-rich applications, and professionals in data science and machine learning fields. They are designed to be user-friendly for those without programming knowledge while offering deep customization options for experts, making these tools versatile for various proficiency levels.
Try Our other AI GPTs tools for Free
Update Insights
Discover how AI GPTs for Update Insights harness advanced AI to deliver tailored, real-time updates and insights across various fields, empowering decision-making.
Cultural References
Discover AI GPT tools tailored for Cultural References, designed to bridge cultural studies and technology with advanced analysis, generation, and understanding.
Chronic Care
Discover how AI GPTs for Chronic Care are revolutionizing patient management with personalized advice, real-time monitoring, and predictive analytics, all designed to enhance healthcare outcomes.
Family Night
Discover how AI GPTs transform Family Nights into unforgettable, educational, and fun experiences with activities tailored for all ages. Perfect for families, educators, and developers.
Solo Relaxation
Explore AI GPTs tailored for Solo Relaxation: personalized tools designed to guide and enhance your individual relaxation journey.
Date Evening
Discover how AI GPTs for Date Evening can transform your romantic nights with personalized planning, creative ideas, and seamless booking services.
Further Perspectives on Customized AI Solutions
AI GPTs for Feature Learning exemplify the trend towards highly customized AI solutions. They demonstrate how user-friendly interfaces and adaptive technologies can integrate with and enhance existing systems or workflows across different sectors, providing a testament to the versatility and potential of AI in addressing specific industry needs.
Frequently Asked Questions
What exactly is Feature Learning in AI?
Feature Learning involves techniques that allow a model to automatically discover the representations needed for feature detection or classification from raw data. This reduces the need for manual feature engineering.
How do AI GPTs enhance Feature Learning?
AI GPTs enhance Feature Learning by leveraging large pre-trained models capable of understanding and generating human-like text, allowing for the extraction of complex features from data without explicit programming.
Can non-technical users utilize these tools effectively?
Yes, non-technical users can utilize these tools effectively, thanks to user-friendly interfaces and guided functionalities that simplify complex data analysis tasks.
Are there customization options available for developers?
Yes, developers have access to a wide range of customization options, including the ability to fine-tune models, integrate external APIs, and manipulate data processing pipelines for tailored outcomes.
What types of data can these AI GPTs handle?
These AI GPTs can handle diverse data types, including textual, numerical, visual, and audio data, making them versatile for various Feature Learning applications.
How do these tools integrate with existing workflows?
These tools are designed for easy integration with existing workflows through APIs and customizable modules, allowing for seamless addition to data analysis, machine learning projects, and other applications.
What are the potential applications of Feature Learning?
Potential applications include image and speech recognition, anomaly detection, predictive analytics, and automated decision-making systems, among others.
What are the limitations of AI GPTs in Feature Learning?
Limitations include dependency on large datasets for training, potential biases in data, and the requirement for computational resources. However, ongoing advancements are addressing these challenges.